DIP: Deep Inverse Patchmatch for High-Resolution Optical Flow
IEEE Conference on Computer Vision and Pattern Recognition(2022)
摘要
Recently, the dense correlation volume method achieves state-of-the-art performance in optical flow. However, the correlation volume computation requires a lot of memory, which makes prediction difficult on high-resolution images. In this paper, we propose a novel Patchmatch-based framework to work on high-resolution optical flow estimation. Specifically, we introduce the first end-to-end Patchmatch based deep learning optical flow. It can get high-precision results with lower memory benefiting from propagation and local search of Patchmatch. Furthermore, a new inverse propagation is proposed to decouple the complex operations of propagation, which can significantly reduce calculations in multiple iterations. At the time of submission, our method ranks 1st on all the metrics on the popular KITTI2015 [28] benchmark, and ranks 2 nd on EPE on the Sintel [7] clean benchmark among published optical flow methods. Experiment shows our method has a strong cross-dataset generalization ability that the F1-all achieves 13.73%, reducing 21% from the best published result 17.4% on KITTI2015. What's more, our method shows a good details preserving result on the high-resolution dataset DAVIS [1] and consumes 2× less memory than RAFT [36]. Code will be available at github.com/zihuarheng/DIP
更多查看译文
关键词
Motion and tracking
AI 理解论文
溯源树
样例
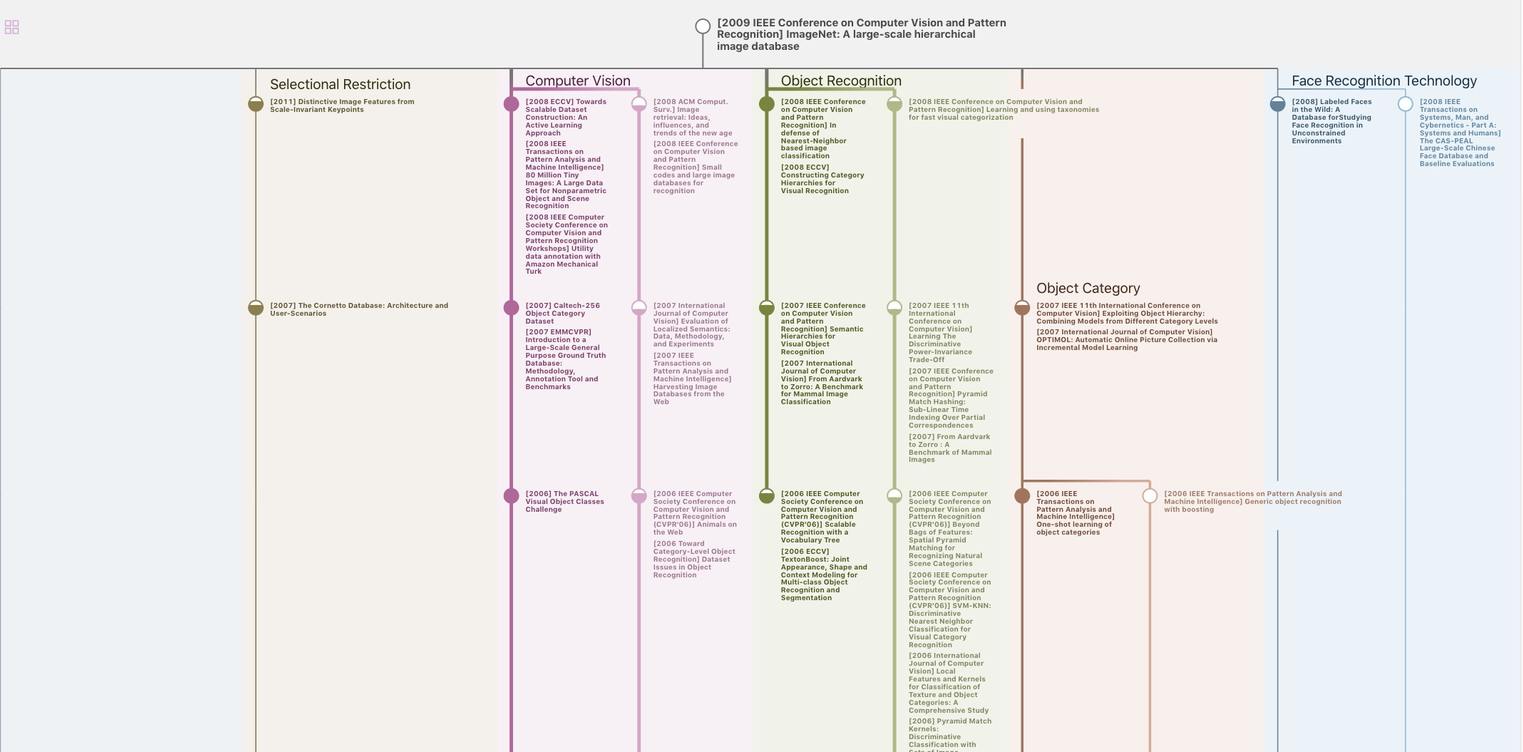
生成溯源树,研究论文发展脉络
Chat Paper
正在生成论文摘要