Generalized matrix factorization based on weighted hypergraph learning for microbe-drug association prediction
Computers in Biology and Medicine(2022)
摘要
The complex and diverse microbial communities are closely related to human health, and the research of microbial communities plays an increasingly critical role in drug development and precision medicine. Identifying potential microbe-drug associations not only benefits drug discovery and clinical therapy, but also contributes to a better understanding of the mechanisms of action of microbes. Compared with the complexity and high cost of biological experiments, computational methods can quickly and efficiently predict potential microbe-drug associations, which could be a useful complement to experimental methods. In this study, we propose a generalized matrix factorization based on weighted hypergraph learning, WHGMF, to predict potential microbial-drug associations. First, we integrate multi-omics data to compute multiple features of microbes and drugs, including functional and semantic similarity of microbes, structural similarity of drugs, and microbe-drug association information. Second, the hypergraph is constructed by using strong neighborhood information, and to improve the performance of the hypergraph, the simple volume is adopted to calculate the hyperedge weight. Finally, hypergraph regularization is introduced for the generalized matrix factorization model, and high-order structural information is used to improve the representation ability of low-dimensional features. Results from multiple experiments demonstrate that WHGMF not only accurately predicts potential microbe-drug associations, but also has considerable adaptability to class-imbalanced datasets. In addition, WHGMF is also suitable for the prediction of new drugs and new microbes. A case study further demonstrates the effectiveness of our method. The code and data in this study are freely available at https://github.com/Mayingjun20179/WHGMF.
更多查看译文
关键词
Microbe-drug association prediction,Weighted hypergraph learning,Generalized matrix decomposition,Network fused
AI 理解论文
溯源树
样例
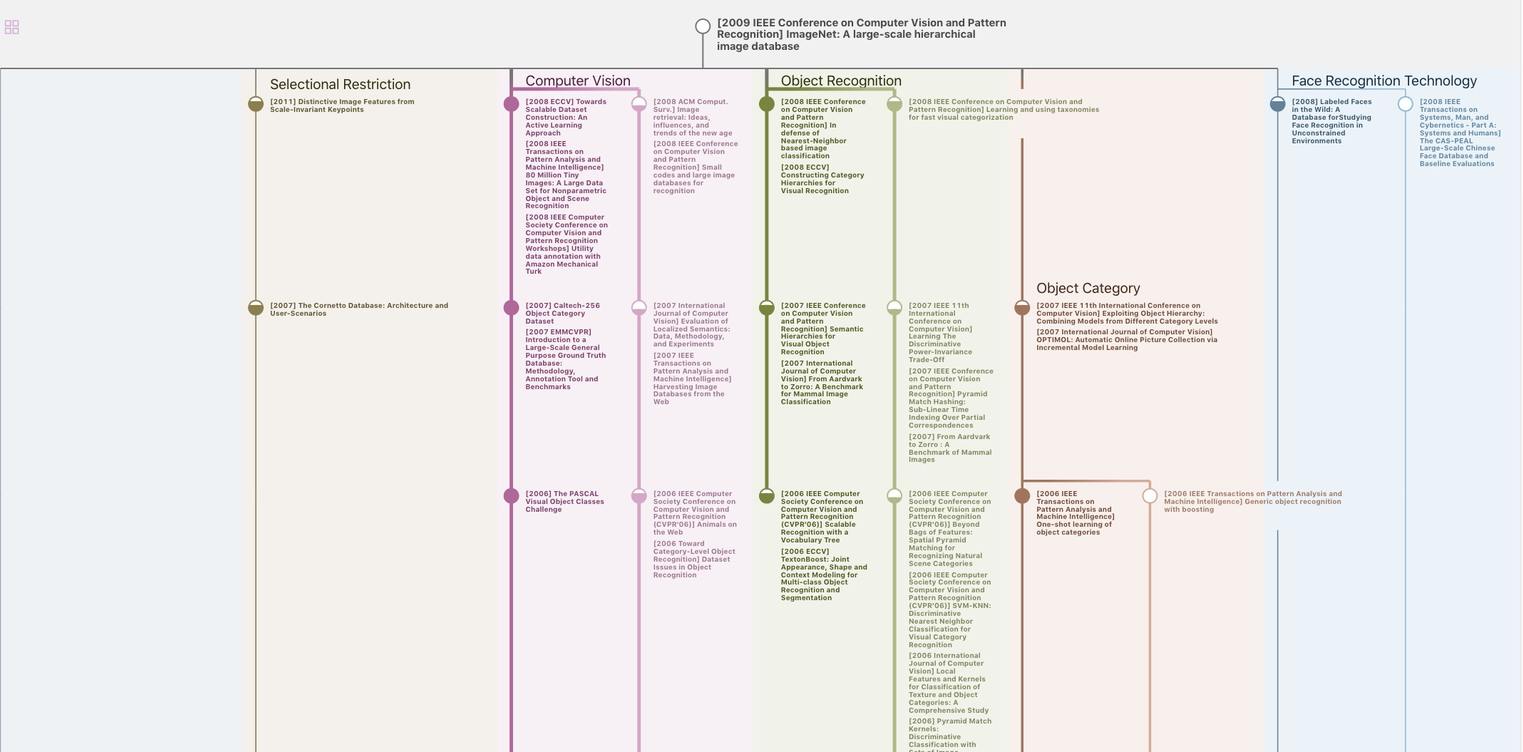
生成溯源树,研究论文发展脉络
Chat Paper
正在生成论文摘要