A deep belief network approach to remaining capacity estimation for lithium-ion batteries based on charging process features
Journal of Energy Storage(2022)
摘要
Accurate remaining capacity estimation for lithium batteries can help people understand the working state of a battery, which ensures the reliability and safety of electric equipment. Since the remaining capacities of lithium-ion batteries are related to internal physicochemical reactions, such as loss of lithium inventory and loss of active material, their remaining capacities are usually difficult to directly estimate. Advancements in deep learning have prompted the development of new data-driven approaches to solve this problem that can capture potential degradation correlation information from high-dimensional data via hidden layers of the deep learning model. This paper presents a novel deep learning method for lithium-ion battery capacity estimation based on charging process features with the following process. First, multiple health indicators are analysed and extracted according to different working conditions of batteries. Next, a grey relation analysis is combined with a cross-validation test, which is employed to eliminate information redundancy and improve prediction accuracy. Moreover, the optimized health indicators are further extracted through the restricted Boltzmann machine component of the deep belief network model, and a fully connected layer is adopted for estimation of lithium-ion battery capacity. A total of 23 battery datasets, including six working condition types, are employed for verification experiments. The maximum root mean square error of a single battery prediction is 3%, and that of multiple battery prediction is still within 6%, which confirms the effectiveness and accuracy of the proposed method.
更多查看译文
关键词
Lithium-ion battery,Deep belief network (DBN),Health indicator (HI),Sample entropy (SE),Capacity estimation
AI 理解论文
溯源树
样例
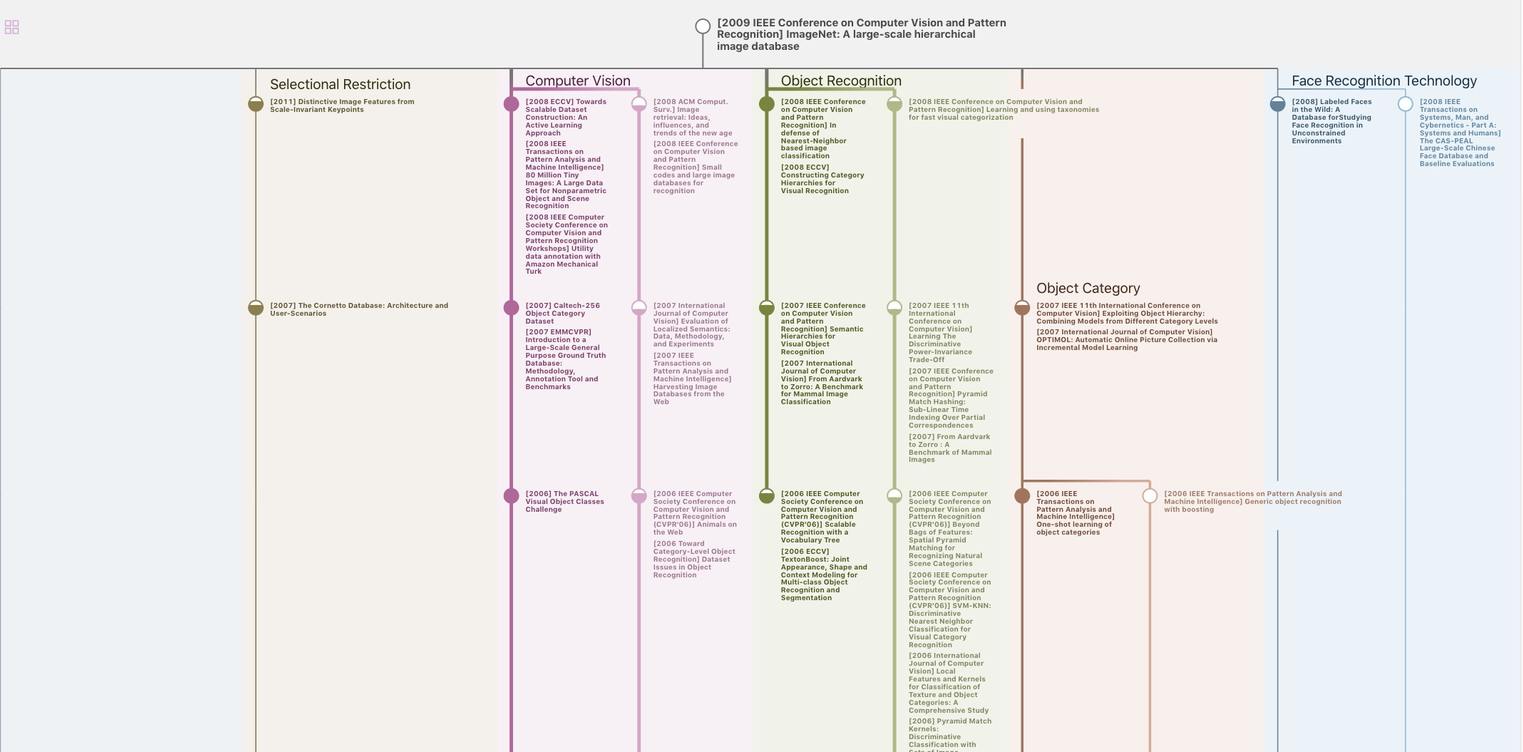
生成溯源树,研究论文发展脉络
Chat Paper
正在生成论文摘要