Ada: Adversarial learning based data augmentation for malicious users detection
APPLIED SOFT COMPUTING(2022)
摘要
Malicious user detection in the recommender systems has attracted much attention in the last two decades because malicious users can seriously affect the recommendation results and user experience. The up-to-date detection models usually concentrate on distinguishing users according to their latent features represented in user embeddings. These models can improve detection performance; however, they are usually not fully up to expectations, especially in the scenarios with unbalanced use samples. From these models that concentrate on user embedding representations, we can summarize the following difficulties: (1) the cost of manual labeling malicious causes the lack of labeled malicious users in training data, which leads to imprecise representations of users; (2) current augmentation methods that aim at mitigating the lack of labeled malicious users are hard to simulate the distribution of malicious users. In this paper, we propose a detection model, using adversarial learning based data augmentation (a.k.a. Ada) to alleviate these problems. Concretely, to get precise representations of users, the model integrates potential user relations and structural similarities into user embeddings. After obtaining precise user representation, it presents a novel data augmentation based on the deep convolutional generative adversarial networks (DCGAN) to simulate the distribution of malicious user embeddings and generate additional fake user embeddings. Experiments on public datasets show our model outperforms state-of-the-art detection models with sparse labeled malicious users, and the ablation study confirms the importance and effectiveness of each component of the model.(C) 2022 Elsevier B.V. All rights reserved.
更多查看译文
关键词
Malicious users detection, Adversarial learning, Data augmentation
AI 理解论文
溯源树
样例
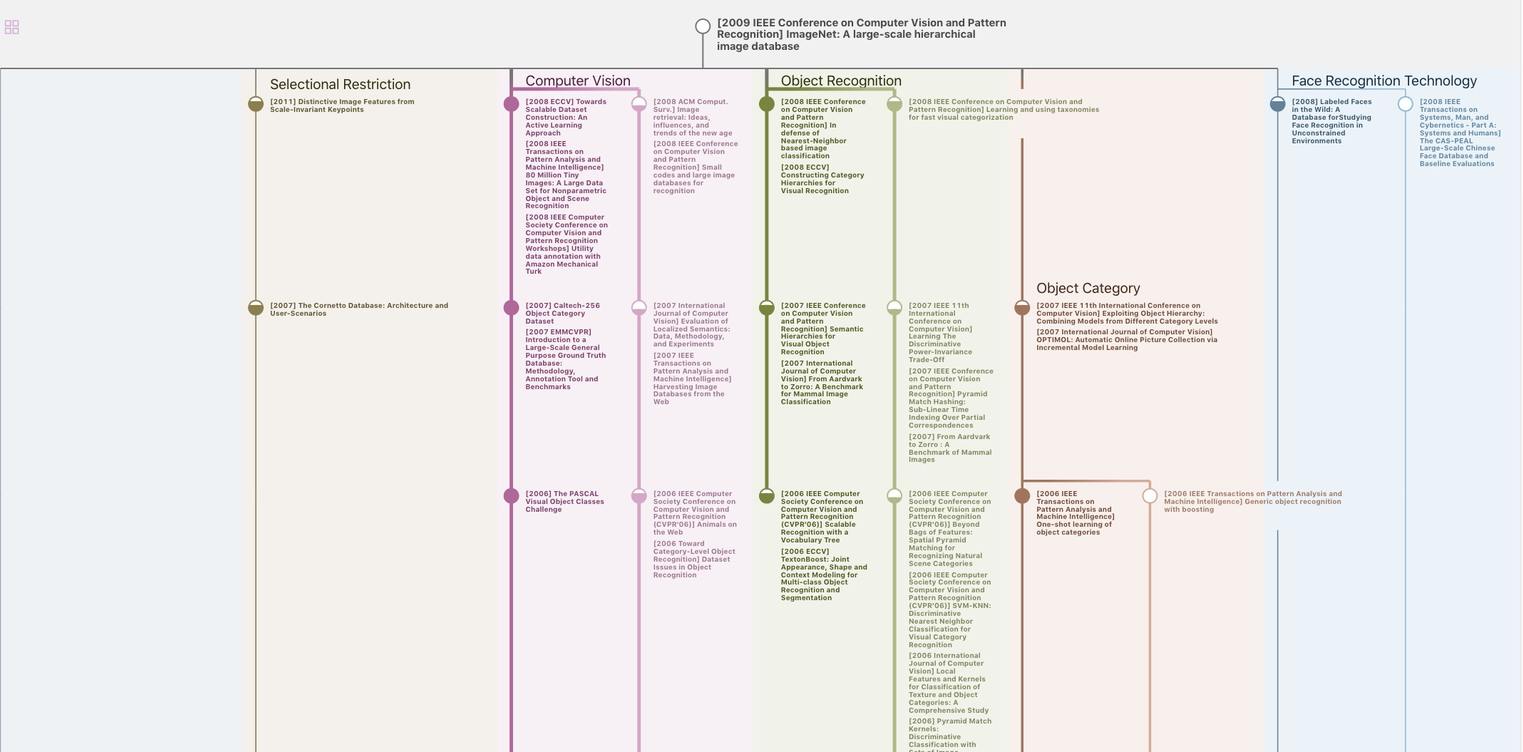
生成溯源树,研究论文发展脉络
Chat Paper
正在生成论文摘要