Hierarchical Topic Evaluation: Statistical vs. Neural Models
semanticscholar(2021)
摘要
Hierarchical topic models (HTMs)—especially those based on Bayesian deep learning—are gaining increasing attention from the ML community. However, in contrast to their flat counterparts, their proper evaluation is rarely addressed. We propose several measures to evaluate HTMs in terms of their (branch-wise and layer-wise) topic hierarchy. We apply these measures to benchmark several HTMs on a wide range of datasets. We compare neural HTMs to traditional statistical HTMs in topic quality and interpretability. Our findings may help better judge advantages and disadvantages in different deep hierarchical topic models and drive future research in this area.
更多查看译文
AI 理解论文
溯源树
样例
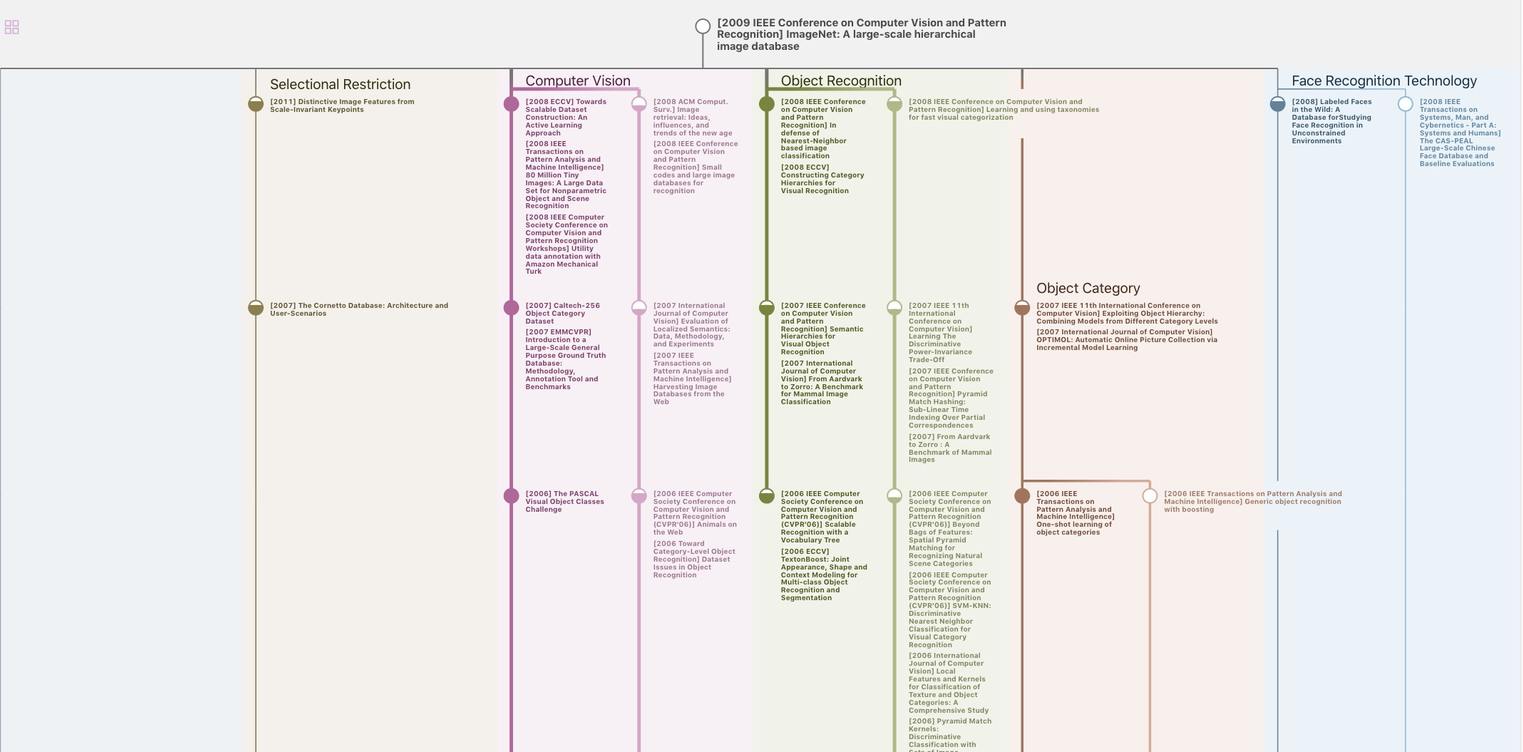
生成溯源树,研究论文发展脉络
Chat Paper
正在生成论文摘要