Inferring Space Object Orientation with Spectroscopy and Convolutional Networks
semanticscholar(2021)
摘要
Accurate inference of a space object’s orientation is imperative for deriving its operational status and coordinating effective space traffic management at large. To formulate the framework necessary for solving the problem of orientation inference, we analyze several standard mathematical representations of rotation with an emphasis on continuity, uniqueness, and deep learning efficacy. On this basis, we are naturally led to the implementation of a lesser known but well-behaved 6D representation of rotation. For the input of our inference models, we employ a distance-invariant observational technique that has long been used to probe the furthest reaches of the universe at the smallest scales – spectroscopy. Facilitated by deep convolutional neural networks (CNN’s), we investigate the viability of using simulated raw, long slit spectroscopic images to infer the orientation of space objects in the nonresolved regime of large orbital radii. We present methods and results of training CNN’s on spectral images of several space objects with an aim to i) standardize the measures used in rotation analysis, ii) establish an upper bound on spectral-based performance, and iii) provide a simple-scenario baseline for the extension of future work in the application of spectroscopy to space domain awareness.
更多查看译文
AI 理解论文
溯源树
样例
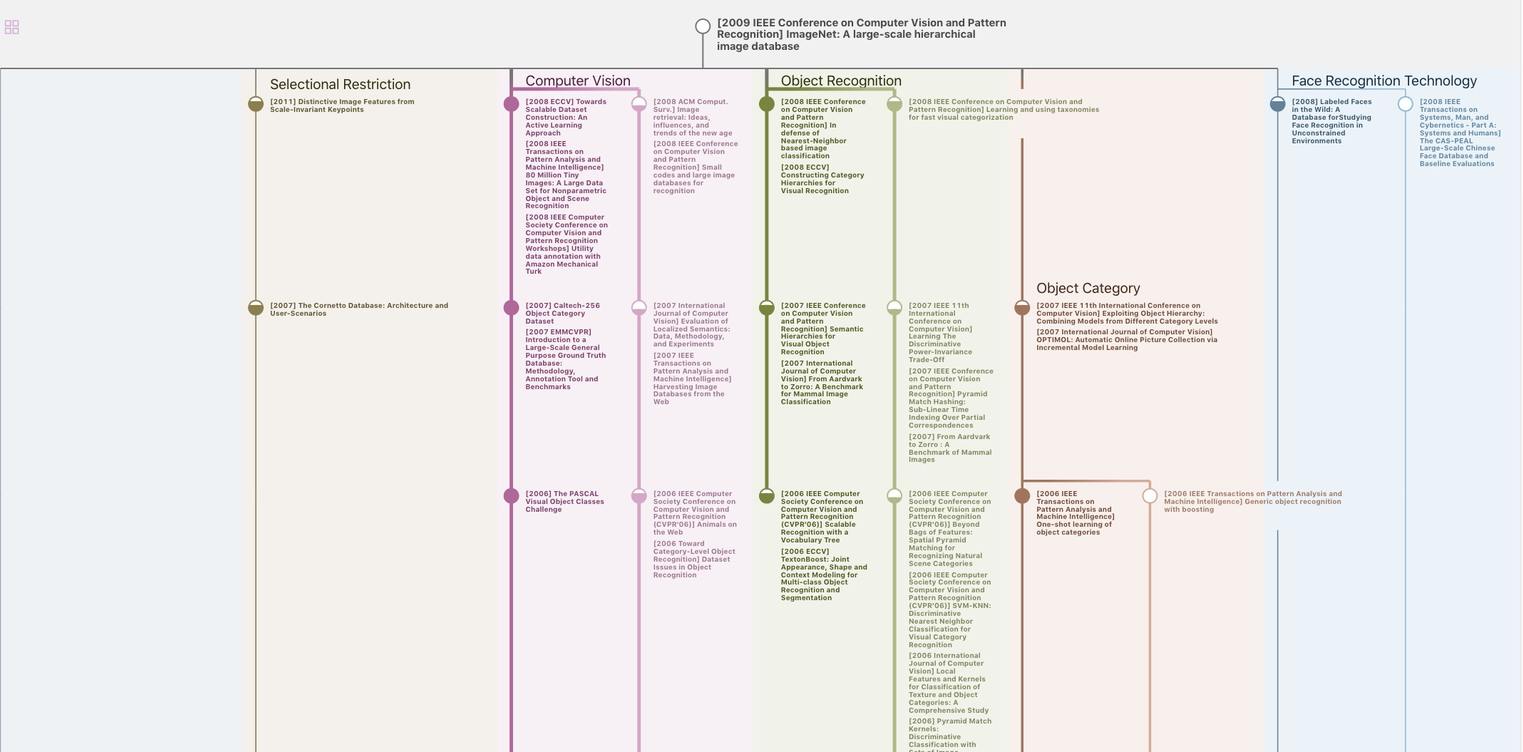
生成溯源树,研究论文发展脉络
Chat Paper
正在生成论文摘要