Computational biomarker predicts lung ICI response via deep learning-driven hierarchical spatial modelling from H&E
semanticscholar(2022)
摘要
Determining which lung cancer patients are likely to respond to immune checkpoint inhibitors (ICI) remains a crucial challenge. Existing FDA-approved biomarkers lack sensitivity and specificity for identifying treatment candidates. To overcome this problem, we present a computational biomarker for predicting ICI response directly from routine H&E stained whole slide images of the initial biopsy. To achieve this, we developed an end-to-end deep learning system (EPL-GNN) that performs hierarchical spatial modeling on whole slide images to learn both spatial and morphological features from 2.1 billion cells and output a response score for each patient. The computational biomarker was trained and evaluated on the largest reported cohort of stage 4 lung cancer patients with ICI treatment response (N=583), resulting in an AUC of 0.69 and sensitivity of 91% on the independent test cohort, which compares favorably to PD-L1 immunohistochemistry (IHC) with an AUC of 0.68 and sensitivity of 57%, and tumor mutation burden (TMB) with an AUC of 0.62. The EPL-GNN model correctly identified 81% of the patients with a negative PD-L1 IHC result as responders. Visualizations of the hierarchical spatial model revealed potential cellular patterns that correspond to ICI treatment response. In addition to the increased sensitivity achieved by the EPL-GNN model, H&E-based Computational Biomarkers offer a faster, less expensive, more objective and reproducible alternative or adjunct to existing IHC or sequencing based biomarkers.
更多查看译文
关键词
computational biomarker,lung,hierarchical spatial modelling,learning-driven
AI 理解论文
溯源树
样例
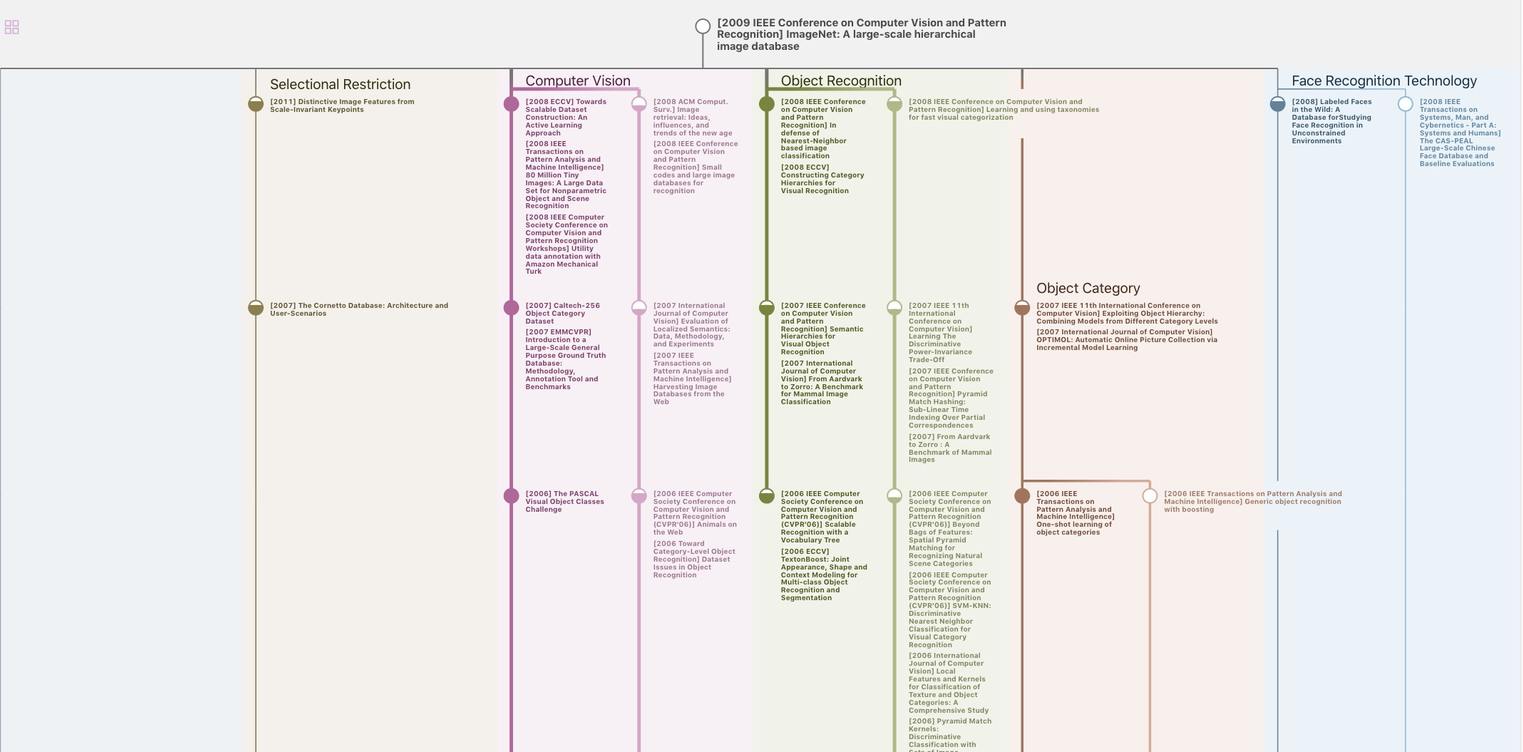
生成溯源树,研究论文发展脉络
Chat Paper
正在生成论文摘要