Interaction Between Human And Explanation in Explainable AI System For Cancer Detection and Preliminary Diagnosis
semanticscholar(2021)
摘要
Nowadays, Artificial Intelligence (AI) systems are everywhere and AI helps to make decisions for us is our daily occurrence. AI provides for us from recommendations product on Amazon and video recommendations on YouTube, to tailored advertisements on Google search result pages. Even though they appear powerful in terms of results and predictions, AI algorithms suffer from transparency problem. Modern AI algorithms are complex and difficult to get the reasoning and the insight into AI algorithms work mechanism. However, in critical decisions that involves individuals well-being such as disease diagnosis or prognosis, it is important to know the reasons behind such a critical decision. An emerging research area called Explainable AI (XAI) looks at how to solve this problem by providing a layer of explanation which helps end users to make sense of AI results. The overall assumption behind XAI research is that explicability can improve trust and social acceptability of AI assisted predictions. In our research, we specifically look at cancer detection and diagnosis and hypothesize that appropriately designed Explainable AI systems can improve trust in AI assisted medical predictions.
更多查看译文
AI 理解论文
溯源树
样例
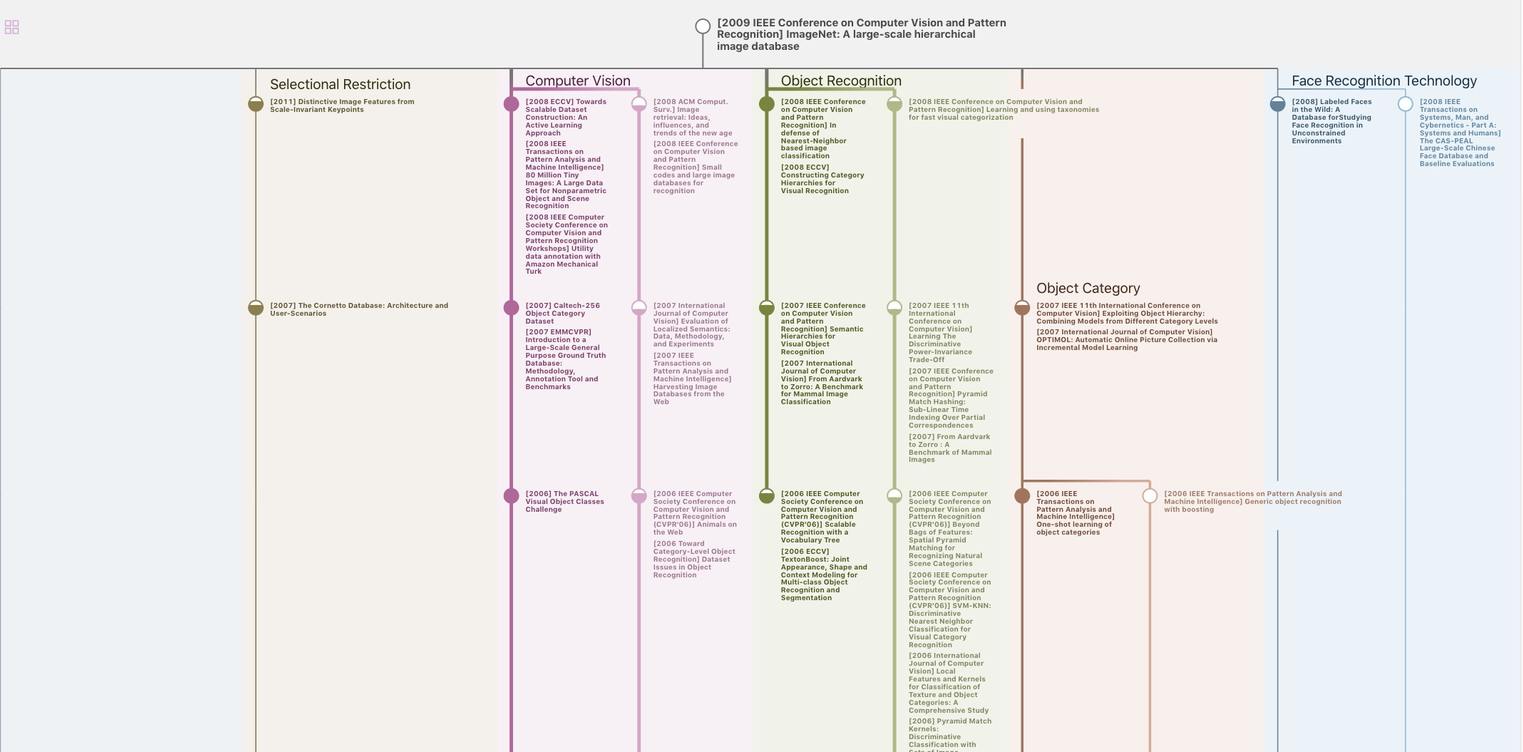
生成溯源树,研究论文发展脉络
Chat Paper
正在生成论文摘要