System Performance as a Function of Calibration Methods, Sample Size and Sampling Variability in Likelihood Ratio-Based Forensic Voice Comparison.
Interspeech(2021)
摘要
In data-driven forensic voice comparison, sample size is an issue which can have substantial effects on system output. Numerous calibration methods have been developed and some have been proposed as solutions to sample size issues. In this paper, we test four calibration methods (i.e. logistic regression, regularised logistic regression, Bayesian model, ELUB) under different conditions of sampling variability and sample size. Training and test scores were simulated from skewed distributions derived from real experiments, increasing sample sizes from 20 to 100 speakers for both the training and test sets. For each sample size, the experiments were replicated 100 times to test the susceptibility of different calibration methods to sampling variability. The C-llr mean and range across replications were used for evaluation. The Bayesian model and regularized logistic regression produced the most stable C-llr values when the sample size is small (i.e. 20 speakers), although mean C-llr is consistently lowest using logistic regression. The ELUB calibration method generally is the least preferred as it is the most sensitive to sample size and sampling variability (mean = 0.66, range = 0.21-0.59).
更多查看译文
关键词
likelihood ratio,forensic voice comparison,calibration,sample size,sampling variability
AI 理解论文
溯源树
样例
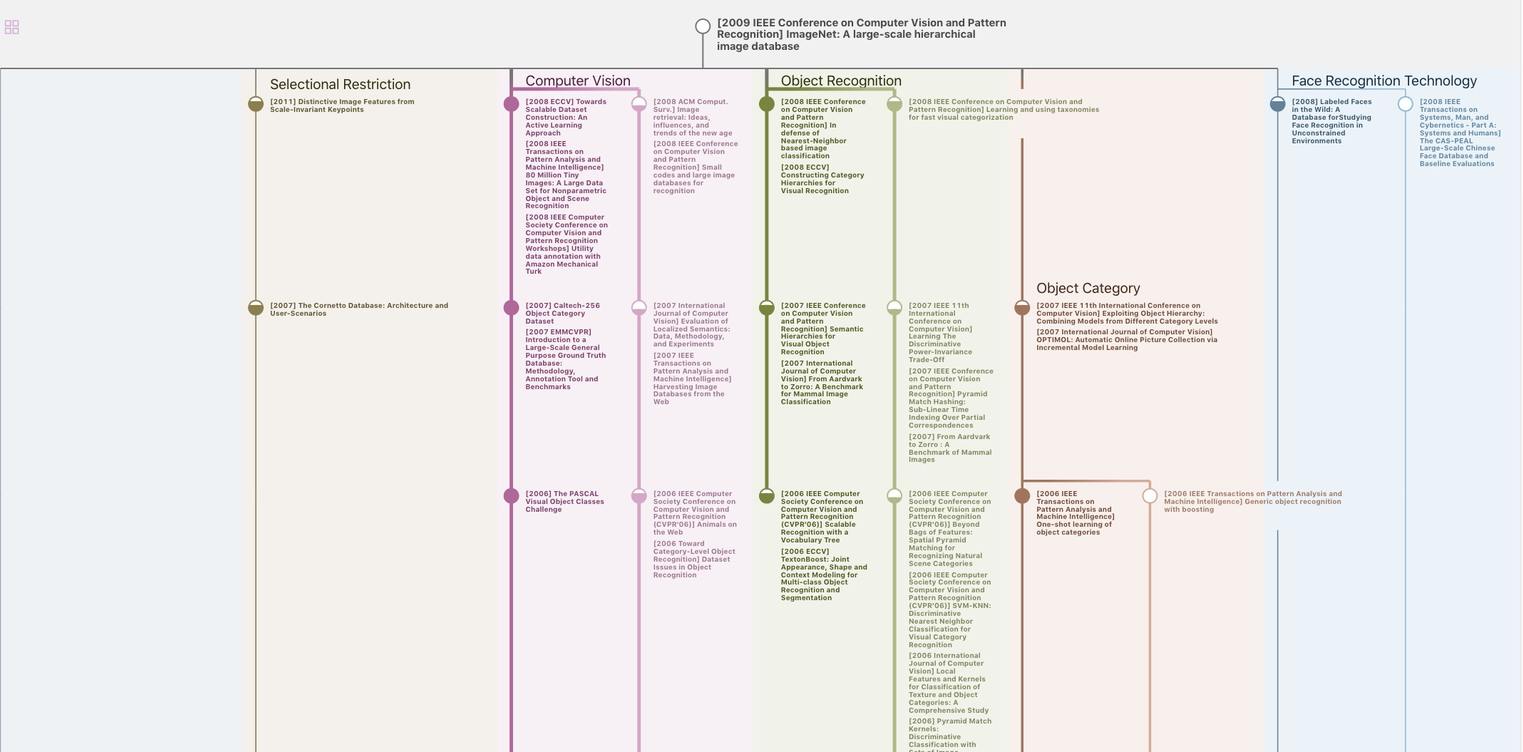
生成溯源树,研究论文发展脉络
Chat Paper
正在生成论文摘要