Magnification Prior: A Self-Supervised Method for Learning Representations on Breast Cancer Histopathological Images
WACV(2023)
摘要
This work presents a novel self-supervised pre-training method to learn efficient representations without labels on histopathology medical images utilizing magnification factors. Other state-of-the-art works mainly focus on fully supervised learning approaches that rely heavily on human annotations. However, the scarcity of labeled and unlabeled data is a long-standing challenge in histopathology. Currently, representation learning without labels remains unexplored in the histopathology domain. The proposed method, Magnification Prior Contrastive Similarity (MPCS), enables self-supervised learning of representations without labels on small-scale breast cancer dataset BreakHis by exploiting magnification factor, inductive transfer, and reducing human prior. The proposed method matches fully supervised learning state-of-the-art performance in malignancy classification when only 20% of labels are used in finetuning and outperform previous works in fully supervised learning settings for three public breast cancer datasets, including BreakHis. Further, It provides initial support for a hypothesis that reducing human-prior leads to efficient representation learning in self-supervision, which will need further investigation. The implementation of this work is available online on GitHub(1).
更多查看译文
关键词
self-supervised learning,contrastive learning,representation learning,breast cancer,histopathological images,transfer learning,medical images
AI 理解论文
溯源树
样例
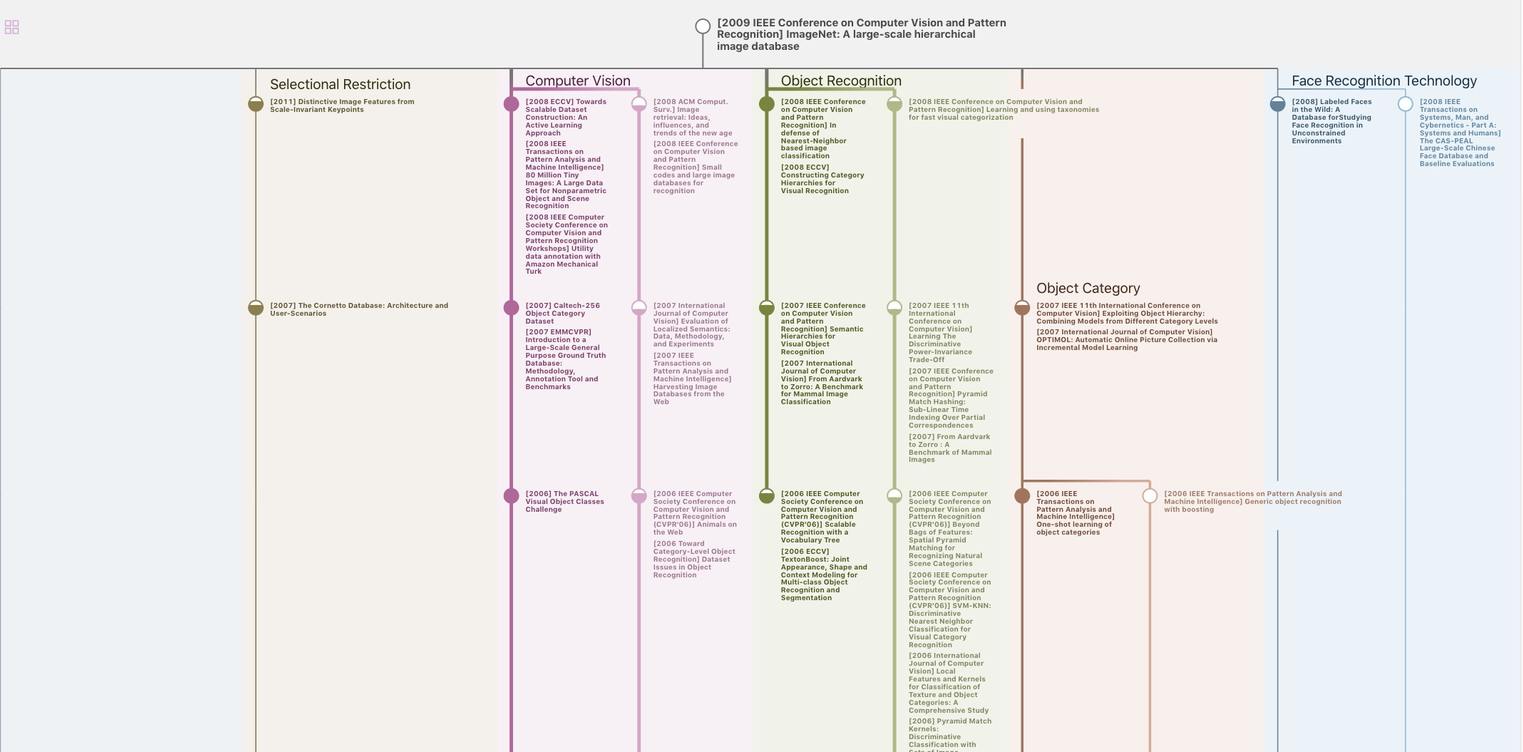
生成溯源树,研究论文发展脉络
Chat Paper
正在生成论文摘要