Unified Speech-Text Pre-training for Speech Translation and Recognition
PROCEEDINGS OF THE 60TH ANNUAL MEETING OF THE ASSOCIATION FOR COMPUTATIONAL LINGUISTICS (ACL 2022), VOL 1: (LONG PAPERS)(2022)
摘要
We describe a method to jointly pre-train speech and text in an encoder-decoder modeling framework for speech translation and recognition. The proposed method incorporates four self-supervised and supervised subtasks for cross modality learning. A self-supervised speech subtask leverages un-labelled speech data, and a (self-)supervised text to text subtask makes use of abundant text training data. Two auxiliary supervised speech tasks are included to unify speech and text modeling space. Our contribution lies in integrating linguistic information from the text corpus into the speech pre-training. Detailed analysis reveals learning interference among subtasks. Two pre-training configurations for speech translation and recognition, respectively, are presented to alleviate subtask interference. Our experiments show the proposed method can effectively fuse speech and text information into one model. It achieves between 1.7 and 2.3 BLEU improvement above the state of the art on the MUST-C speech translation dataset and comparable WERs to wav2vec 2.0 on the LIBRISPEECH speech recognition task. (1)
更多查看译文
关键词
speech-text translation,recognition,pre-training
AI 理解论文
溯源树
样例
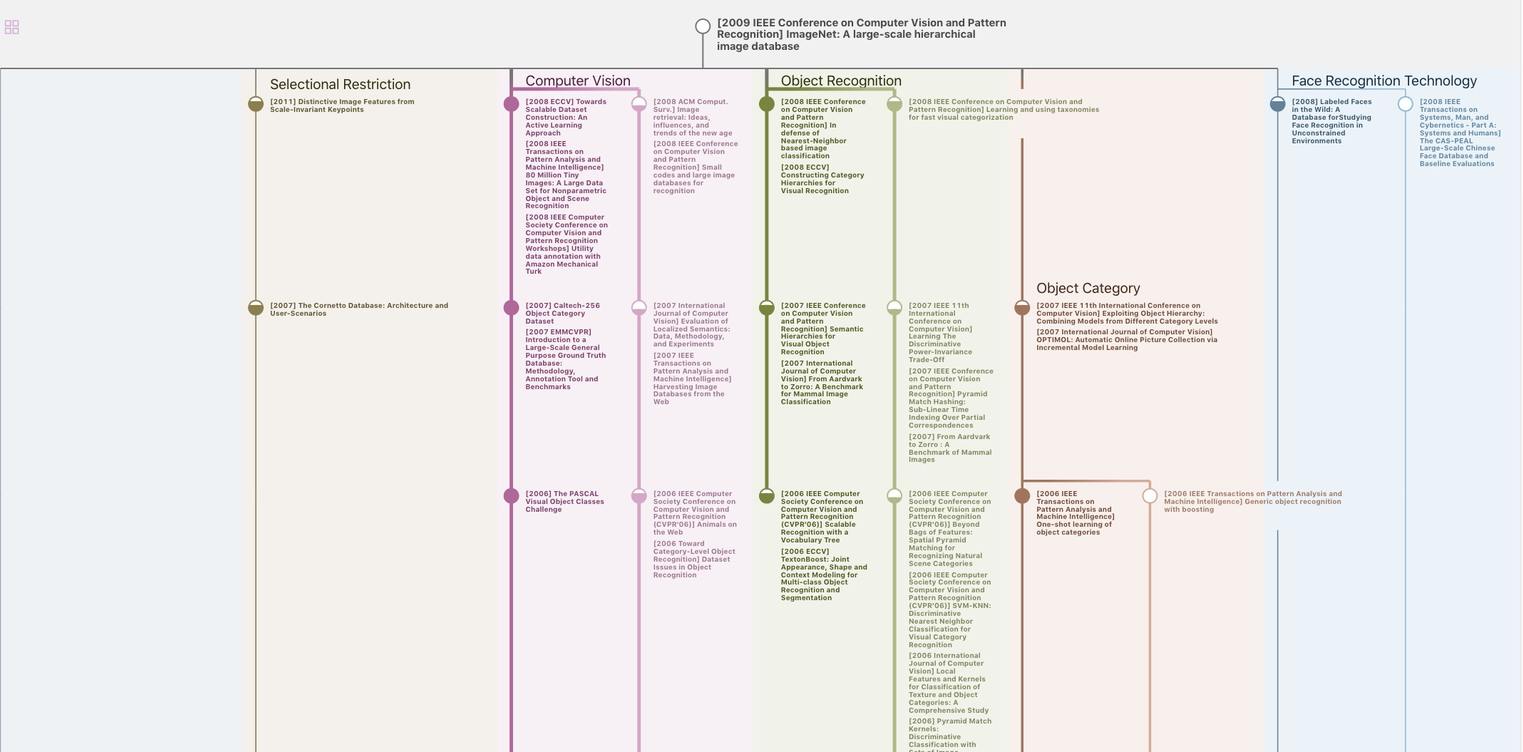
生成溯源树,研究论文发展脉络
Chat Paper
正在生成论文摘要