Learning a Structured Latent Space for Unsupervised Point Cloud Completion
IEEE Conference on Computer Vision and Pattern Recognition(2022)
摘要
Unsupervised point cloud completion aims at estimating the corresponding complete point cloud of a partial point cloud in an unpaired manner. It is a crucial but challenging problem since there is no paired partial-complete supervision that can be exploited directly. In this work, we pro-pose a novel framework, which learns a unified and structured latent space that encoding both partial and complete point clouds. Specifically, we map a series of related par-tial point clouds into multiple complete shape and occlusion code pairs and fuse the codes to obtain their repre-sentations in the unified latent space. To enforce the learning of such a structured latent space, the proposed method adopts a series of constraints including structured ranking regularization, latent code swapping constraint, and distribution supervision on the related partial point clouds. By establishing such a unified and structured latent space, better partial-complete geometry consistency and shape completion accuracy can be achieved. Extensive experi-ments show that our proposed method consistently outper-forms state-of-the-art unsupervised methods on both syn-thetic ShapeNet and real-world KITTI, ScanNet, and Mat- terport3D datasets.
更多查看译文
关键词
3D from multi-view and sensors, Self-& semi-& meta- & unsupervised learning
AI 理解论文
溯源树
样例
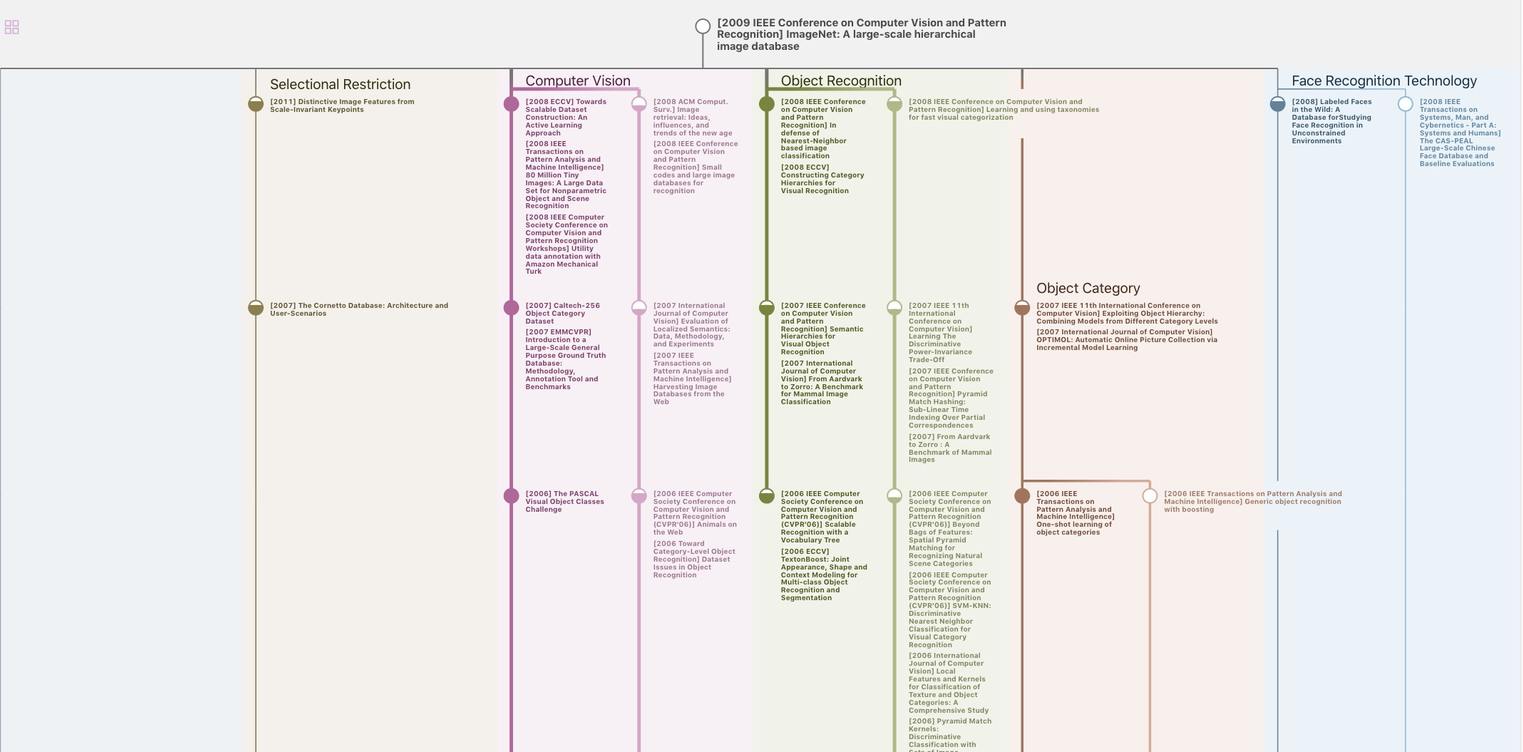
生成溯源树,研究论文发展脉络
Chat Paper
正在生成论文摘要