Using a long short-term memory (LSTM) neural network to boost river streamflow forecasts over the western United States
HYDROLOGY AND EARTH SYSTEM SCIENCES(2022)
摘要
Abstract. Accurate river streamflow forecasts are a vital tool in the fields of water security, flood preparation and agriculture, as well as in industry more generally. Over the last century, physics-based models traditionally used to produce streamflow forecasts have become increasingly sophisticated, with forecasts improving accordingly. However, the development of such models is often bound by two soft limits: empiricism – many physical relationships are represented by computationally efficient empirical formulae; and data sparsity – the calibration of these models often requires long time-series of high-resolution observational data at both surface and subsurface levels. Artificial neural networks have previously been shown to be highly effective at simulating nonlinear systems where knowledge of the underlying physical relationships is incomplete. However, they also suffer from issues related to data sparsity. Recently, hybrid forecasting systems, which combine the traditional physics-based approach with statistical forecasting techniques, have been investigated for use in hydrological applications. In this study, we test the efficacy of a type of neural network, the long-short term memory (LSTM), at predicting streamflow at ten river gauge stations across various climatic regions of the western United States. The LSTM is trained on the catchment-mean meteorological and hydrological variables from the ERA5 and GloFAS-ERA5 reanalysis as well as historical streamflow observations. The performance of these hybrid forecasts is evaluated and compared to the performance of both raw and bias-corrected output from the Copernicus Emergency Management Service (CEMS) physics-based Global Flood Awareness System (GloFAS). Two periods are considered, a testing phase (June 2019 to June 2020), during which the models were fed with ERA5 data to investigate how well they simulated streamflow at the ten stations; and an operational phase (September 2020 to October 2021), during which the models were fed forecast variables from ECMWF's Integrated Forecast System (IFS), to investigate how well they could predict streamflow at lead times of up to ten days. All three models performed well in the testing phase, with the LSTM performing the best (skilful at nine stations, of which six were highly skilful). Similarly, the LSTM forecasts beat the raw and bias-corrected GloFAS forecasts during the operational phase, with skilful 5-day forecasts at nine stations, of which five were highly skilful. Implications and potential improvements to this work are discussed. In summary, this is the first time an LSTM has been used in a hybrid system to create a medium-range streamflow forecast, and in beating established physics-based models, shows promise for the future of neural networks in hydrological forecasting.
更多查看译文
关键词
streamflow forecasts,lstm,river,neural network,short-term
AI 理解论文
溯源树
样例
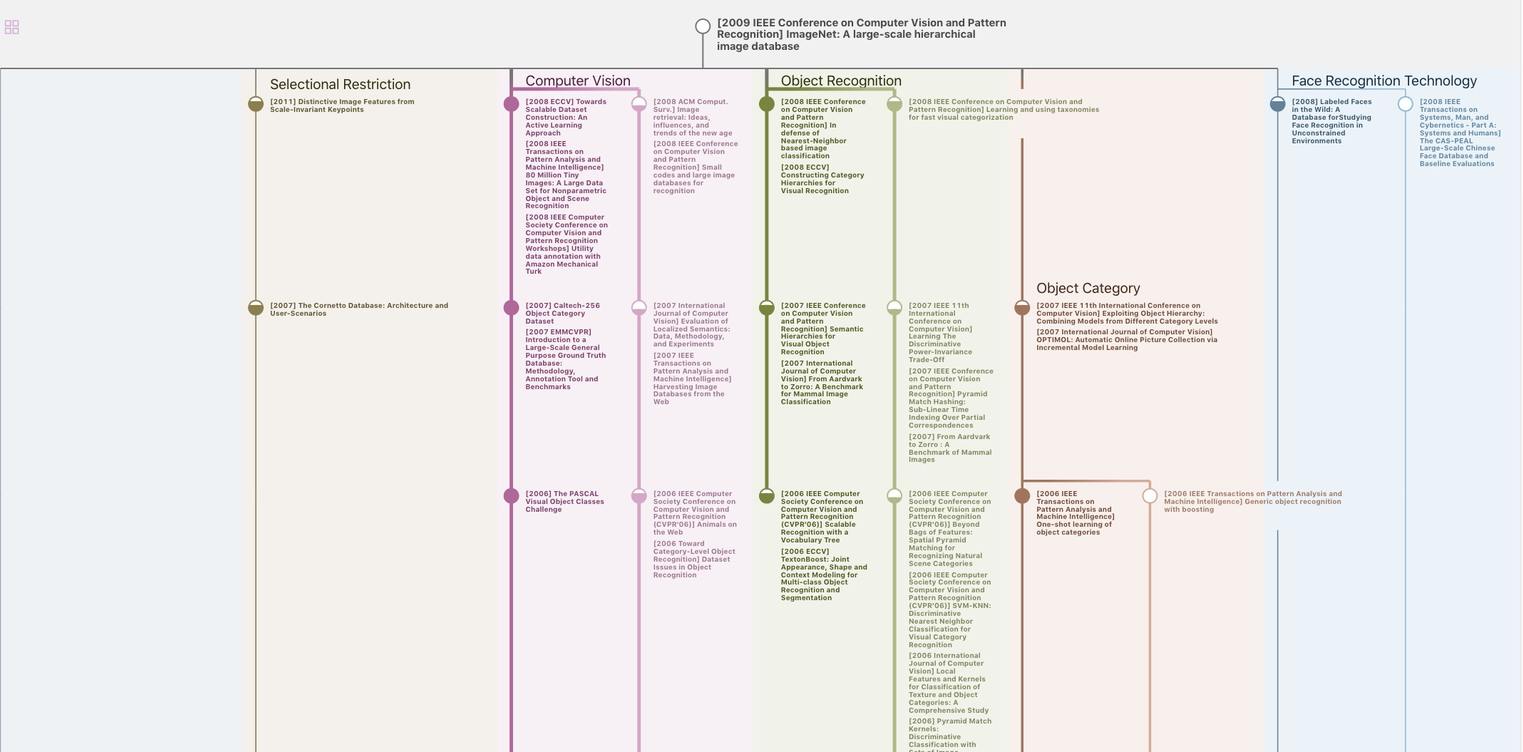
生成溯源树,研究论文发展脉络
Chat Paper
正在生成论文摘要