Unsupervised Domain Adaptation through Shape Modeling for Medical Image Segmentation
user-61442502e55422cecdaf6898(2021)
摘要
Shape information is a strong and valuable prior in segmenting organs in medical images. However, most current deep learning based segmentation algorithms have not taken shape information into consideration, which can lead to bias towards texture. We aim at modeling shape explicitly and using it to help medical image segmentation. Previous methods proposed Variational Autoencoder (VAE) based models to learn the distribution of shape for a particular organ and used it to automatically evaluate the quality of a segmentation prediction by fitting it into the learned shape distribution. Based on which we aim at incorporating VAE into current segmentation pipelines. Specifically, we propose a new unsupervised domain adaptation pipeline based on a pseudo loss and a VAE reconstruction loss under a teacher-student learning paradigm. Both losses are optimized simultaneously and, in return, boost the segmentation task performance. Extensive experiments on three public Pancreas segmentation datasets as well as two in-house Pancreas segmentation datasets show consistent improvements with at least 2.8 points gain in the Dice score, demonstrating the effectiveness of our method in challenging unsupervised domain adaptation scenarios for medical image segmentation. We hope this work will advance shape analysis and geometric learning in medical imaging.
更多查看译文
关键词
shape modeling,segmentation,medical image
AI 理解论文
溯源树
样例
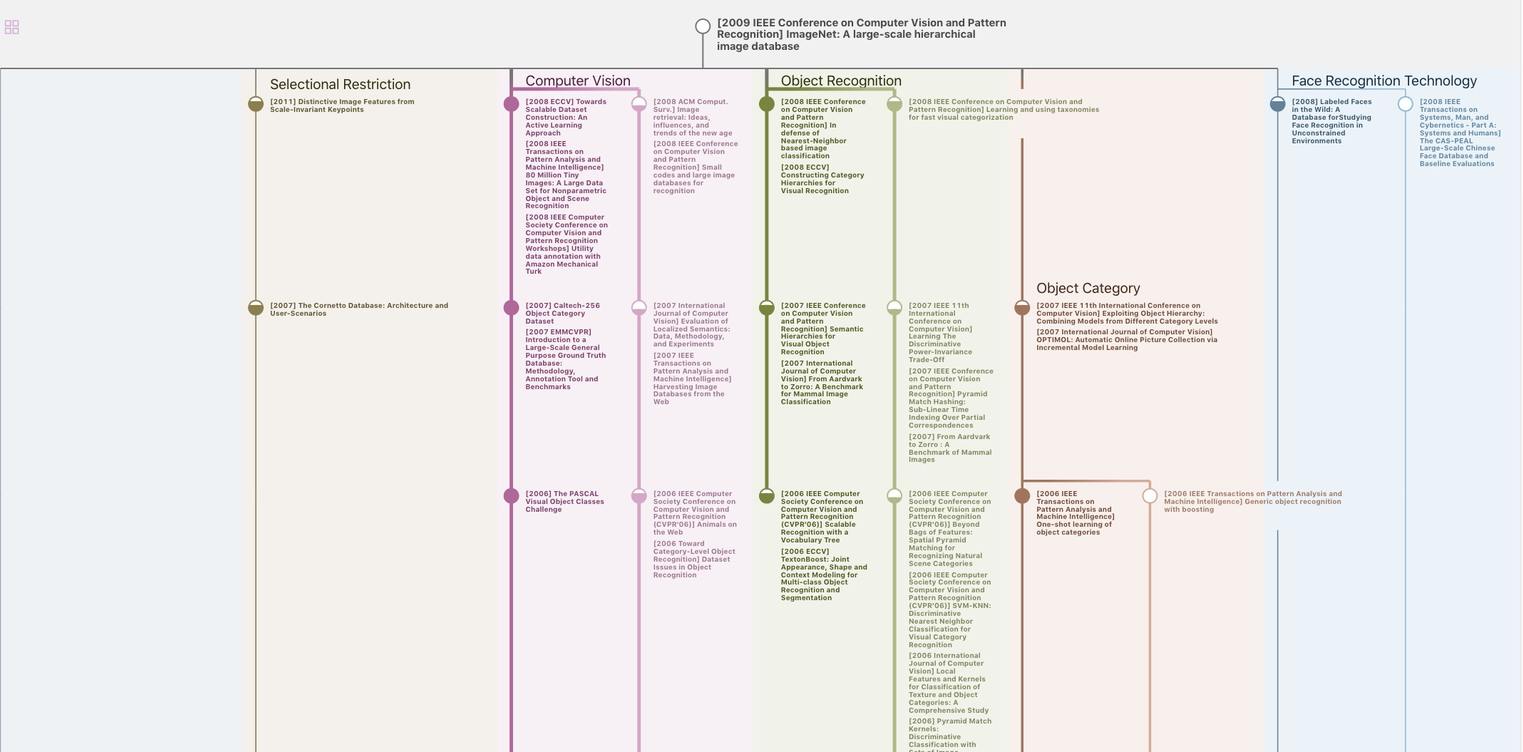
生成溯源树,研究论文发展脉络
Chat Paper
正在生成论文摘要