FedGradNorm: Personalized Federated Gradient-Normalized Multi-Task Learning.
International Workshop on Signal Processing Advances in Wireless Communications (SPAWC)(2022)
摘要
Multi-task learning (MTL) is a novel framework to learn several tasks simultaneously with a single shared network where each task has its distinct personalized header network for fine-tuning. MTL can be implemented in federated learning settings as well, in which tasks are distributed across clients. In federated settings, the statistical heterogeneity due to different task complexities and data heterogeneity due to non-iid nature of local datasets can both degrade the learning performance of the system. In addition, tasks can negatively affect each other's learning performance due to negative transference effects. To cope with these challenges, we propose FedGradNorm which uses a dynamic-weighting method to normalize gradient norms in order to balance learning speeds among different tasks. FedGradNorm improves the overall learning performance in a personalized federated learning setting. We provide convergence analysis for FedGradNorm by showing that it has an exponential convergence rate. We also conduct experiments on multi-task facial landmark (MTFL) and wireless communication system dataset (RadComDynamic). The experimental results show that our framework can achieve faster training performance compared to equal-weighting strategy. In addition to improving training speed, FedGradNorm also compensates for the imbalanced datasets among clients.
更多查看译文
关键词
learning,gradient-normalized,multi-task
AI 理解论文
溯源树
样例
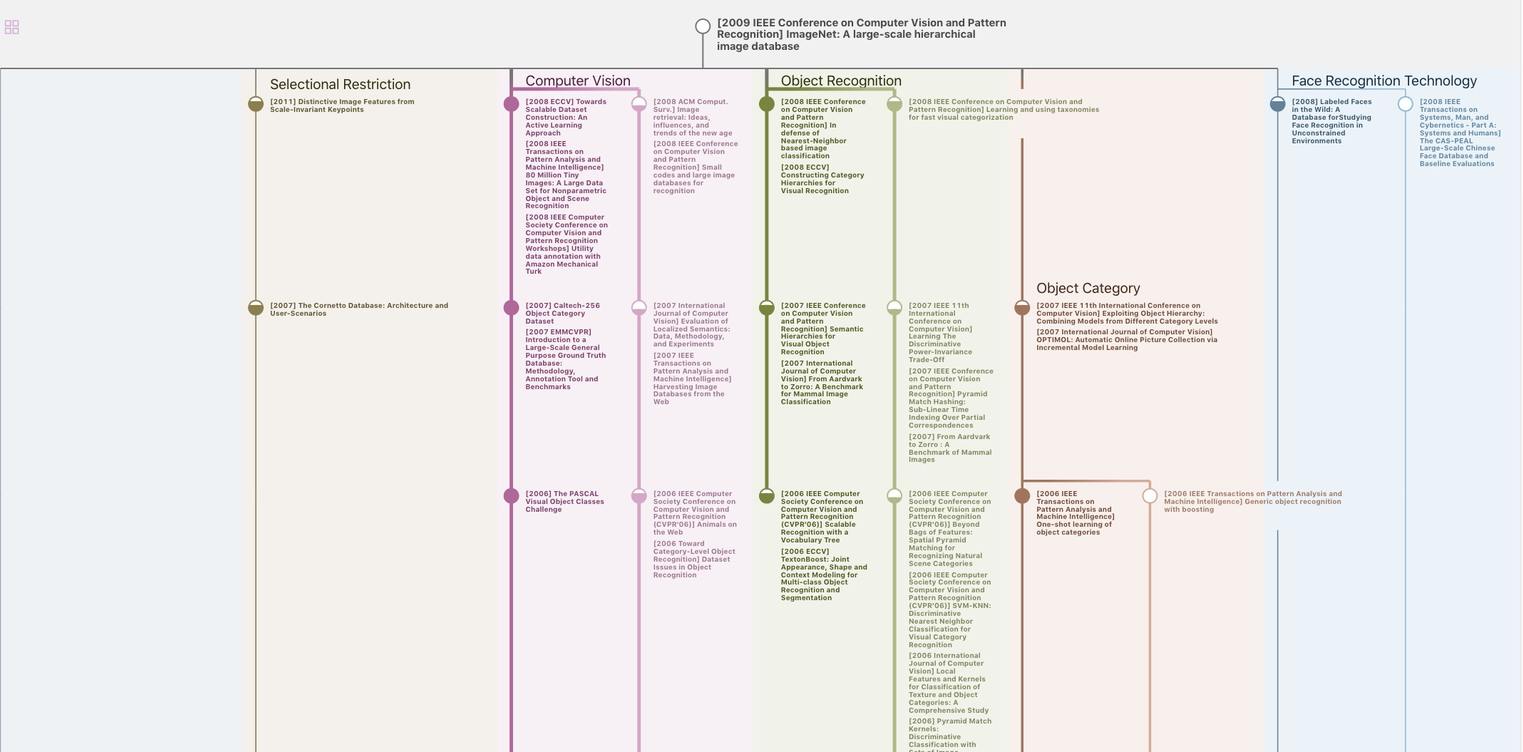
生成溯源树,研究论文发展脉络
Chat Paper
正在生成论文摘要