A continuous vertically resolved ozone dataset from the fusion of chemistry climate models with observations using a Bayesian neural network
EarthArXiv (California Digital Library)(2021)
摘要
Continuous historic datasets of vertically resolved stratospheric ozone, support the case for ozone recovery, are necessary for the running of offline models and increase understanding of the impacts of ozone on the wider atmospheric system. 5 Vertically resolved ozone datasets are typically constructed from multiple satellite, sonde and ground-based measurements that do not provide continuous coverage. As a result, several methods have been used to infill these gaps, most commonly relying on regression against observed time series. However, these existing methods either provide low accuracy infilling especially over polar regions, unphysical extrapolation, or an incomplete estimation of uncertainty. To address these methodological shortcomings we used and further developed an infilling framework that fuses observations with output from an ensemble of 10 chemistry-climate models within a Bayesian neural network. We used this deep learning framework to produce a continuous record of vertically resolved ozone with uncertainty estimates. Under rigorous testing the infilling framework extrapolated and interpolated skillfully and maintained realistic interannual variability due to the inclusion of physically and chemically realistic models. This framework and the ozone dataset it produced, enables a more thorough investigation of vertically resolved trends throughout the atmosphere. 15
更多查看译文
关键词
chemistry climate models,bayesian neural network,ozone,neural network
AI 理解论文
溯源树
样例
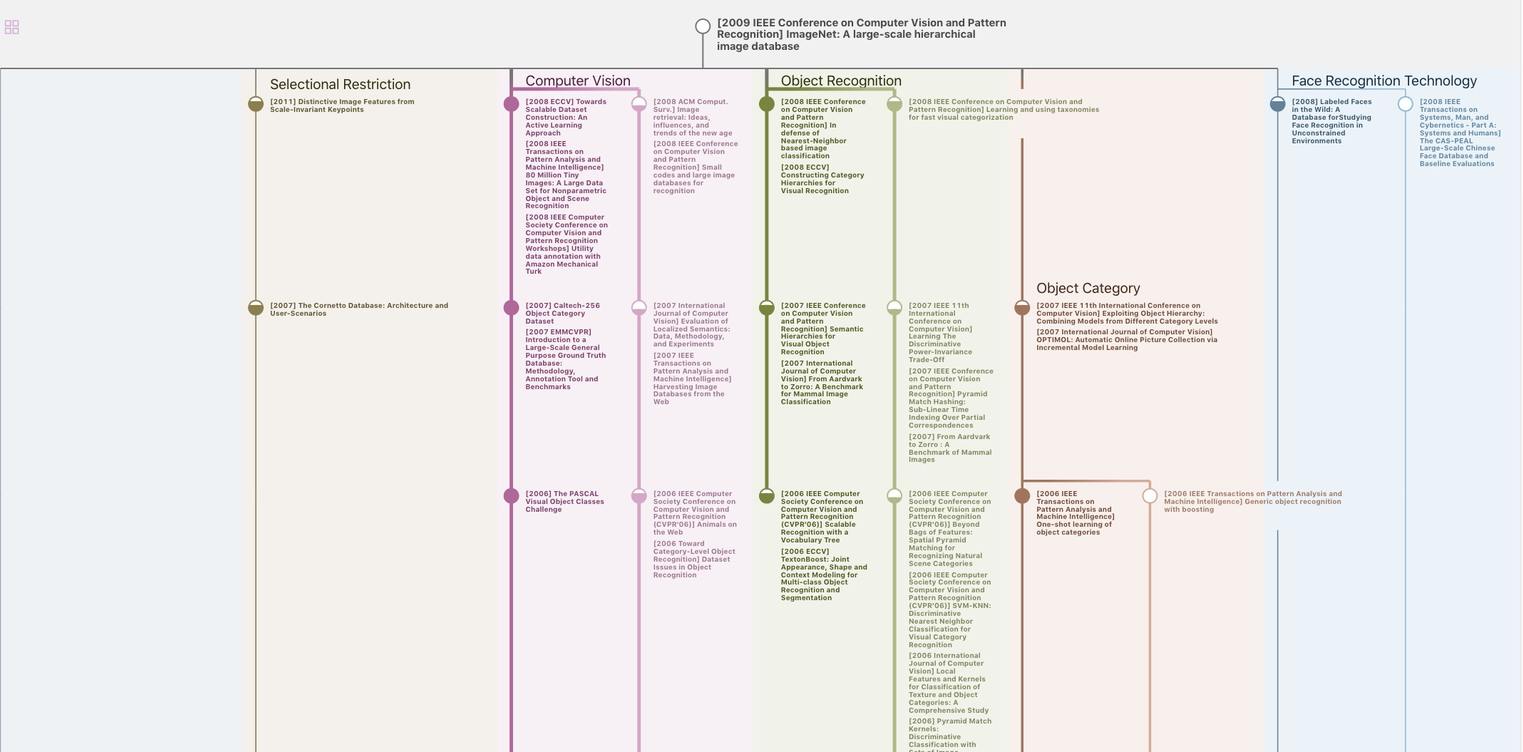
生成溯源树,研究论文发展脉络
Chat Paper
正在生成论文摘要