Multi-metric learning by a pair of twin-metric learning framework
Applied Intelligence(2022)
摘要
Multi-metric learning is important for improving classification performance since learning a single metric is usually insufficient for complex data. The existing multi-metric learning methods are based on the triplet constraints, and thus are with high computing complexity. In this work, we propose an efficient multi-metric learning framework by a pair of two-metric learning schemes (called TMML) to jointly train two local metrics and a global metric, where the distances between samples are automatically adjusted to maximize classification margin. Instead of the triplet constraints, the proposed TMML is based on the pair constraints to reduce the computational burden. Moreover, a global regularization is introduced to improve generalization and control overfitting. The proposed TMML improves the limitation of a single metric, where a pair of local metrics are interrelated to conduct adaptation for the local characteristics, while global metrics are to depict the common properties from all the data. Furthermore, we develop an alternating direction iterative algorithm to optimize the proposed TMML. The convergence of the algorithm is analyzed theoretically. Numerical experiments are carried out on different scale datasets. Under different evaluation criteria, experiments show that the proposed TMML is superior to the single metric learning methods, and achieves better performance than other state-of-the-art multi-metric learning methods in most cases.
更多查看译文
关键词
Multi-metric learning, Two-metric learning, Pair constraints, Classification, Iteration algorithms
AI 理解论文
溯源树
样例
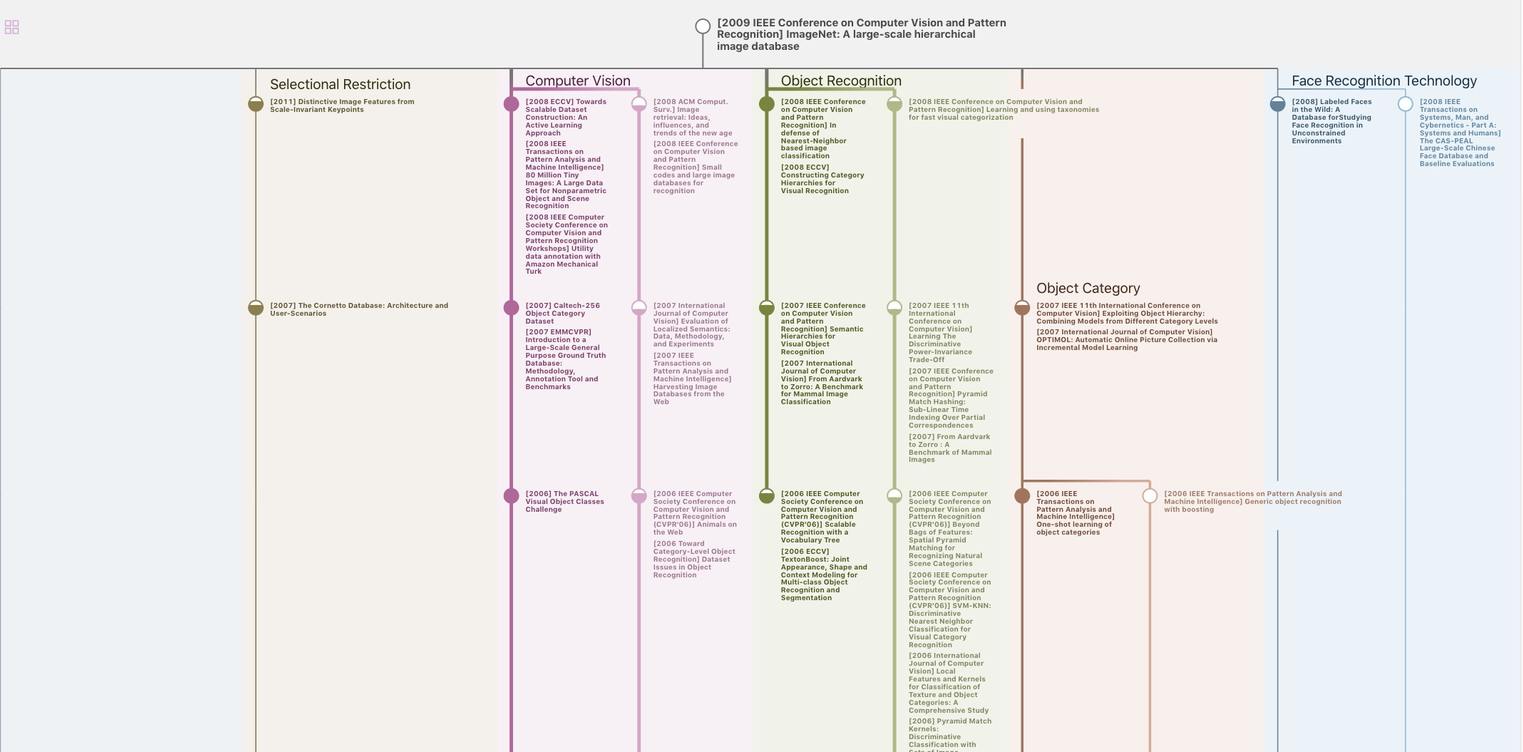
生成溯源树,研究论文发展脉络
Chat Paper
正在生成论文摘要