Out-of-Distribution Generalization with Deep Equilibrium Models
semanticscholar(2021)
Abstract
Deep learning models often make unexpected mistakes under distribution shifts, preventing their widespread adoption in safety-critical applications. In this paper, we investigate whether Deep Equilibrium (DEQ) Models generalize better under systematic distribution shifts than their fixeddepth counterparts. We present two sets of experiments to address this question, both of which indicate that DEQ models enjoy superior outof-distribution generalization. We first observe, on various tasks, that DEQ models spend more time processing inputs of greater complexity, in a trend that extends predictably to levels of complexity larger than those observed during training. We then inspect how the internal representations of DEQ models derived from out-of-distribution (OOD) samples change as they approach equilibria. We find that the statistics of the internal representations of OOD samples are drawn closer to those derived from in-distribution samples in DEQ models, in sharp contrast to the behavior of fixed-depth architectures. Based on these results, we hypothesize that the convergence-based forward-pass termination criterion of DEQ models endows them with an inductive bias towards better out-of-distribution generalization.
MoreTranslated text
AI Read Science
Must-Reading Tree
Example
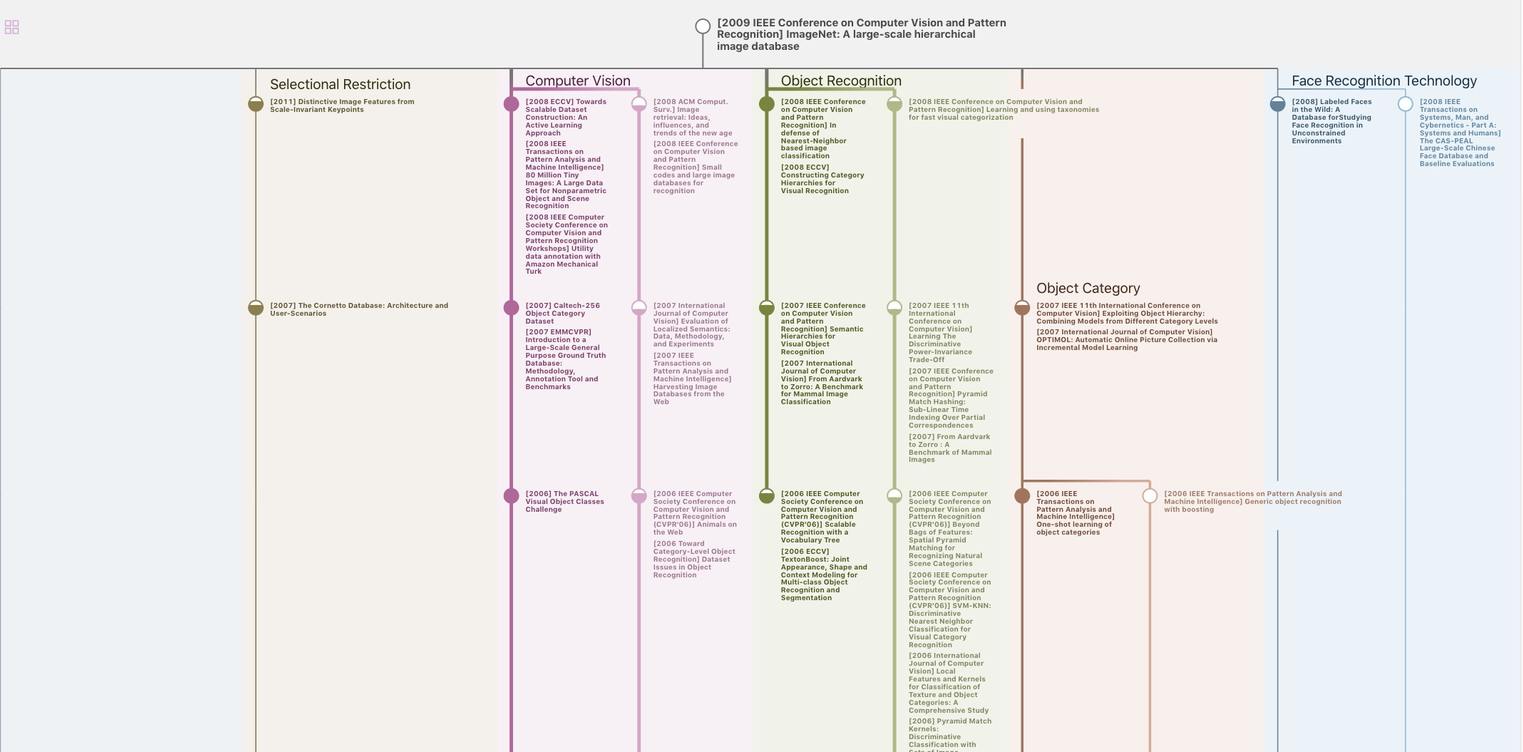
Generate MRT to find the research sequence of this paper
Chat Paper
Summary is being generated by the instructions you defined