Enabling Fast and Flexible Distributed Deep Learning with Programmable Switches
CoRR(2022)
摘要
Deep learning has been used in a wide range of areas and made a huge breakthrough. With the ever-increasing model size and train-ing data volume, distributed deep learning emerges which utilizes a cluster to train a model in parallel. Unfortunately, the performance is often far from linear speedup due to the communication overhead between cluster nodes. To address this challenge, this paper designs and implements Libra, a network aggregator, that utilizes in-network computation to optimize the communication for distributed DL training in two aspects: 1) reduce active connections and 2) aggregate exchanged network packets. We implemented our Libra on Intel Tofino switches, customized a lightweight host stack and integrated it into an open-source training framework PS-lite. The experimental result shows that our Libra can achieve 1.5~4 times speedup.
更多查看译文
AI 理解论文
溯源树
样例
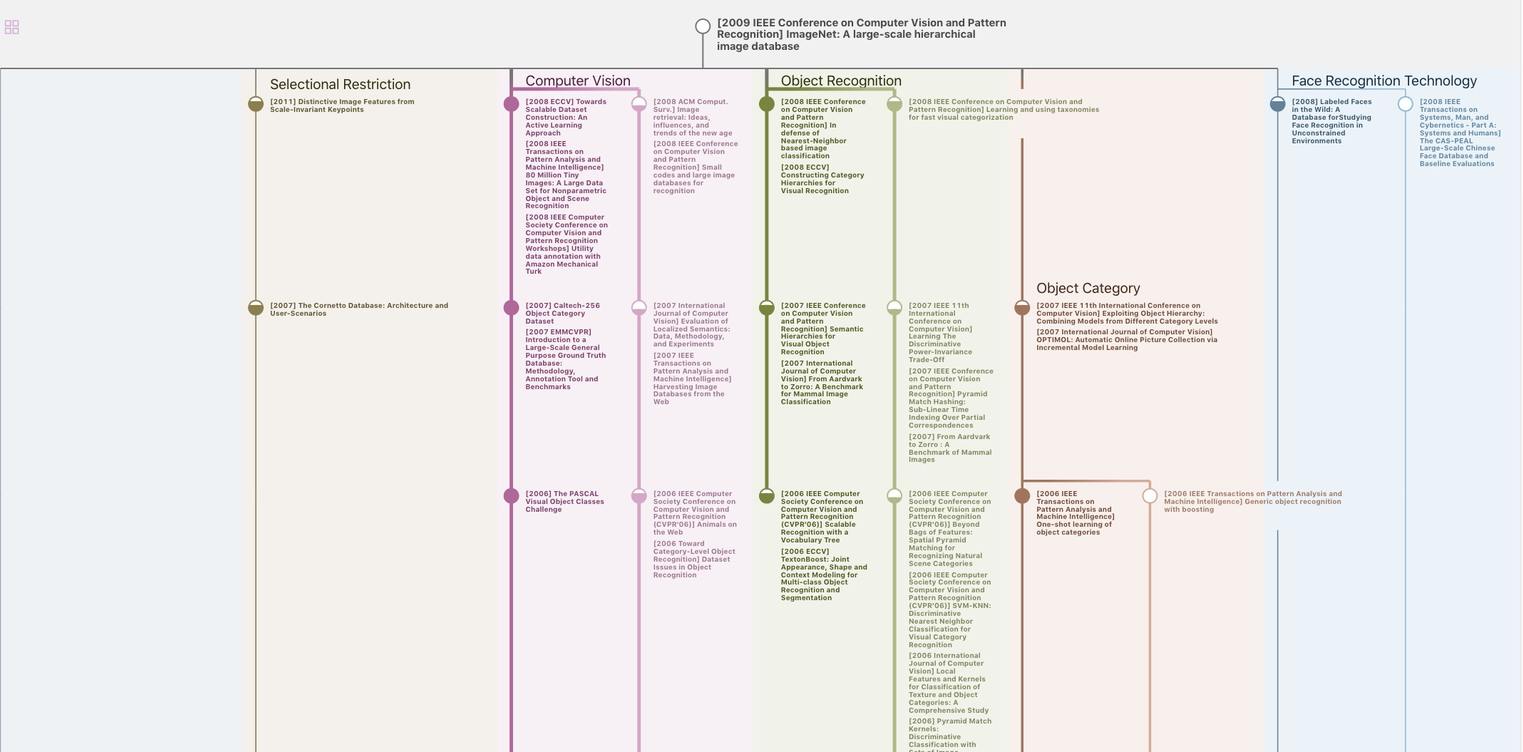
生成溯源树,研究论文发展脉络
Chat Paper
正在生成论文摘要