Diversity of Learning to Control Complex Rehabilitation Robots Using High-Dimensional Interfaces
biorxiv(2022)
摘要
Learning to perform everyday tasks, using a complex robot, presents a nested problem. It is nested, because, on the surface, there is a problem of robot control—but within it, there lies a deeper, more challenging problem that demands the control nuances necessary to perform complicated functional tasks. For individuals with limited mobility, such as those with cervical spinal cord injuries, the addition of physical burden is added to this motor learning burden. An explicit training regime can be designed to accelerate and aid the learning process, with the long-term aim to help individuals (injured or uninjured) acquire the skill of complex robot control. However, such training regimes are not well-established nor are the methods of evaluation. In this paper, we gain a baseline understanding of how humans learn to control a 7 degree-of-freedom assistive robotic arm, using a novel high-dimensional interface, in the absence of explicit training. We examine how participants transition between distinct workspace zones to extract their learning possibilities. We gain additional granularity in individual learning, based on how participants spend their time in the workspace, with the robot, and how the time spent is distributed across trials. These analyses highlight the high diversity of learning. Lastly, we provide benefits and opportunities for targeted training regimes that are explicit and heavily favor individualized support.
### Competing Interest Statement
The authors have declared no competing interest.
更多查看译文
AI 理解论文
溯源树
样例
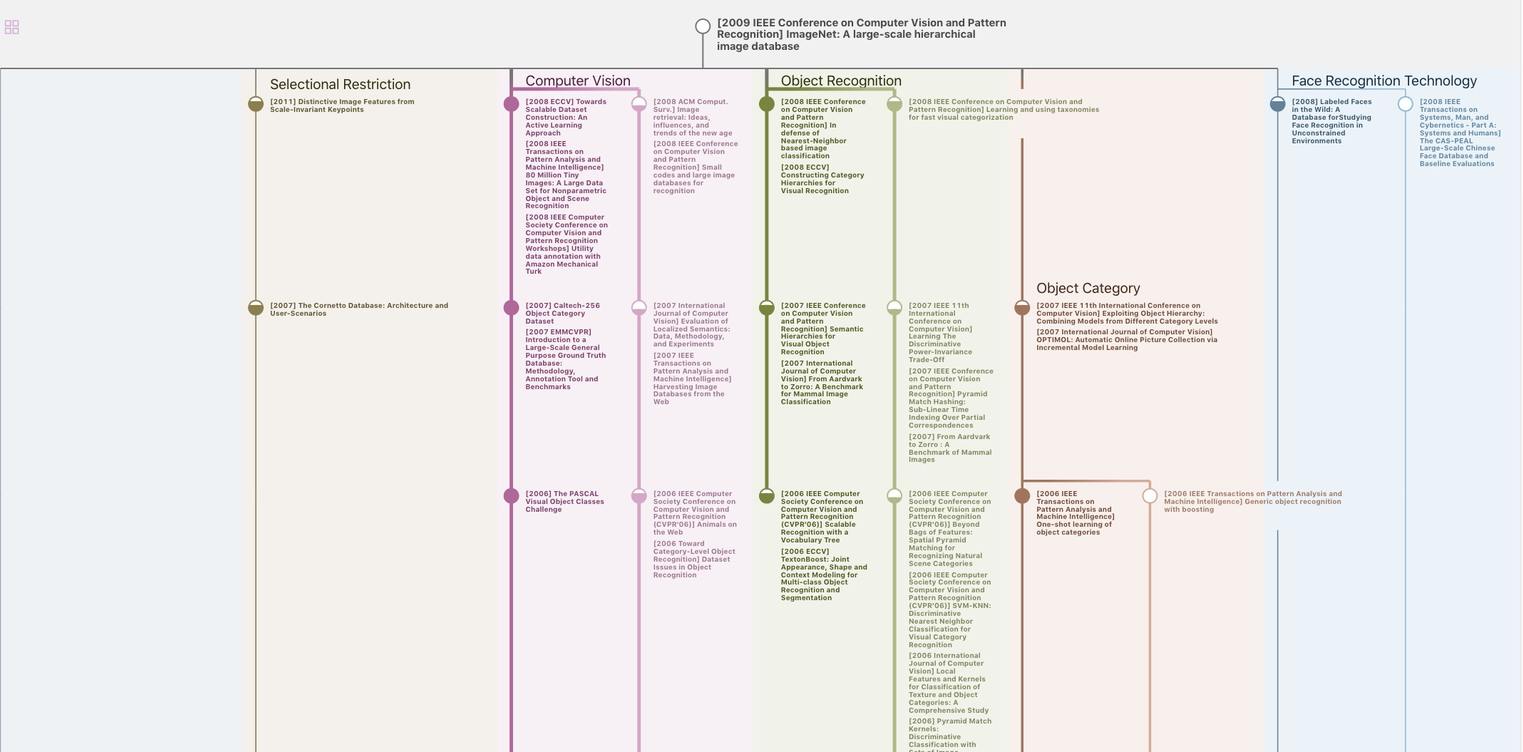
生成溯源树,研究论文发展脉络
Chat Paper
正在生成论文摘要