Hybrid Transfer in Deep Reinforcement Learning for Ads Allocation
Conference on Information and Knowledge Management(2022)
摘要
ABSTRACTAds allocation, which involves allocating ads and organic items to limited slots in feed with the purpose of maximizing platform revenue, has become a research hotspot. Notice that, platforms (e.g., e-commerce platforms, video platforms, food delivery platforms and so on) usually have multiple entrances for different categories and some entrances have few visits. Data from these entrances has low coverage, which makes it difficult for the agent to learn. To address this challenge, we propose Similarity-based Hybrid Transfer for Ads Allocation (SHTAA), which effectively transfers samples as well as knowledge from data-rich entrance to data-poor entrance. Specifically, we define an uncertainty-aware similarity for MDP to estimate the similarity of MDP for different entrances. Based on this similarity, we design a hybrid transfer method, including instance transfer and strategy transfer, to efficiently transfer samples and knowledge from one entrance to another. Both offline and online experiments on Meituan food delivery platform demonstrate that the proposed method could achieve better performance for data-poor entrance and increase the revenue for the platform.
更多查看译文
关键词
deep reinforcement learning,reinforcement learning,hybrid transfer,allocation
AI 理解论文
溯源树
样例
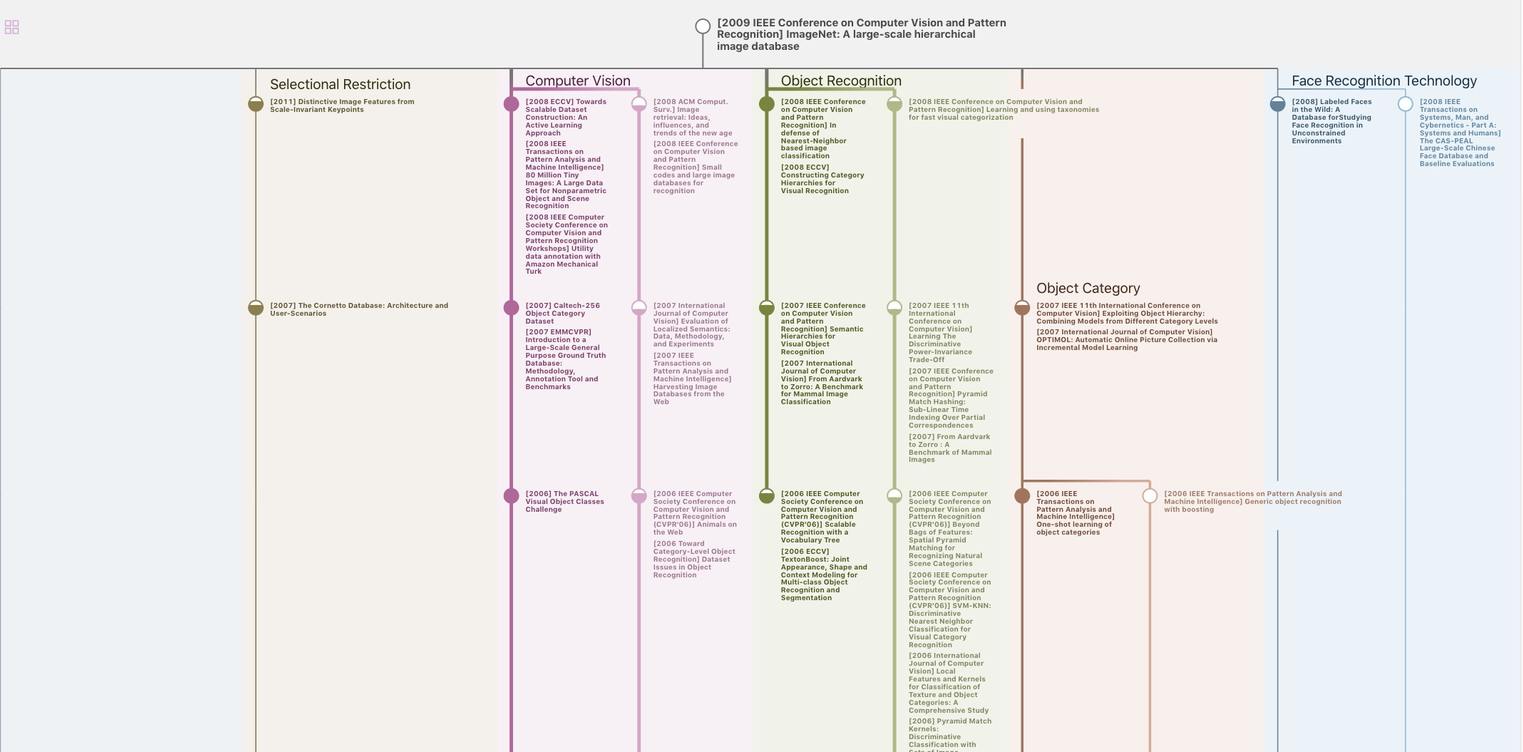
生成溯源树,研究论文发展脉络
Chat Paper
正在生成论文摘要