Attention-based fusion factor in FPN for object detection
Applied Intelligence(2022)
摘要
At present, most advanced detectors usually use the feature pyramid to detect objects of different scales. Among them, FPN is one of the representative works of multi-scale feature summation to construct the feature pyramid. However, the existing FPN-based feature extraction networks pay more attention to capturing effective semantic information and ignore the influence of the dataset scale distribution on the FPN feature fusion process. To solve this problem, we propose a novel attention structure, which can be applied to any FPN-based network model. Different from the general attention that gets its own attention from itself, our proposed method makes better use of the influence of the lower layer feature of the adjacent layer on feature fusion, which guides the filtering of the upper layer feature. By considering the difference in the feature information of the same sample in different feature maps, it is better to filter out the invalid sample features of the upper layer relative to the lower layer. Our method can better learn the degree of deep features participating in shallow learning so that each layer of FPN is more focused on its own layer learning while effectively transferring features. Our experimental results show that our method can significantly improve the multi-scale object detection performance of the model.
更多查看译文
关键词
Deep learning,Object detection,Attention mechanism,Multi-scale
AI 理解论文
溯源树
样例
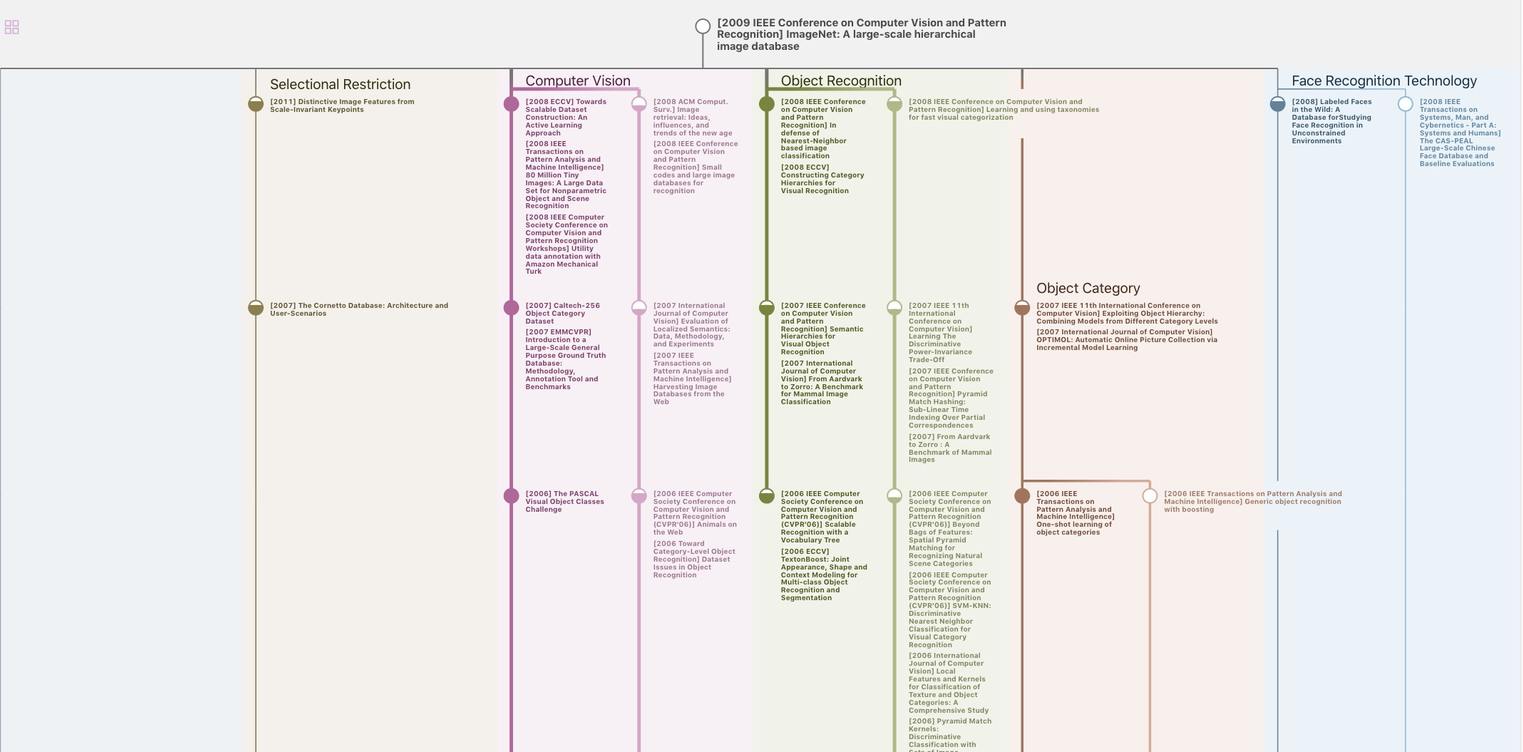
生成溯源树,研究论文发展脉络
Chat Paper
正在生成论文摘要