Lagrangian Observations and Modeling of Turbulence Along a Tidally Influenced River
WATER RESOURCES RESEARCH(2022)
摘要
We examine and model the patterns of velocities and turbulence from flow-following measurements along a tidally influenced river, collected from the heavily sediment-laden Kaipara River, New Zealand, using Lagrangian 'Floc Drifter' platforms released at different locations and times. Numerical modeling was undertaken in Delft3D with the k-epsilon turbulence closure scheme. While model calibration using Eulerian measurements was classified as excellent, results explored in a Lagrangian framework revealed deficiencies in model performance. Generally, the model reproduced flow speeds and patterns of dissipation rates of turbulent kinetic energy epsilon of the right order of magnitude, but did not always correctly reproduce the observed epsilon, particularly around abrupt meander bends. These discrepancies were attributed partly to errors in velocity predictions, but also indicated that other processes (omitted or not accurately represented in our model) such as wind-driven mixing and secondary flow strongly influenced turbulence dynamics. Predicted epsilon were relatively consistent across different tidal phases and days, suggesting the bathymetry and geometry of the river were the greatest control on the along-river structure of turbulence. The vertical and cross-sectional distributions of turbulent quantities (epsilon, k and nu) displayed larger variations vertically than longitudinally and agree with previous studies. Results show that flow-following measurements offer a stringent validation for numerical modeling of hydrodynamics over large (riverine) spatial scales at a temporal and spatial resolution not possible with traditional Eulerian measurements. Ultimately, robust numerical predictions of velocities and turbulence are critical to accurately model and predict the dispersal and fate of fine-sediments in aquatic environments.
更多查看译文
关键词
turbulence modeling, Lagrangian, river
AI 理解论文
溯源树
样例
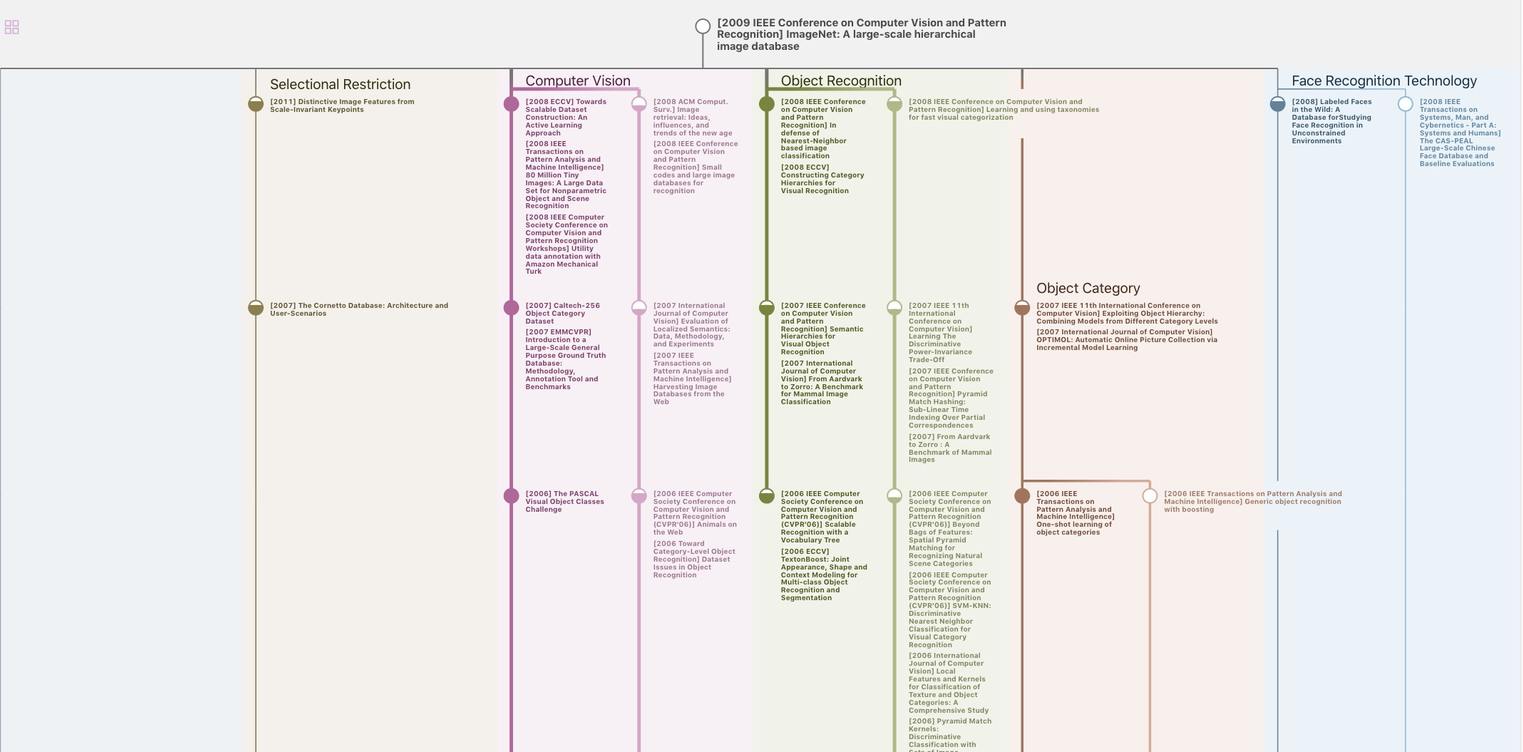
生成溯源树,研究论文发展脉络
Chat Paper
正在生成论文摘要