Optimized weighted local kernel features for hyperspectral image classification
Multimedia Tools and Applications(2022)
摘要
Hyperspectral images (HSIs) have been widely used to study earth surface phenomena. Traditionally, spectral features have been used to classify hyperspectral images, which result in noisy classified maps. Researchers have demonstrated that spatial information, such as local covariance matrix features, can enhance classification accuracy by providing complementary information. It can be proved that the covariance matrix is represented as the linear kernel function and can only model linear dependencies and does not contain any information about higher-order statistics that are important in HSI classification. To address this issue, this paper proposes a new method for classifying hyperspectral images based on weighted local kernel matrix features (WLKM) and improved binary grey wolf optimization (IBGWO). Spatial features, with the capability of modeling complicated nonlinear relationships between features, are first produced based on WLKM features. Then, the proposed method follows an improved binary grey wolf optimization algorithm based on a modified crossover operator to select the most informative features from spatial-spectral features vectors which are resulted from stacked WLKM and spectral features. Experiments on the three hyperspectral images named Indian Pines, Pavia University, and Salinas show that the proposed method can efficiently classify HSI and has better performance than some state-of-the-art spatial-spectral classification methods.
更多查看译文
关键词
Hyperspectral, Classification, Kernel matrix, Covariance, Feature extraction
AI 理解论文
溯源树
样例
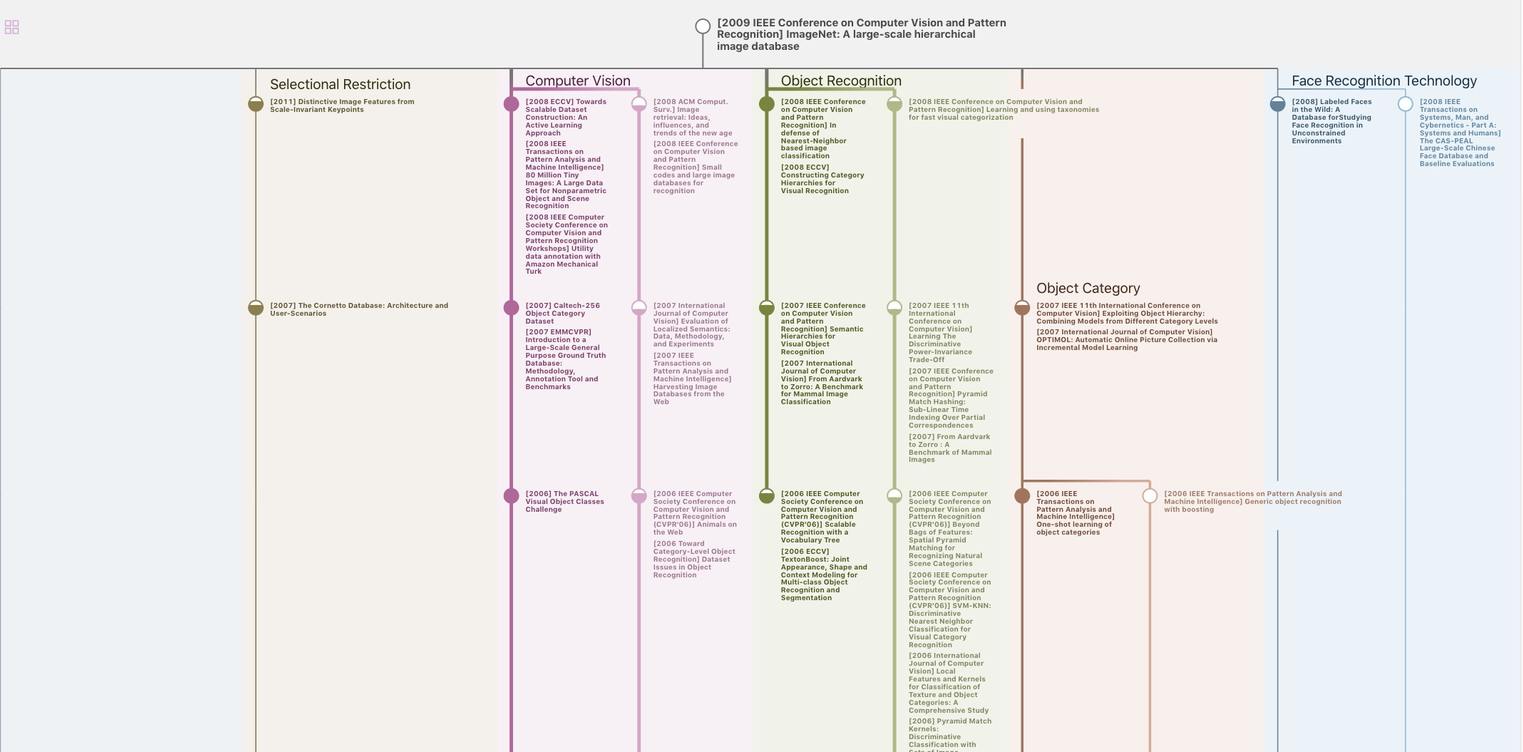
生成溯源树,研究论文发展脉络
Chat Paper
正在生成论文摘要