Graph-based relational reasoning in a latent space for skeleton-based action recognition
Journal of Visual Communication and Image Representation(2022)
摘要
Motivated by the powerful capability of deep neural networks in feature learning, a new graph-based neural network is proposed to learn local and global relational information on skeleton sequences represented as spatio-temporal graphs (STGs). The pipeline of our network architecture consists of three main stages. As the first stage, spatial–temporal sub-graphs (sub-STGs) are projected into a latent space in which every point is represented as a linear subspace. The second stage is based on message passing to acquire the localized correlated features of the nodes in the latent space. The third stage relies on graph convolutional networks (GCNs) to reason the long-range spatio-temporal dependencies through a graph representation of the latent space. Finally, the average pooling layer and the softmax classifier are then employed to predict the action categories based on the extracted local and global correlations. We validate our model in terms of action recognition using three challenging datasets: the NTU RGB+D, Kinetics Motion, and SBU Kinect Interaction datasets. The experimental results demonstrate the effectiveness of our approach and show that our proposed model outperforms the state-of-the-art methods.
更多查看译文
关键词
Deep learning,Graph neural networks,Graph convolutional network,Message passing,Spatio-temporal graph,Grassmannian geometry
AI 理解论文
溯源树
样例
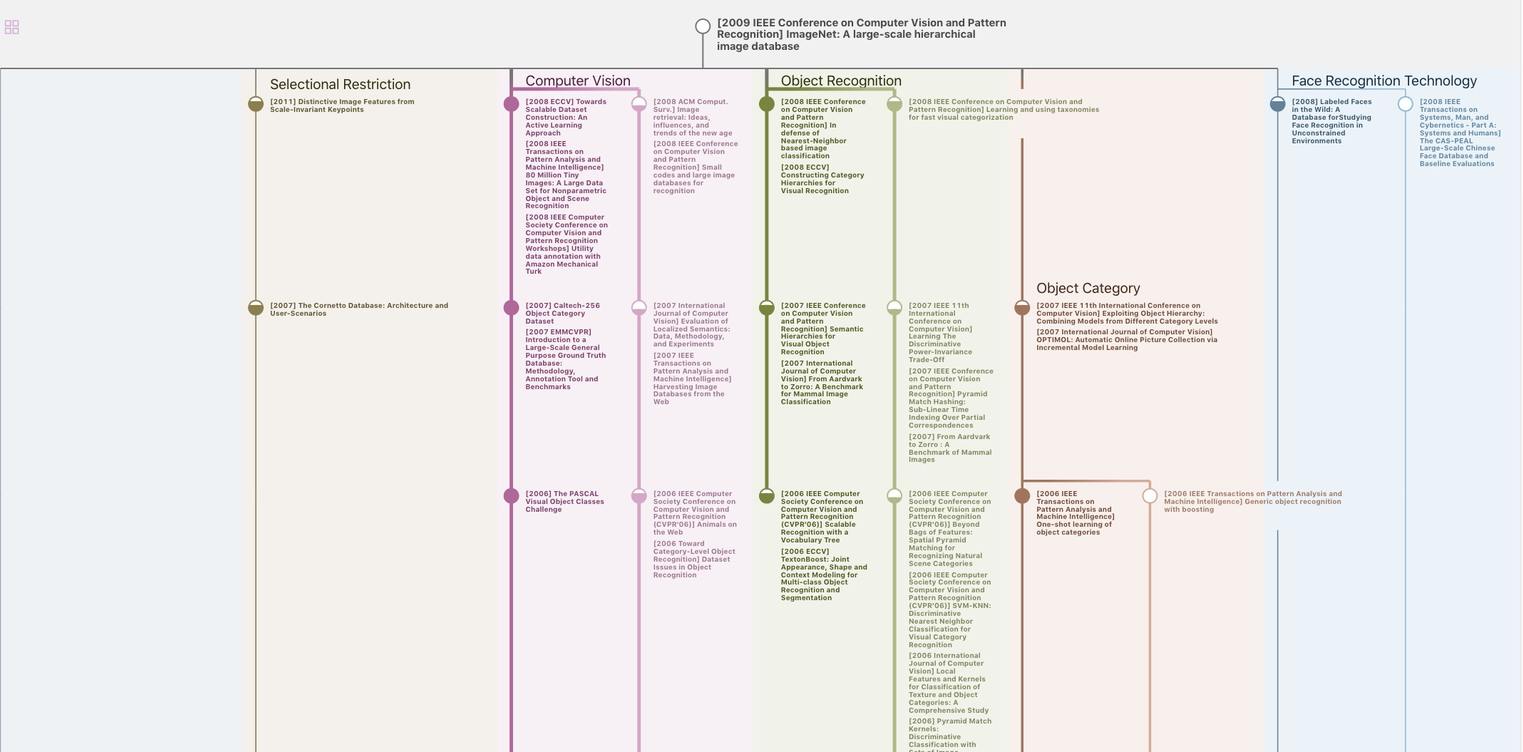
生成溯源树,研究论文发展脉络
Chat Paper
正在生成论文摘要