A Conversational Paradigm for Program Synthesis.
CoRR(2022)
摘要
Program synthesis strives to generate a computer program as a solution to a given problem specification. We propose a conversational program synthesis approach via large language models, which addresses the challenges of searching over a vast program space and user intent specification faced in prior approaches. Our new approach casts the process of writing a specification and program as a multi-turn conversation between a user and a system. It treats program synthesis as a sequence prediction problem, in which the specification is expressed in natural language and the desired program is conditionally sampled. We train a family of large language models, called C ODE G EN , on natural language and programming language data. With weak supervision in the data and the scaling up of data size and model size, conversational capacities emerge from the simple autoregressive language modeling. To study the model behavior on conversational program synthesis, we develop a multi-turn programming benchmark (MTPB), where solving each problem requires multi-step synthesis via multi-turn conversation between the user and the model. Our findings show the emergence of conversational capabilities and the effectiveness of the proposed conversational program synthesis paradigm. In addition, our model C ODE G EN (with up to 16B parameters trained on TPU-v4) outperforms OpenAI’s Codex on the HumanEval benchmark. We make the training library JAX FORMER including checkpoints available as open source contribution: https://github.com/salesforce/CodeGen . to achieve program synthesis: (1) the vastness of the search space, and (2) the difficulty of properly specifying user intent. In an attempt to address these challenges, we propose and train C ODE G EN , an interactive code generation model for program synthesis. In addition, we develop a new multi-turn programming benchmark to investigate the programming synthesis capacities of C ODE G EN . enhances as a function of the model size and data size. It suggests that the capacity of conversational program synthesis scales as a function of the model size and data size. The models are simply trained with an autoregressive language modeling objective. While the model and the data scale up, conversational capacity emerges.
更多查看译文
AI 理解论文
溯源树
样例
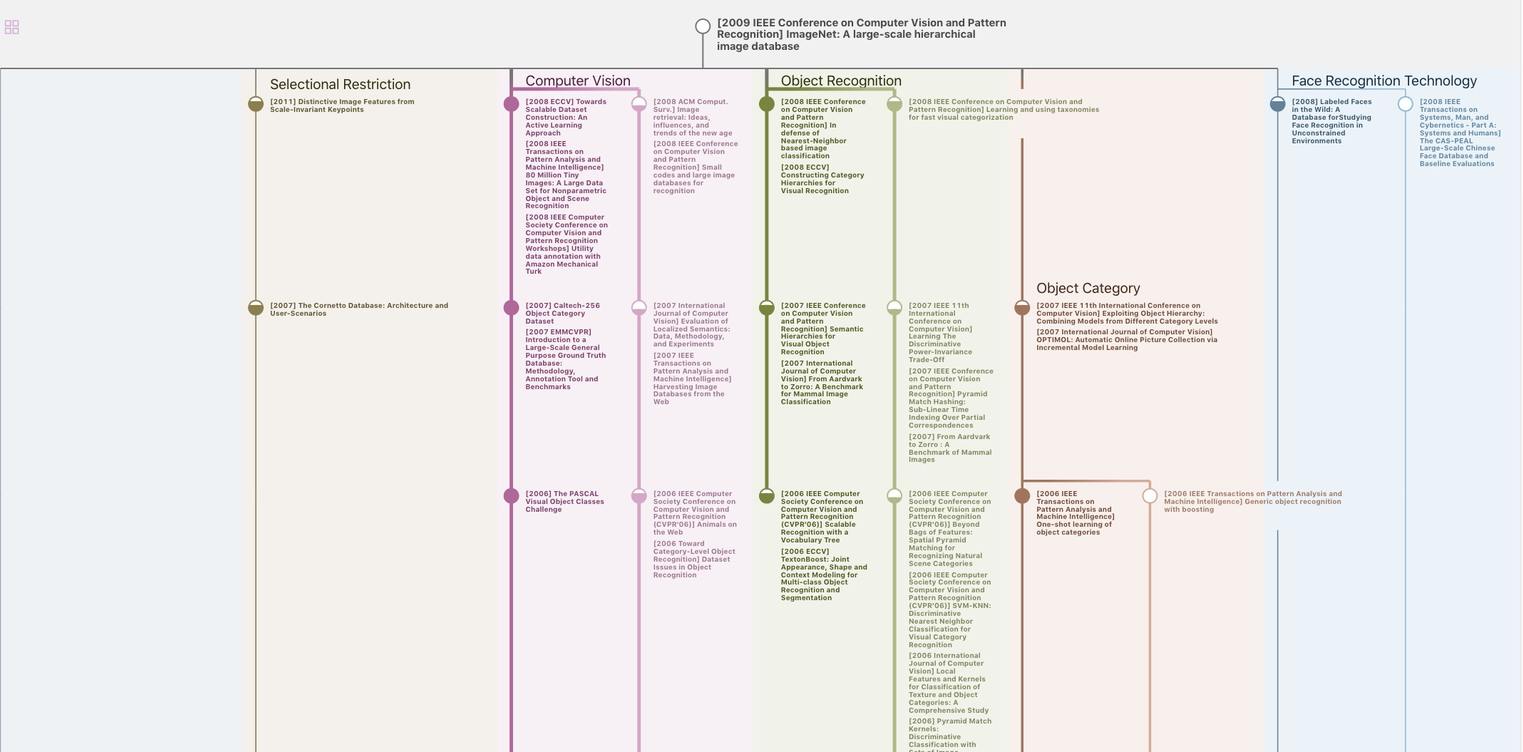
生成溯源树,研究论文发展脉络
Chat Paper
正在生成论文摘要