Downsampling for Binary Classification with a Highly Imbalanced Dataset Using Active Learning
Big Data Research(2022)
摘要
In many industrial applications, classification tasks are often associated with imbalanced class labels in training datasets. Imbalanced datasets can severely affect the accuracy of class predictions, and thus they need to be handled by appropriate data processing before analyzing the data since most machine learning techniques assume that the input data is balanced. In general, the skewness between class labels is managed by either increasing the number of samples in minorities or decreasing the number of samples in majorities. In this research, we are seeking to find a better way of downsampling by selecting the most informative samples in the given imbalanced dataset through the active learning strategy to mitigate the effect of imbalanced class labels. The data selection is performed by the criterion used in optimal experimental designs, from which the generalization error of the trained model is minimized sequentially, under the penalized logistic regression as a classification model. It is important to note that the informative samples can be either minority or majority instead of selecting majority samples only. This paper also suggests that the performance is improved especially with the highly imbalanced dataset, if tuning hyper-parameter λ and cost weights are applied to the active downsampling technique. The proposed algorithm shows better performance compared to other resampling methods with smaller sample sizes.
更多查看译文
关键词
Active learning,Imbalanced data,Downsampling,Penalized logistic regression,Cost weight
AI 理解论文
溯源树
样例
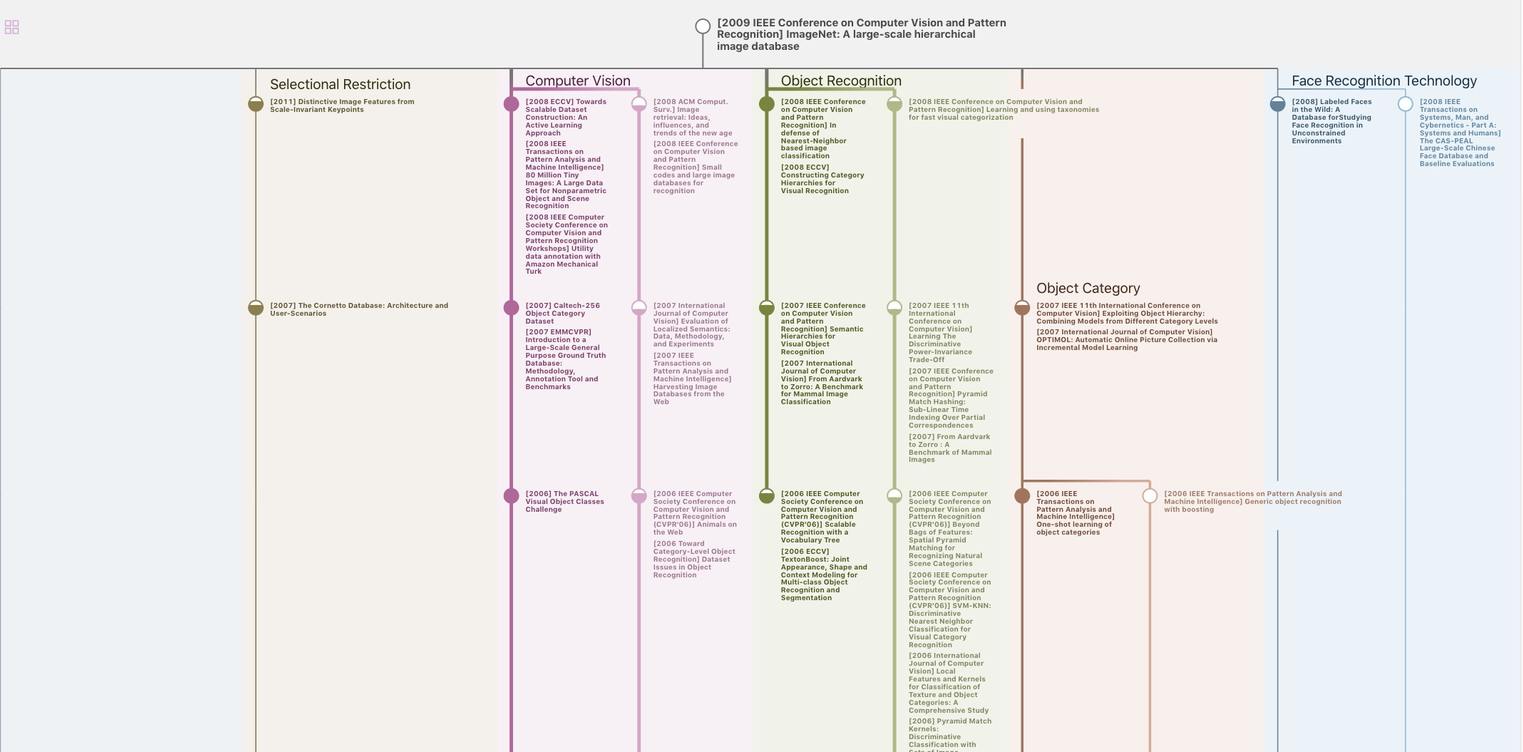
生成溯源树,研究论文发展脉络
Chat Paper
正在生成论文摘要