A Dual Weighting Label Assignment Scheme for Object Detection
IEEE Conference on Computer Vision and Pattern Recognition(2022)
摘要
Label assignment (LA), which aims to assign each training sample a positive (pos) and a negative (neg) loss weight, plays an important role in object detection. Existing LA methods mostly focus on the design of pos weighting function, while the neg weight is directly derived from the pos weight. Such a mechanism limits the learning capacity of detectors. In this paper, we explore a new weighting paradigm, termed dual weighting (DW), to specify pos and neg weights separately. We first identify the key influential factors of pos/neg weights by analyzing the evaluation metrics in object detection, and then design the pos and neg weighting functions based on them. Specifically, the pos weight of a sample is determined by the consistency degree between its classification and localization scores, while the neg weight is decomposed into two terms: the probability that it is a neg sample and its importance conditioned on being a neg sample. Such a weighting strategy offers greater flexibility to distinguish between important and less important samples, resulting in a more effective object detector. Equipped with the proposed DW method, a single FCOS-ResNet-50 detector can reach 41.5% mAP on COCO under 1× schedule, outperforming other existing LA methods. It consistently improves the baselines on COCO by a large margin under various backbones without bells and whistles. Code is available at https://github.com/strongwolf/DW.
更多查看译文
关键词
Recognition: detection,categorization,retrieval
AI 理解论文
溯源树
样例
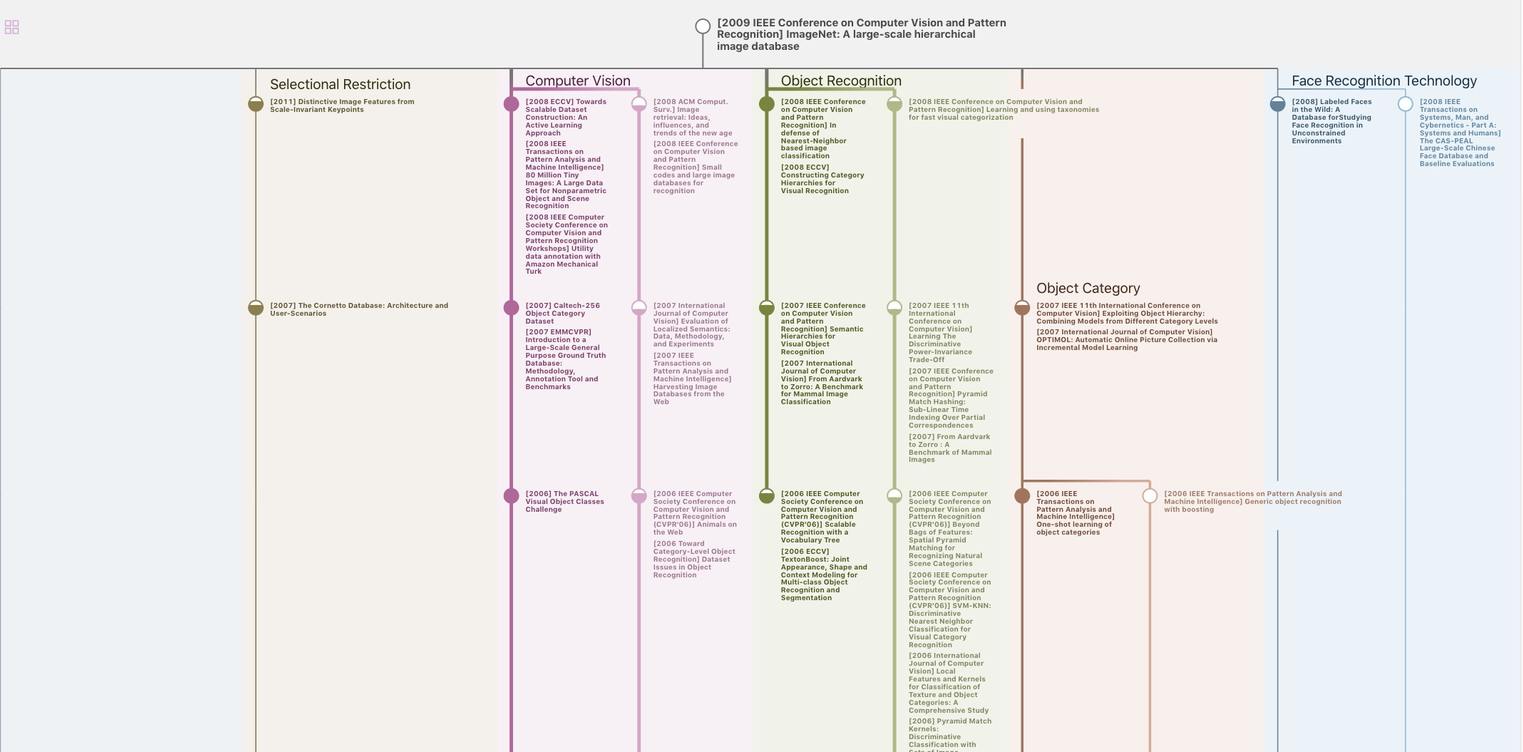
生成溯源树,研究论文发展脉络
Chat Paper
正在生成论文摘要