Prototype Feature Extraction for Multi-task Learning
International World Wide Web Conference(2022)
摘要
ABSTRACT Multi-task learning (MTL) has been widely utilized in various industrial scenarios, such as recommender systems and search engines. MTL can improve learning efficiency and prediction accuracy by exploiting commonalities and differences across tasks. However, MTL is sensitive to relationships among tasks and may have performance degradation in real-world applications, because existing neural-based MTL models often share the same network structures and original input features. To address this issue, we propose a novel multi-task learning model based on Prototype Feature Extraction (PFE) to balance task-specific objectives and inter-task relationships. PFE is a novel component to disentangle features for multiple tasks. To better extract features from original inputs before gating networks, we introduce a new concept, namely prototype feature center, to disentangle features for multiple tasks. The extracted prototype features fuse various features from different tasks to better learn inter-task relationships. PFE updates prototype feature centers and prototype features iteratively. Our model utilizes the learned prototype features and task-specific experts for MTL. We implement PFE on two public datasets. Empirical results show that PFE outperforms state-of-the-art MTL models by extracting prototype features. Furthermore, we deploy PFE in a real-world recommender system (one of the world’s top-tier short video sharing platforms) to showcase that PFE can be widely applied in industrial scenarios.
更多查看译文
关键词
Multi-task Learning, Recommender System, Neural Network
AI 理解论文
溯源树
样例
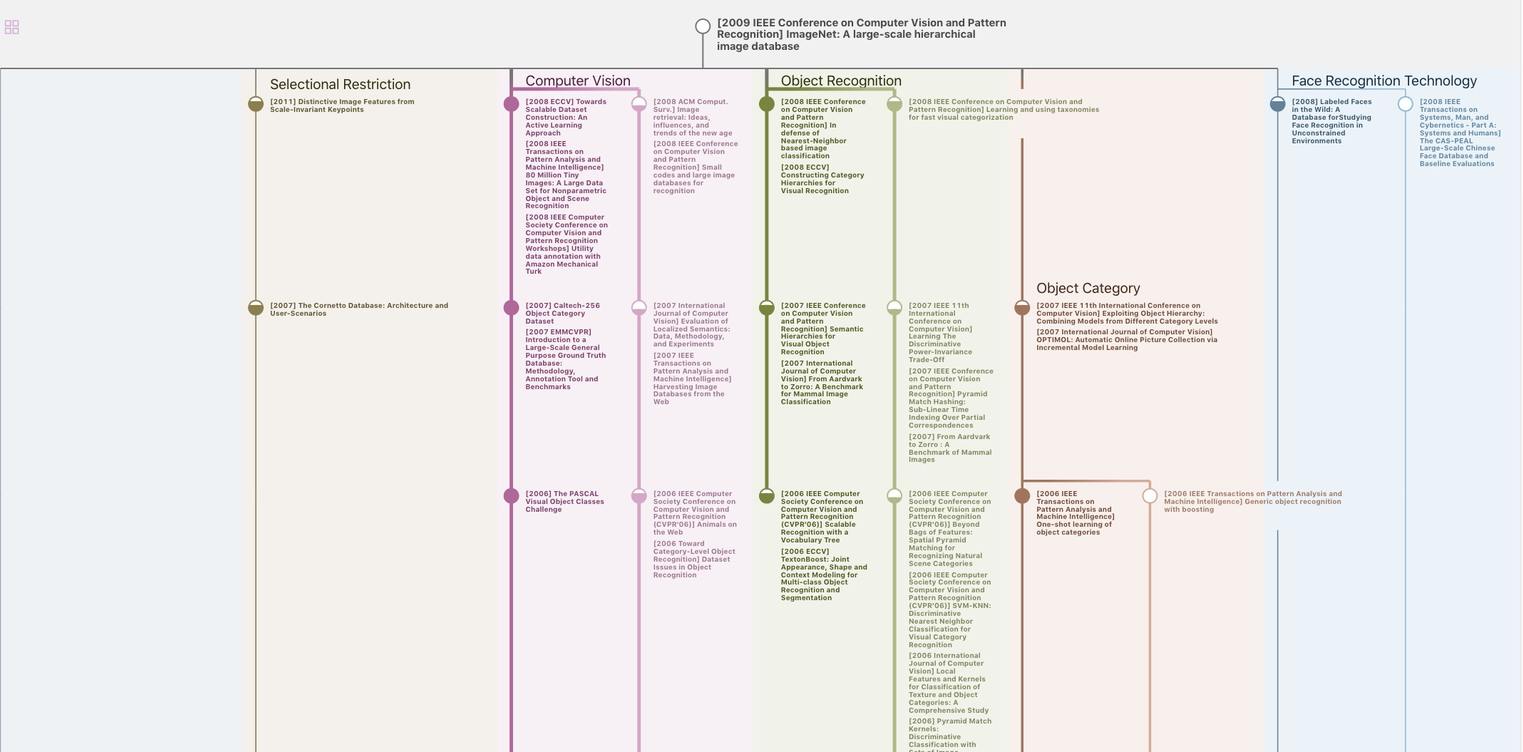
生成溯源树,研究论文发展脉络
Chat Paper
正在生成论文摘要