Marginal Contrastive Correspondence for Guided Image Generation
IEEE Conference on Computer Vision and Pattern Recognition(2022)
摘要
Exemplar-based image translation establishes dense correspondences between a conditional input and an exemplar (from two different domains) for leveraging detailed exemplar styles to achieve realistic image translation. Existing work builds the cross-domain correspondences implicitly by minimizing feature- wise distances across the two domains. Without explicit exploitation of domain-invariant features, this approach may not reduce the domain gap effectively which often leads to sub-optimal correspon-dences and image translation. We design a Marginal Contrastive Learning Network (MCL-Net) that explores contrastive learning to learn domain-invariant features for realistic exemplar-based image translation. Specifically, we design an innovative marginal contrastive loss that guides to establish dense correspondences explicitly. Nevertheless, building correspondence with domain-invariant semantics alone may impair the texture patterns and lead to degraded texture generation. We thus design a Self-Correlation Map (SCM) that incorporates scene structures as auxiliary information which improves the built correspondences substantially. Quantitative and qualitative experiments on multifarious image translation tasks show that the proposed method outperforms the state-of-the-art consistently.
更多查看译文
关键词
Image and video synthesis and generation, Computational photography
AI 理解论文
溯源树
样例
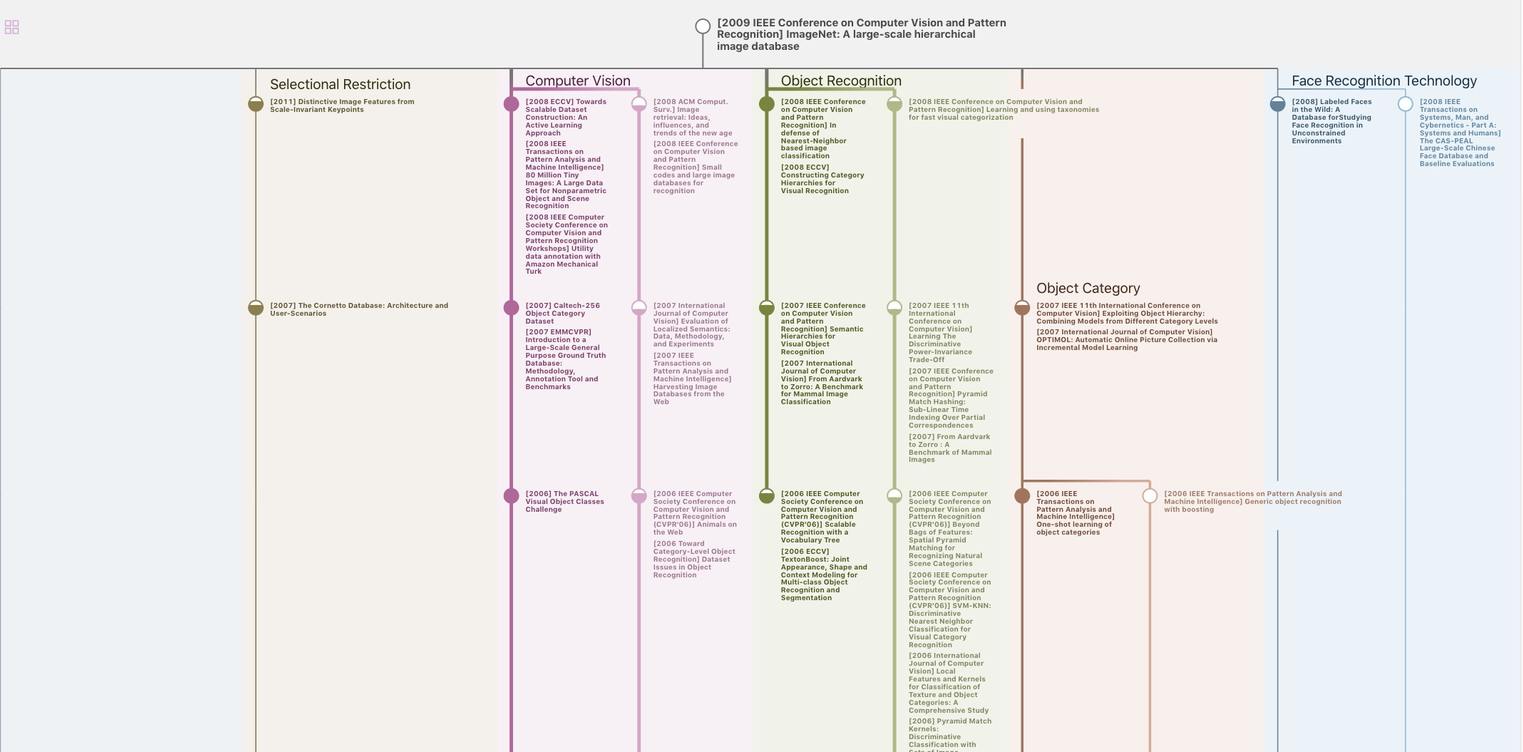
生成溯源树,研究论文发展脉络
Chat Paper
正在生成论文摘要