Beyond Bounding Box: Multimodal Knowledge Learning for Object Detection
arxiv(2022)
摘要
Multimodal supervision has achieved promising results in many visual language understanding tasks, where the language plays an essential role as a hint or context for recognizing and locating instances. However, due to the defects of the human-annotated language corpus, multimodal supervision remains unexplored in fully supervised object detection scenarios. In this paper, we take advantage of language prompt to introduce effective and unbiased linguistic supervision into object detection, and propose a new mechanism called multimodal knowledge learning (\textbf{MKL}), which is required to learn knowledge from language supervision. Specifically, we design prompts and fill them with the bounding box annotations to generate descriptions containing extensive hints and context for instances recognition and localization. The knowledge from language is then distilled into the detection model via maximizing cross-modal mutual information in both image- and object-level. Moreover, the generated descriptions are manipulated to produce hard negatives to further boost the detector performance. Extensive experiments demonstrate that the proposed method yields a consistent performance gain by 1.6\% $\sim$ 2.1\% and achieves state-of-the-art on MS-COCO and OpenImages datasets.
更多查看译文
关键词
multimodal knowledge learning,bounding box,detection
AI 理解论文
溯源树
样例
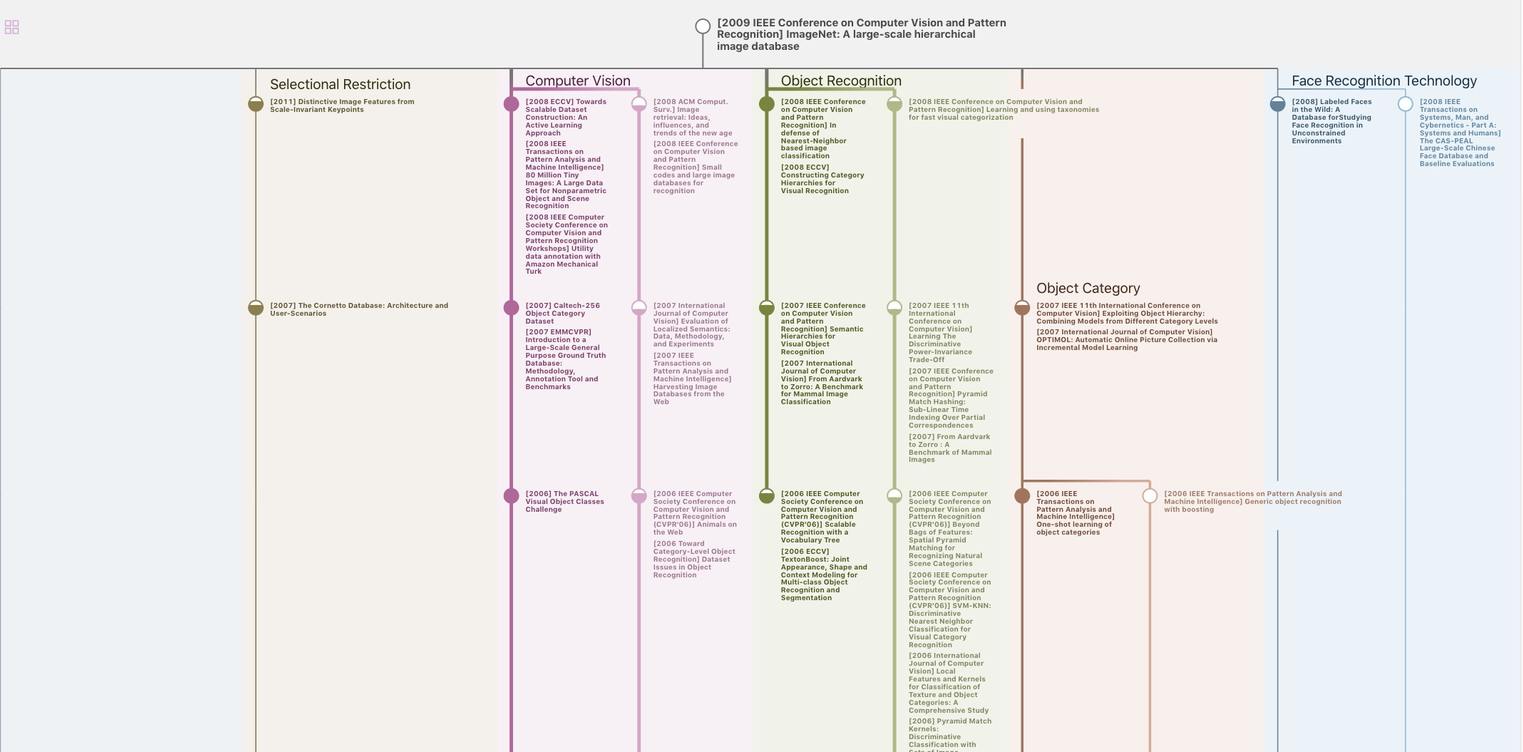
生成溯源树,研究论文发展脉络
Chat Paper
正在生成论文摘要