Learning to Induce Causal Structure
ICLR 2023(2022)
摘要
The fundamental challenge in causal induction is to infer the underlying graph structure given observational and/or interventional data. Most existing causal induction algorithms operate by generating candidate graphs and evaluating them using either score-based methods (including continuous optimization) or independence tests. In our work, we instead treat the inference process as a black box and design a neural network architecture that learns the mapping from both observational and interventional data to graph structures via supervised training on synthetic graphs. The learned model generalizes to new synthetic graphs, is robust to train-test distribution shifts, and achieves state-of-the-art performance on naturalistic graphs for low sample complexity.
更多查看译文
关键词
causality,deep learning
AI 理解论文
溯源树
样例
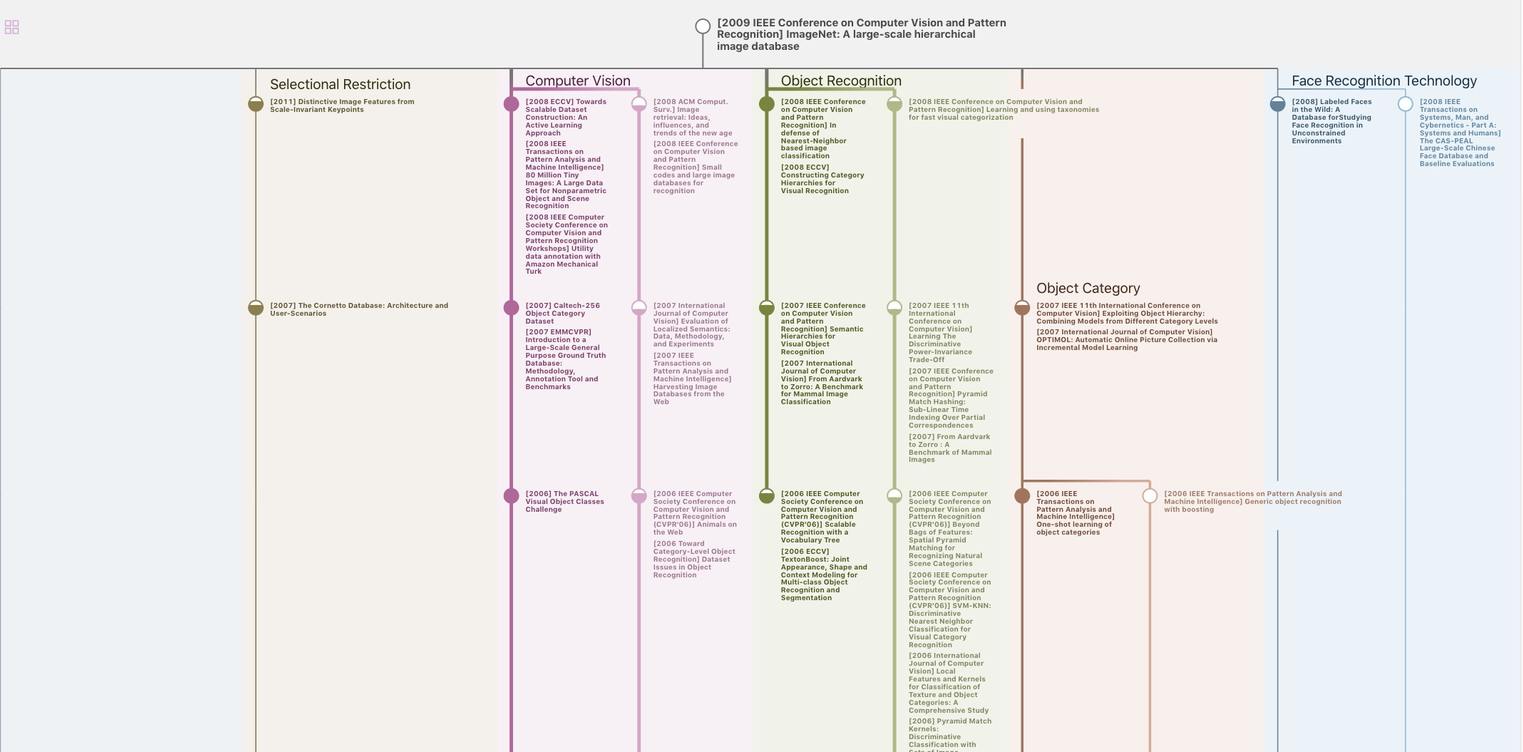
生成溯源树,研究论文发展脉络
Chat Paper
正在生成论文摘要