Abstract PD11-01: An artificial intelligence-based predictor of CDH1 biallelic mutations and invasive lobular carcinoma
Cancer Research(2022)
Abstract
Abstract Introduction: Invasive lobular carcinoma (ILC) is the most frequent special histologic subtype of breast cancer (BC). ILC is identifiable by pathologic assessment given its distinctive discohesive growth pattern, largely caused by CDH1 inactivation. Compared to common forms of BC, ILCs display lower responses to chemotherapy and selective estrogen receptor modulators. The low interobserver agreement for the diagnosis of ILC, however, renders the inclusion of histologic subtyping in therapeutic decision-making challenging. Artificial intelligence (AI)-based algorithms hold promise for improving pathologic diagnosis; their performance, however, depends on the ground truth labeling used. Here, we seek to develop an AI-based methodology for detection of ILC using ‘CDH1 biallelic mutations’ (i.e., mutation + loss-of-heterozygosity of the wild-type allele or two pathogenic somatic mutations) as ground truth, reasoning that in BC, >95% of CDH1 bi-allelic inactivation is found in ILCs.Materials and methods: We developed a convolutional neural network system to detect CDH1 biallelic genetic inactivation (AI-CDH1) using whole slide images (WSI) of 1,100 primary BCs with available targeted sequencing data. The model was trained using a 10-fold cross-validation method to detect biallelic mutations. The mean number of positive and negative samples in the training set was 85.2 (SD=2.57) and 562.8 (SD=10.51) per fold, respectively. The evaluation set consisted of a mean of 14.2 (SD=2.04) positive and 93.8 (SD=9.13) negative samples. We evaluated the performance of the AI-CDH1 classifier to predict the lobular phenotype and CDH1 status using original and revised labels, following a histopathologic re-review of the histologic type and CDH1 status curation. The latter was conducted by incorporating information on biallelic CDH1 inactivation beyond CDH1 mutations (homozygous deletions, deleterious structural rearrangements, and loss-of-heterozygosity and gene promoter methylation).Results: The AI-CDH1 classifier predicted biallelic CDH1 mutations with an area under the curve (AUC)=0.944 (95 CI: 0.925-0.963), sensitivity=91.6% and specificity=85.9%, PPV=49.8%, NPV=98.5% and accuracy=86.7%, and the original ‘lobular phenotype’ with an AUC=0.941 (95 CI: 0.922-0.960), sensitivity=89%, specificity=86.7%, PPV=55.6%, NPV=97.7% and accuracy=87.1%. Review of the CDH1 gene status revealed that 7/957 BCs lacking CDH1 biallelic mutations harbored biallelic CDH1 inactivation by promoter methylation, homozygous deletions or structural rearrangements. The AI-CDH1 classifier detected all seven reclassified BCs and predicted the revised CDH1 biallelic inactivation with an AUC=0.948 (95 CI: 0.930-0.966), sensitivity=92%, specificity=86.5%, PPV=52.3%, NPV=98.5% and accuracy=87.2%. Upon histologic re-review, which resulted in reclassification of 36/927 non-lobular BCs as ‘lobular’ and 5/173 ‘lobular’ BCs as ‘non-lobular’, the AI-CDH1 classifier detected the ‘lobular phenotype’ with an AUC=0.953 (95 CI: 0.935-0.971), sensitivity=90.7%, specificity=89.7%, PPV=66.8%, NPV=97.7% and accuracy=89.9%. Using the revised histologic re-classification and CDH1 biallelic inactivation status labels, the AI-CDH1 classifier predicted the lobular phenotype irrespective of CDH1 status (P>0.05).Conclusions: By training a machine learning system to detect ‘CDH1 biallelic mutations’, as ground truth rather than histologic diagnosis of lobular carcinoma, which might be confounded by human subjectivity, we developed an AI-based system that can detect ILCs accurately, providing a new paradigm for the development of AI-based cancer classification systems. Citation Format: Jorge S Reis-Filho, Fresia Pareja, Fatemeh Derakhshan, David N Brown, Jillian Sue, Pier Selenica, Yi Kan Wang, Arnaud Da Cruz Paula, Monami Banerjee, Zahra Ebrahimzadeh, Manuel Isava, Matthew Lee, Ran Godrich, Adam Casson, Ruben Padron, George Shaikovski, Alexander van Eck, Antonio Marra, Higinio Dopeso, Hannah Y Wen, Edi Brogi, Matthew G Hanna, Chris Kanan, Jeremy D Kunz, Felipe C Geyer, Carla Leibowitz, David Klimstra, Leo Grady, Thomas J Fuchs. An artificial intelligence-based predictor of CDH1 biallelic mutations and invasive lobular carcinoma [abstract]. In: Proceedings of the 2021 San Antonio Breast Cancer Symposium; 2021 Dec 7-10; San Antonio, TX. Philadelphia (PA): AACR; Cancer Res 2022;82(4 Suppl):Abstract nr PD11-01.
