Bayesian deep learning-based H-1-MRS of the brain: Metabolite quantification with uncertainty estimation using Monte Carlo dropout
Magnetic resonance in medicine(2022)
摘要
Purpose To develop a Bayesian convolutional neural network (BCNN) with Monte Carlo dropout sampling for metabolite quantification with simultaneous uncertainty estimation in deep learning-based proton MRS of the brain. Methods Human brain spectra were simulated using basis spectra for 17 metabolites and macromolecules (N = 100 000) at 3.0 Tesla. In addition, actual in vivo spectra (N = 5) were modified by adjusting SNR and linewidth with increasing severity of spectral degradation (N = 50). A BCNN was trained on the simulated spectra to generate a noise-free, line-narrowed, macromolecule signal-removed, metabolite-only spectrum from a typical human brain spectrum. At inference, each input spectrum was Monte Carlo dropout sampled (50 times), and the resulting mean spectrum and variance spectrum were used for metabolite quantification and uncertainty estimation, respectively. Results Using the simulated spectra, the mean absolute percent errors of the BCNN-predicted metabolite content were < 10% for Cr, Glu, Gln, mI, NAA, and Tau (< 5% for Glu, NAA, and mI). For all metabolites, the correlations (r's) between the ground-truth error and BCNN-predicted uncertainty ranged 0.72-0.94 (0.83 +/- 0.06; p < 0.001). Using the modified in vivo spectra, the extent of variation in the estimated metabolite content against the increasing severity of spectral degradation tended to be smaller with BCNN than with linear combination of model spectra (LCModel). Overall, the variation in metabolite content tended to be more highly correlated with the uncertainty from BCNN than with the Cramer-Rao lower-bounds from LCModel (0.938 +/- 0.019 vs. 0.881 +/- 0.057 [p = 0.115]). Conclusion The BCNN with Monte Carlo dropout sampling may be used in deep learning-based MRS for the estimation of uncertainty in the machine-predicted metabolite content, which is important in the clinical application of deep learning-based MRS.
更多查看译文
关键词
Bayesian neural network, brain metabolites, convolutional neural network, Monte Carlo dropout, proton MRS, uncertainty
AI 理解论文
溯源树
样例
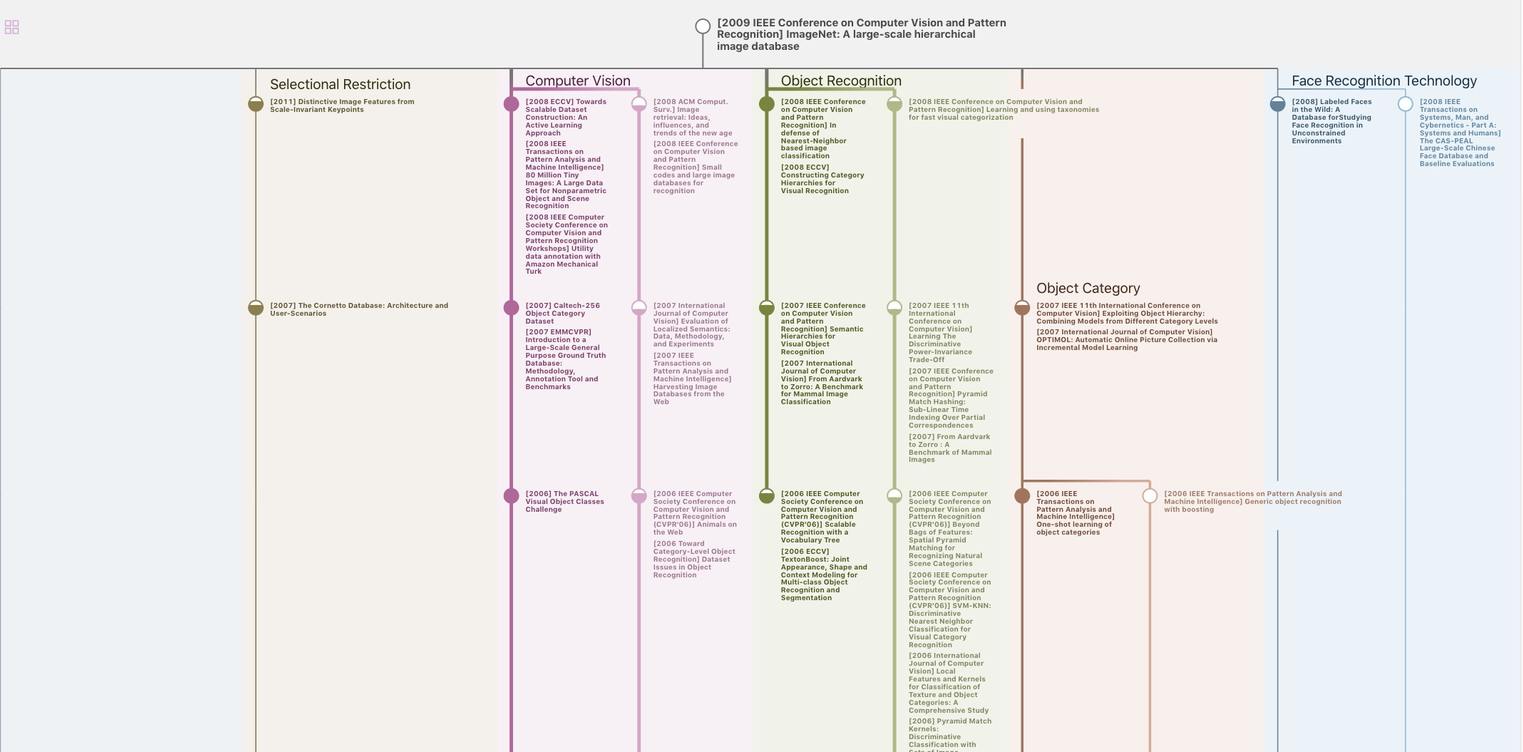
生成溯源树,研究论文发展脉络
Chat Paper
正在生成论文摘要