Use All The Labels: A Hierarchical Multi-Label Contrastive Learning Framework
IEEE Conference on Computer Vision and Pattern Recognition(2022)
摘要
Current contrastive learning frameworks focus on leveraging a single supervisory signal to learn representations, which limits the efficacy on unseen data and downstream tasks. In this paper, we present a hierarchical multi-label representation learning framework that can leverage all available labels and preserve the hierarchical relationship between classes. We introduce novel hierarchy preserving losses, which jointly apply a hierarchical penalty to the contrastive loss, and enforce the hierarchy constraint. The loss function is data driven and automatically adapts to arbitrary multi-label structures. Experiments on several datasets show that our relationship-preserving embedding performs well on a variety of tasks and outperform the base-line supervised and self-supervised approaches. Code is available at https://github.com/salesforce/hierarchicalContrastiveLearning.
更多查看译文
关键词
Representation learning, Recognition: detection,categorization,retrieval, Transfer/low-shot/long-tail learning
AI 理解论文
溯源树
样例
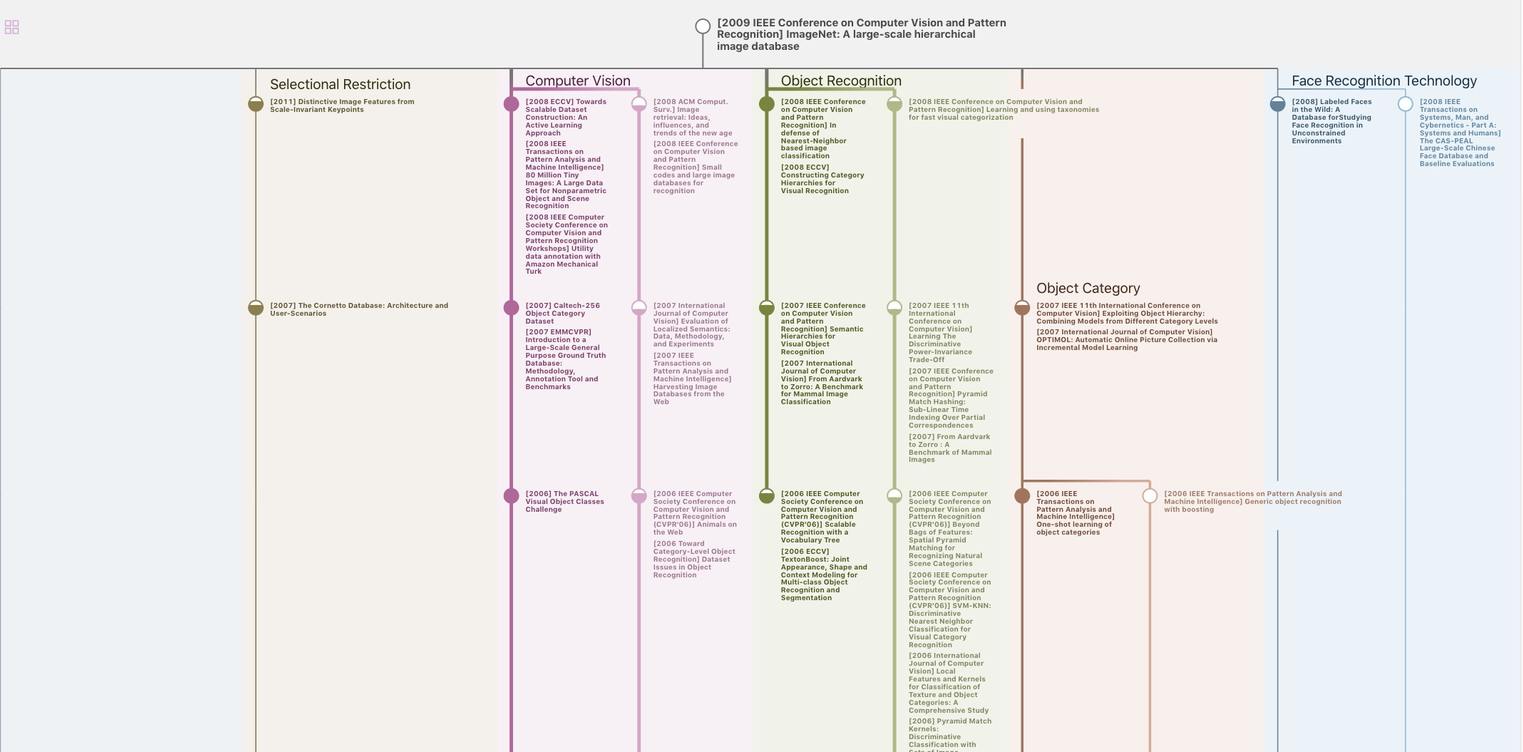
生成溯源树,研究论文发展脉络
Chat Paper
正在生成论文摘要