Data augmentation and multimodal learning for predicting drug response in patient-derived xenografts from gene expressions and histology images.
Frontiers in medicine(2023)
摘要
Patient-derived xenografts (PDXs) are an appealing platform for preclinical drug studies. A primary challenge in modeling drug response prediction (DRP) with PDXs and neural networks (NNs) is the limited number of drug response samples. We investigate multimodal neural network (MM-Net) and data augmentation for DRP in PDXs. The MM-Net learns to predict response using drug descriptors, gene expressions (GE), and histology whole-slide images (WSIs). We explore whether combining WSIs with GE improves predictions as compared with models that use GE alone. We propose two data augmentation methods which allow us training multimodal and unimodal NNs without changing architectures with a single larger dataset: 1) combine single-drug and drug-pair treatments by homogenizing drug representations, and 2) augment drug-pairs which doubles the sample size of all drug-pair samples. Unimodal NNs which use GE are compared to assess the contribution of data augmentation. The NN that uses the original and the augmented drug-pair treatments as well as single-drug treatments outperforms NNs that ignore either the augmented drug-pairs or the single-drug treatments. In assessing the multimodal learning based on the MCC metric, MM-Net outperforms all the baselines. Our results show that data augmentation and integration of histology images with GE can improve prediction performance of drug response in PDXs.
更多查看译文
关键词
data augmentation,drug response prediction,gene expression,histology whole-slide images,multimodal deep learning,patient-derived xenograft (PDX),preclinical drug studies
AI 理解论文
溯源树
样例
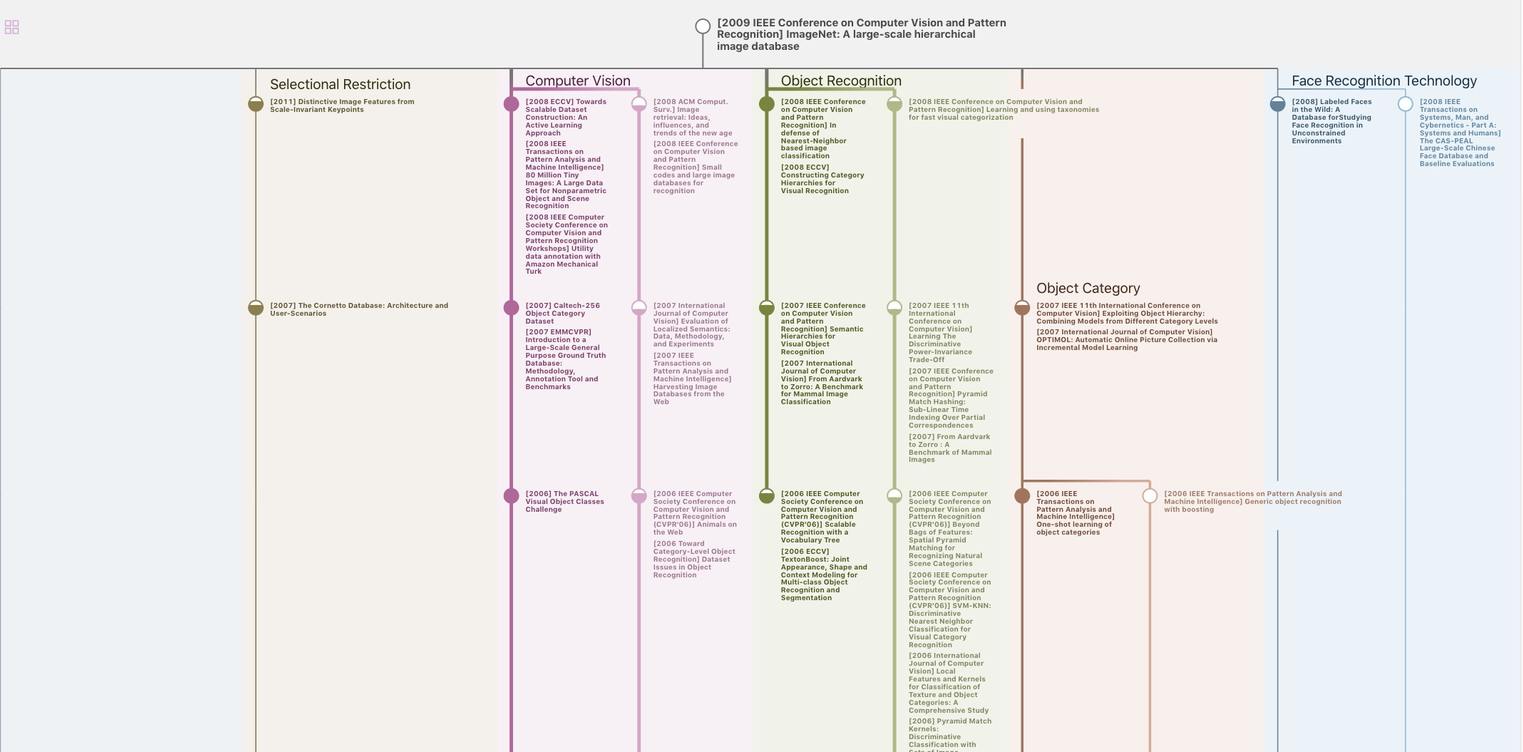
生成溯源树,研究论文发展脉络
Chat Paper
正在生成论文摘要