CycleNER: An Unsupervised Training Approach for Named Entity Recognition
International World Wide Web Conference(2022)
摘要
ABSTRACTNamed Entity Recognition (NER) is a crucial natural language understanding task for many down-stream tasks such as question answering and retrieval. Despite significant progress in developing NER models for multiple languages and domains, scaling to emerging and/or low-resource domains still remains challenging, due to the costly nature of acquiring training data. We propose CycleNER, an unsupervised approach based on cycle-consistency training that uses two functions: (i) sentence-to-entity – S2E and (ii) entity-to-sentence – E2S, to carry out the NER task. CycleNER does not require annotations but a set of sentences with no entity labels and another independent set of entity examples. Through cycle-consistency training, the output from one function is used as input for the other (e.g. S2E → E2S) to align the representation spaces of both functions and therefore enable unsupervised training. Evaluation on several domains comparing CycleNER against supervised and unsupervised competitors shows that CycleNER achieves highly competitive performance with only a few thousand input sentences. We demonstrate competitive performance against supervised models, achieving 73% of supervised performance without any annotations on CoNLL03, while significantly outperforming unsupervised approaches.
更多查看译文
关键词
natural language processing, named entity recognition, cycleconsistency, training, unsupervised training
AI 理解论文
溯源树
样例
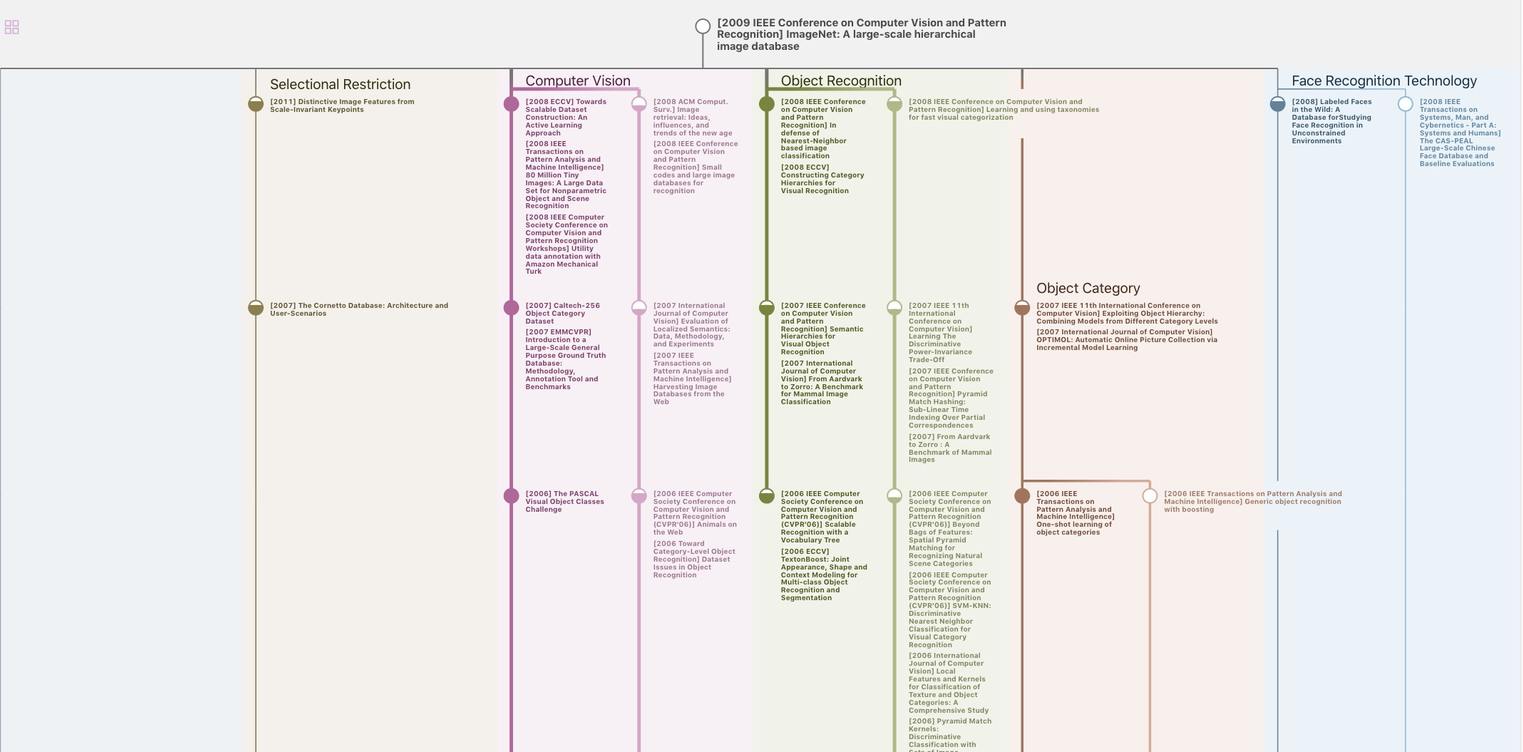
生成溯源树,研究论文发展脉络
Chat Paper
正在生成论文摘要