Zero-Shot Meta-Learning for Small-Scale Data From Human Subjects
arxiv(2023)
摘要
While developments in machine learning led to impressive performance gains on big data, many human subjects data are, in actuality, small and sparsely labeled. Existing methods applied to such data often do not easily generalize to out-of-sample subjects. Instead, models must make predictions on test data that may be drawn from a different distribution, a problem known as zero-shot learning. To address this challenge, we develop an end-to-end framework using a meta-learning approach, which enables the model to rapidly adapt to a new prediction task with limited training data for out-of-sample test data. We use three real-world small-scale human subjects datasets (two randomized control studies and one observational study), for which we predict treatment outcomes for held-out treatment groups. Our model learns the latent treatment effects of each intervention and, by design, can naturally handle multitask predictions. We show that our model performs the best holistically for each held-out group and especially when the test group is distinctly different from the training group. Our model has implications for improved generalization of small-size human studies to the wider population.
更多查看译文
关键词
zero-shot learning,meta-learning,small-scale data,human subjects
AI 理解论文
溯源树
样例
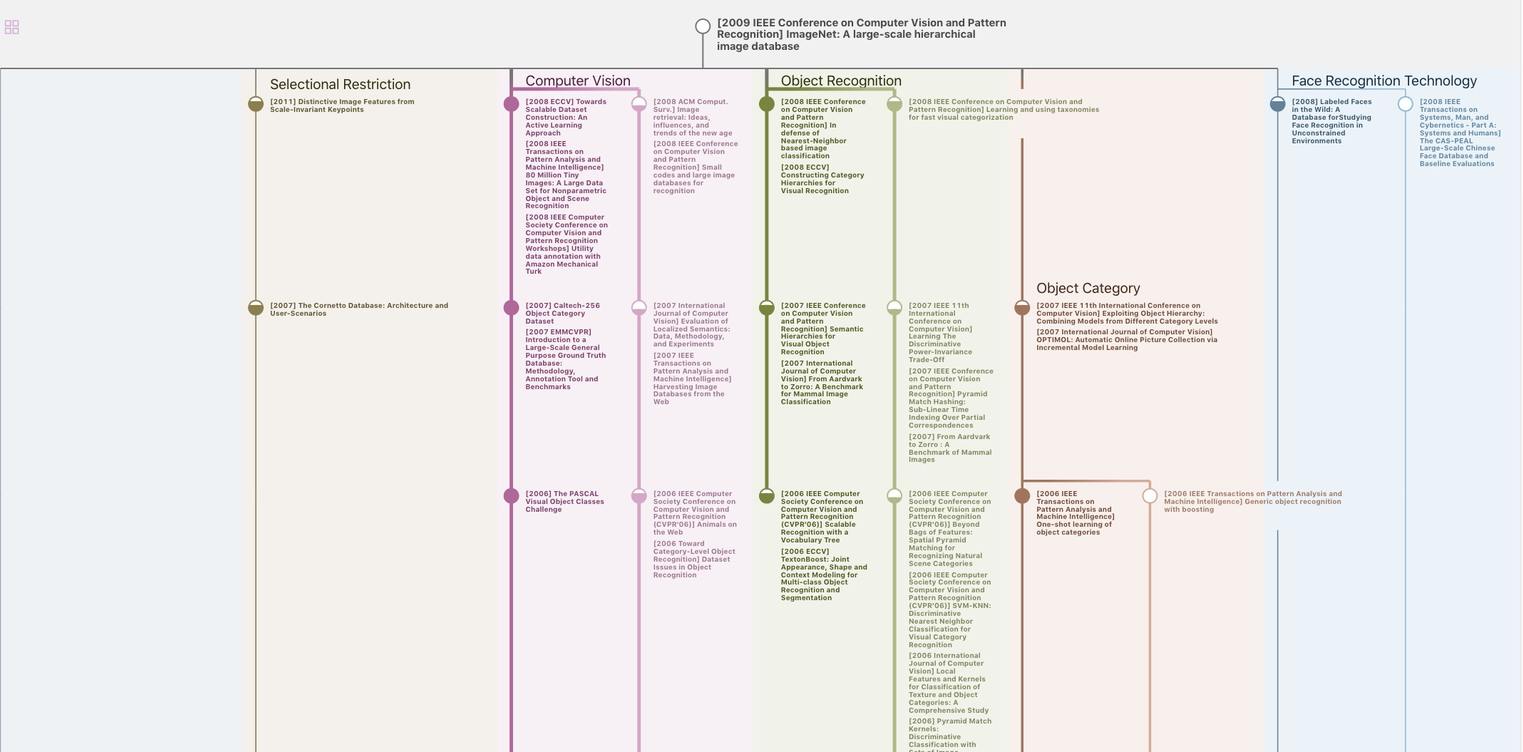
生成溯源树,研究论文发展脉络
Chat Paper
正在生成论文摘要