Adjoint-based optimization of a regional water elevation model
arxiv(2022)
摘要
Calibration of unknown model parameters is a crucial task in most ocean model applications. We present an adjoint-based optimization of a bottom friction coefficient in North Sea-Baltic Sea simulation. The system is modeled with an unstructured mesh finite element shallow water model. The adjoint model provides a gradient of model misfit with respect to spatially-varying bottom friction field. A gradient-based quasi-Newton optimization method is applied to minimize the misfit. A key benefit of adjoint-based optimization is that the cost of does not depend on the number of unknown variables. Adjoint models are typically very laborious to implement manually. In this work, we leverage a domain specific language framework in which the discrete adjoint model can be obtained automatically. The adjoint model is both exactly compatible with the discrete forward model and computationally efficient. Optimizing spatially-variable parameter fields is typically an under-determined problem as there are more degrees of freedom compared to the amount of observational data which can lead to over-fitting. In this work, we employ Hessian-based regularization to penalize the curvature of the friction field to overcome this problem. We simulate the sea surface height (SSH) dynamics in the North Sea-Baltic Sea for a 3-month period in 2019. We show that the optimization process is robust and results in significant improvement of the model performance: SSH performance is comparable or better compared to existing state-of-the-art Baltic Sea models. The results are especially encouraging in the Danish Straits region, characterized by complex small-scale topography, highlighting the benefit of unstructured meshes.
更多查看译文
AI 理解论文
溯源树
样例
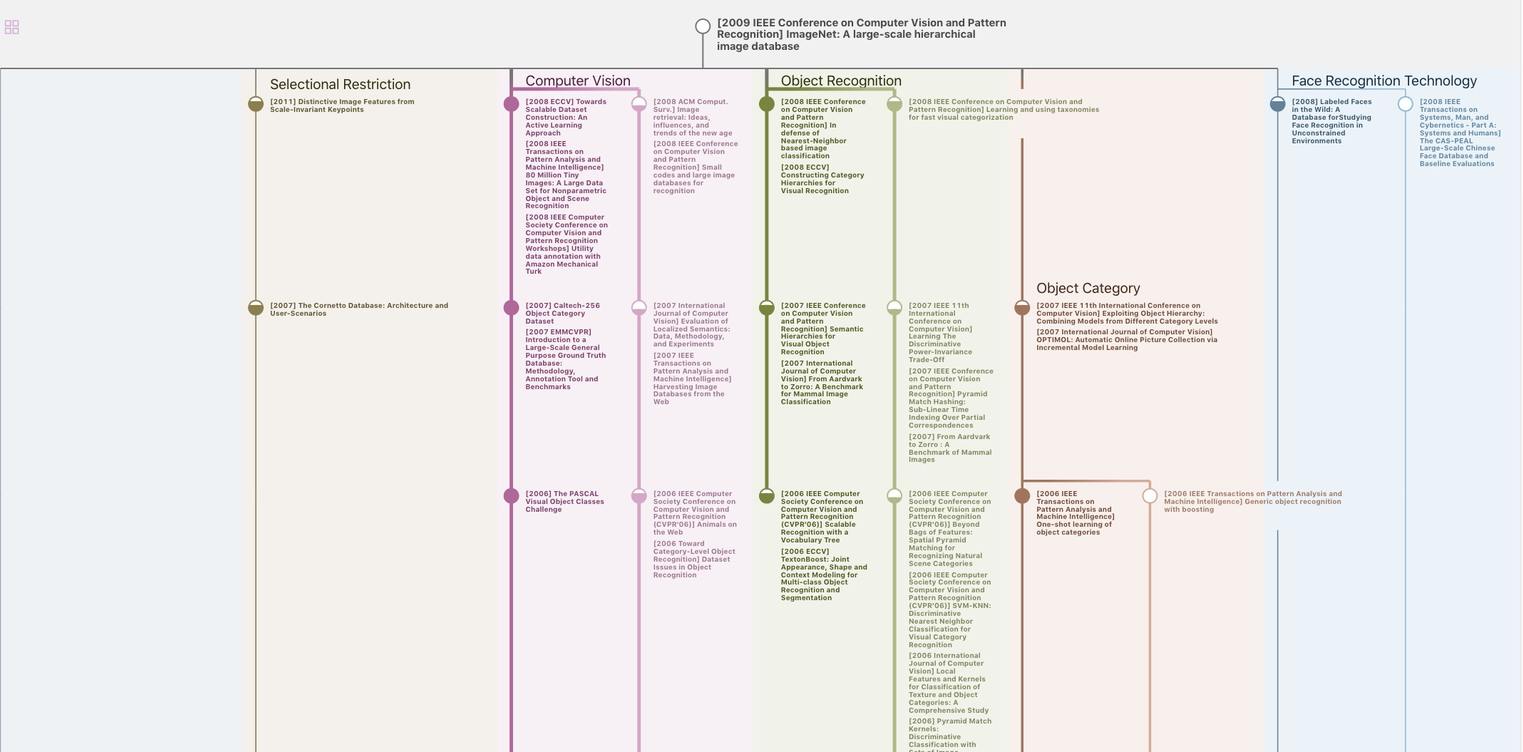
生成溯源树,研究论文发展脉络
Chat Paper
正在生成论文摘要