A coevolution algorithm based on two-staged strategy for constrained multi-objective problems
Applied Intelligence(2022)
摘要
Constrained Multiobjective Problem (CMOP) is widely used in engineering applications, but the current constrained Multiobjective Optimization algorithms (CMOEA) often fails to effectively balance convergence and diversity. For this purpose, a two-stage co-evolution constrained multi-objective optimization evolutionary algorithm (TSC-CMOEA) is presented to solve constrained multi-objective optimization problems. This method divides the search process into two phases: in the first stage, the synchronous co-evolution is used, and the population corresponding to the help problem and the population corresponding to the raw problem cooperate with each other and share the offspring to produce better solutions, so as to quickly cross the infeasible region and approach the Pareto front; The second stage discards the help problem when it fails and maintains only the evolution of the main population to save computing resources and enhance convergence. The combination of synchronous co-evolution and staged strategy allows the population to traverse infeasible regions more efficiently and converge quickly to feasible and non-dominant regions. The test results on benchmark CMOPs show that the convergence and population distribution of TSC-CMOEA is significantly better than those of NSGA-II, NSGA-III, C-MOEA/D, PPS, ToP and CCMO.
更多查看译文
关键词
Constraint handling technique, Multiobjective optimization, Coevolution, Constrained multi-objective evolutionary algorithms
AI 理解论文
溯源树
样例
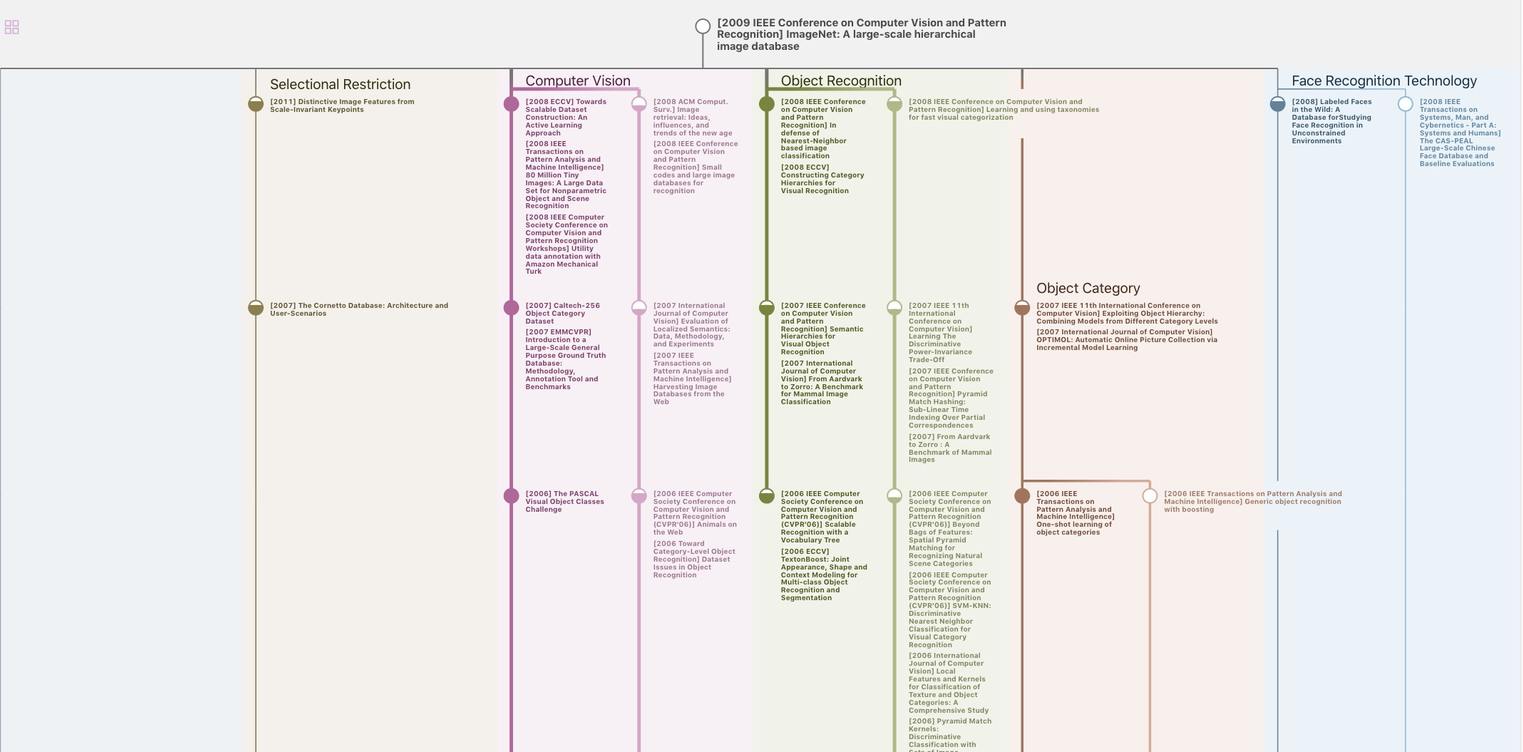
生成溯源树,研究论文发展脉络
Chat Paper
正在生成论文摘要