Beyond Value: C HECK L IST for Testing Inferences in Planning-Based RL
International Conference on Automated Planning and Scheduling(2022)
摘要
Reinforcement learning (RL) agents are commonly evaluated via their expected value over a distribution of test scenarios. Unfortunately, this evaluation approach provides limited evi- dence for post-deployment generalization beyond the test distribution. In this paper, we address this limitation by extend- ing the recent C HECK L IST testing methodology from natural language processing to planning-based RL. Specifically, we consider testing RL agents that make decisions via on-line tree search using a learned transition model and value function. The key idea is to improve the assessment of future performance via a C HECK L IST approach for exploring and assessing the agent’s inferences during tree search. The approach provides the user with an interface and general query- rule mechanism for identifying potential inference flaws and validating expected inference invariances. We present a user study involving knowledgeable AI researchers using the approach to evaluate an agent trained to play a complex real- time strategy game. The results show the approach is effective in allowing users to identify previously-unknown flaws in the agent’s reasoning. In addition, our analysis provides insight into how AI experts use this type of testing approach, which may help improve future instantiations.
更多查看译文
关键词
Testing,Reinforcement Learning,Trust
AI 理解论文
溯源树
样例
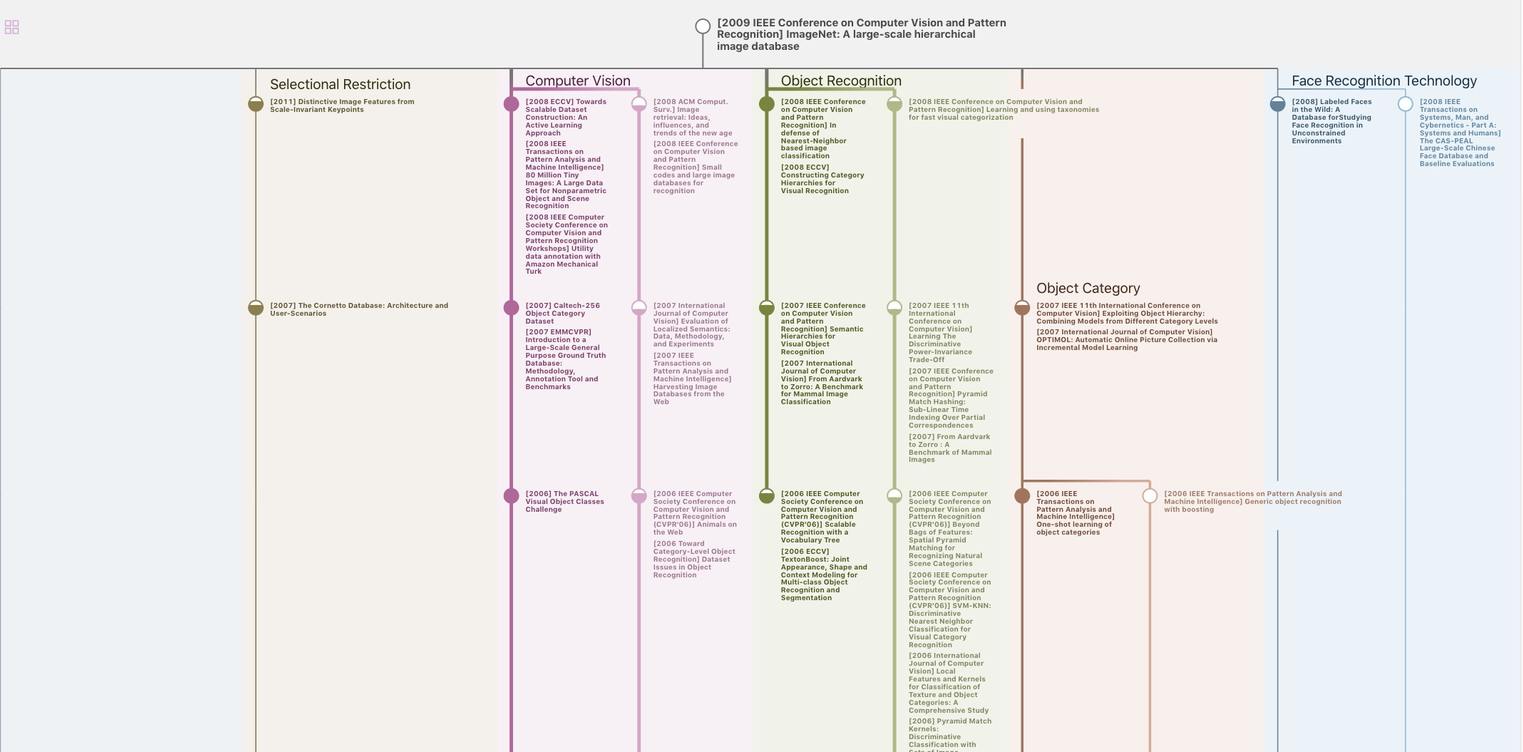
生成溯源树,研究论文发展脉络
Chat Paper
正在生成论文摘要