Class-Wise Adaptive Self Distillation for Heterogeneous Federated Learning
semanticscholar(2022)
摘要
The heterogeneity of data distributions among clients (nonIID) has been identified as one of the key challenges in federated learning. In the local training phase, each client model optimized towards its own local optima instead of solving the global objective, which results in forgetting the global knowledge and raises a drift across client updates. Some previous methods leverage knowledge distillation (KD) to avoid the federated forgetting, but most of them do not consider the global teacher model’s ability on different categories and might mislead the local student models’ training consequently. To address this issue, we propose a Class-wise Adaptive self-Distillation method for Federated Learning, which is named FedCAD. Before local training at each round, FedCAD assesses the inference confidence on each category of the global model using an auxiliary dataset, which is used to indicate how much the global model should be trusted. Based on the assessments, a class-wise adaptive weight is used to dynamically adjust the impact of the global teacher model on the local training of each category. In this way, the distilled knowledge from the global teacher can be selectively learned by the local students to avoid negative impacts. The extensive experimental results on the public datasets, i.e., CIFAR10, CIFAR100 and FEMINST, demonstrate that the proposed FedCAD has better performance in terms of convergence speed and classification accuracy, compared to other state-of-the-art FL methods.
更多查看译文
AI 理解论文
溯源树
样例
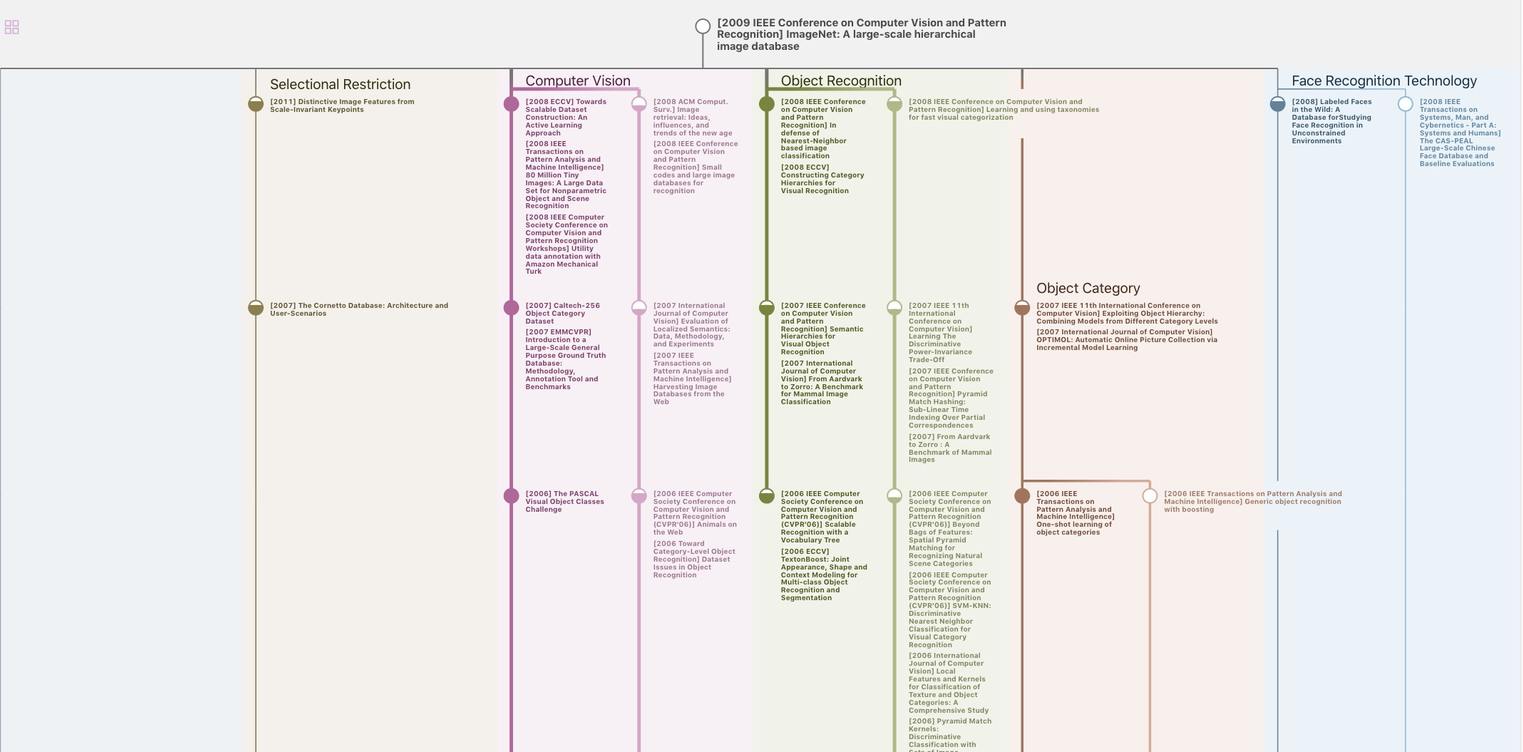
生成溯源树,研究论文发展脉络
Chat Paper
正在生成论文摘要