Multi-scale Speaker Diarization with Dynamic Scale Weighting
Conference of the International Speech Communication Association (INTERSPEECH)(2022)
摘要
Speaker diarization systems are challenged by a trade-off between the temporal resolution and the fidelity of the speaker representation. By obtaining a superior temporal resolution with an enhanced accuracy, a multi-scale approach is a way to cope with such a trade-off. In this paper, we propose a more advanced multi-scale diarization system based on a multi-scale diarization decoder. There are two main contributions in this study that significantly improve the diarization performance. First, we use multi-scale clustering as an initialization to estimate the number of speakers and obtain the average speaker representation vector for each speaker and each scale. Next, we propose the use of 1-D convolutional neural networks that dynamically determine the importance of each scale at each time step. To handle a variable number of speakers and overlapping speech, the proposed system can estimate the number of existing speakers. Our proposed system achieves a state-of-art performance on the CALLHOME and AMI MixHeadset datasets, with 3.92% and 1.05% diarization error rates, respectively.
更多查看译文
关键词
speaker diarization, multi-scale
AI 理解论文
溯源树
样例
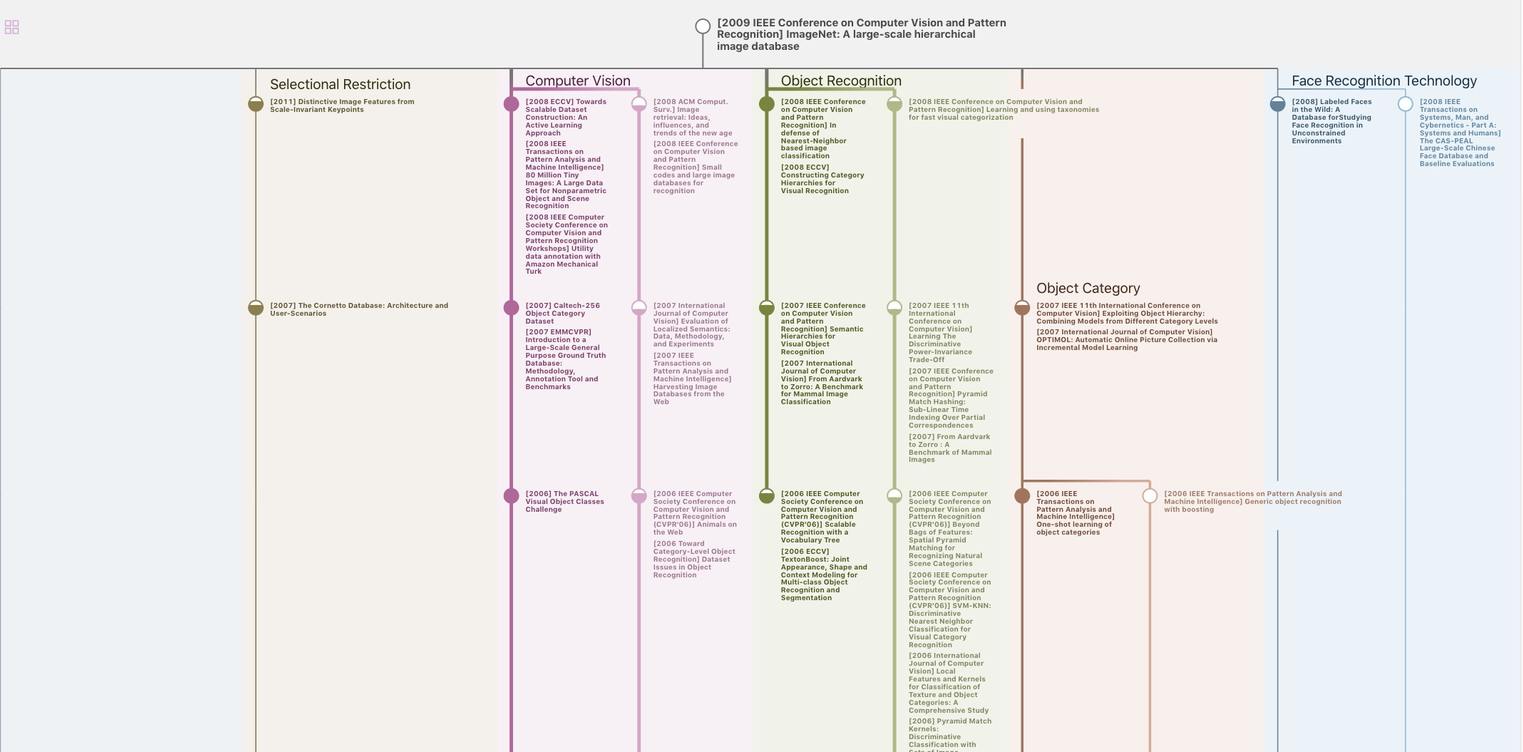
生成溯源树,研究论文发展脉络
Chat Paper
正在生成论文摘要