MoreTranslated text
Key words
cdh1,biallelic mutations,intelligence-based
AI Read Science
Must-Reading Tree
Example
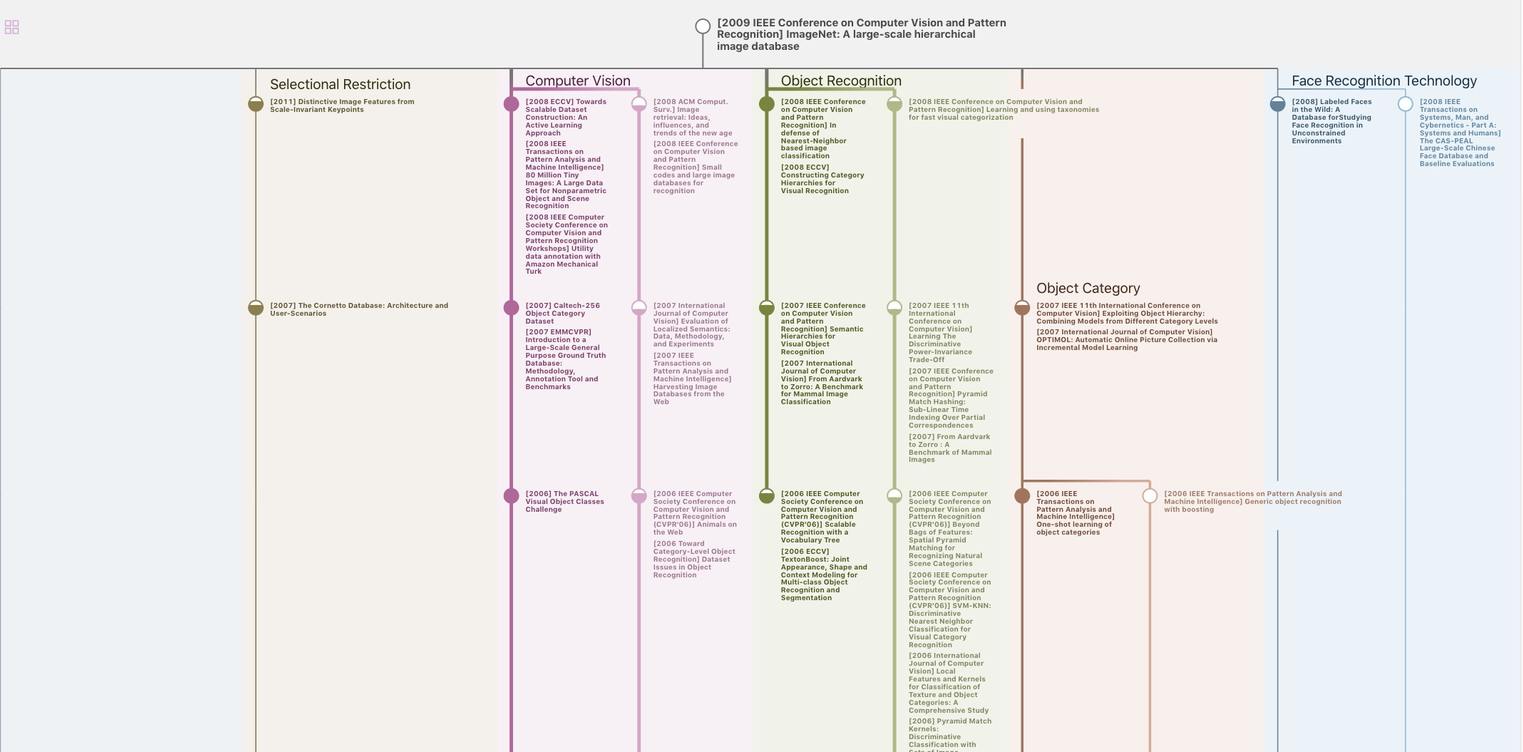
Generate MRT to find the research sequence of this paper
Chat Paper
Summary is being generated by the instructions you defined