Federated Learning in Multi-Center Critical Care Research: A Systematic Case Study using the eICU Database
arxiv(2022)
摘要
Federated learning (FL) has been proposed as a method to train a model on different units without exchanging data. This offers great opportunities in the healthcare sector, where large datasets are available but cannot be shared to ensure patient privacy. We systematically investigate the effectiveness of FL on the publicly available eICU dataset for predicting the survival of each ICU stay. We employ Federated Averaging as the main practical algorithm for FL and show how its performance changes by altering three key hyper-parameters, taking into account that clients can significantly vary in size. We find that in many settings, a large number of local training epochs improves the performance while at the same time reducing communication costs. Furthermore, we outline in which settings it is possible to have only a low number of hospitals participating in each federated update round. When many hospitals with low patient counts are involved, the effect of overfitting can be avoided by decreasing the batchsize. This study thus contributes toward identifying suitable settings for running distributed algorithms such as FL on clinical datasets.
更多查看译文
关键词
federated learning,case study,research,multi-center
AI 理解论文
溯源树
样例
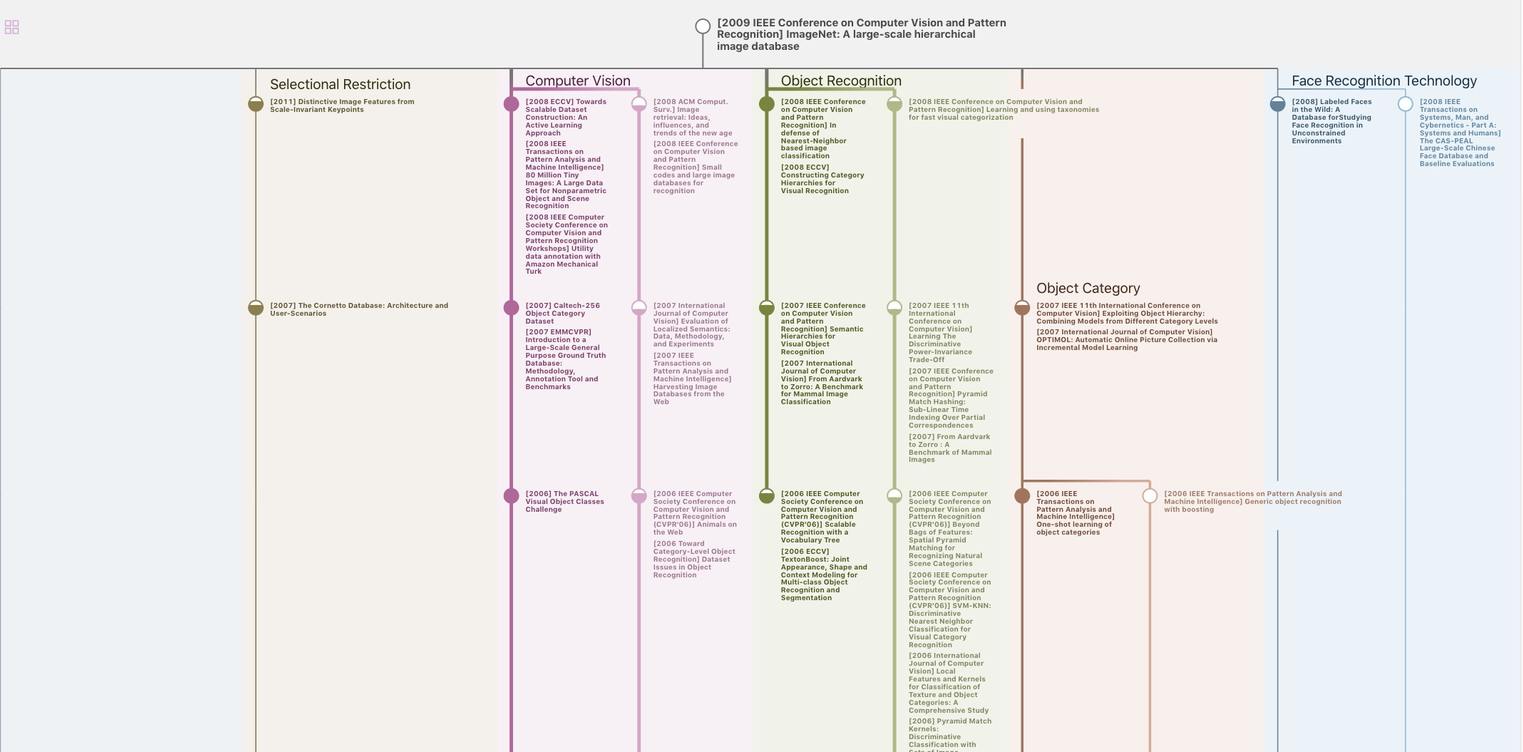
生成溯源树,研究论文发展脉络
Chat Paper
正在生成论文摘要