An electrocorticographic decoder for arm movement for brain-machine interface using an echo state network and Gaussian readout
APPLIED SOFT COMPUTING(2022)
摘要
Brain-machine interface (BMI) studies typically use an electrocorticogram (ECoG) to record neural signals from the surface of the cortex because of the high spatial and temporal resolution and high signal-to-noise levels of the data obtained. However, in certain medical conditions, it may not be possible to place the ECoG electrodes at the target brain regions for BMI. Consequently, developing an ECoG decoder with suitable feature extraction and selection processes is challenging. This study investigated the possibility of a novel ECoG decoder for arm movement BMI. The ECoG signals were recorded from four individuals with intractable epilepsy while imaging and performing a reach-andgrasp movement. We examined the performance of the ECoG decoder using an echo state network and 24 Gaussian readouts within the classification problem paradigm of the arm movement directions. A genetic algorithm was used to optimize the hyperparameters of the ECoG decoder. The ECoG decoder successfully classified 24 arm movement directions in the x-y, x-z, and y-z planes in execution and imagination tasks. The best hit rates were 90.9 +/- 5.3 %, 92.6 +/- 3.9, and 92.6 +/- 4.2 for the x-y, x-z, and y-z planes, respectively. A robot arm control simulation indicated that a real-time movement BMI system could use the novel ECoG decoder. Thus, the echo state network with Gaussian readouts for classification can be a successful ECoG decoder model for motor BMIs. (C) 2021 Published by Elsevier B.V.
更多查看译文
关键词
Brain-machine interface, Electrocorticography, Machine learning, Echo state network, Gaussian readouts, Artificial neural networks, Optimization
AI 理解论文
溯源树
样例
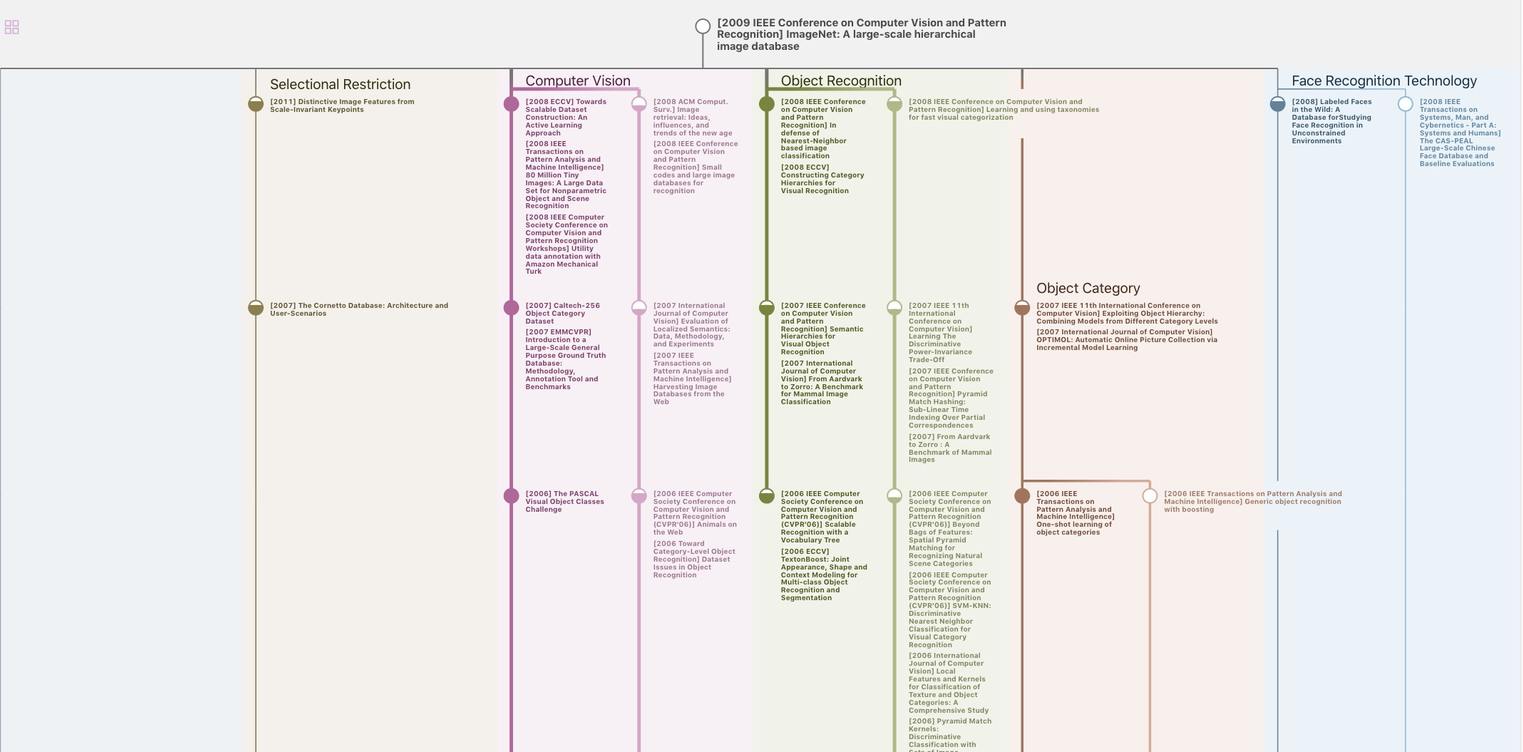
生成溯源树,研究论文发展脉络
Chat Paper
正在生成论文摘要