Reducing high-frequency time series data in driving studies
Decision Making Based on Data Proceedings IASE 2019 Satellite Conference(2019)
摘要
Driving behavior studies often capture electronic measures at 1-30 Hz for long intervals. It is important to find stochastic models that describe such data, with parameters that can be interpreted and accurately estimated. In this report, we review a family of models that are useful in describing the lateral position of a vehicle in a simulator. These models consist of “projection” and “signed error” pieces, with the latter containing a parameter representing the tendency for drivers to return the vehicles to a central position. We use ad hoc and likelihood-based methods to fit these models, but these all result in biased estimates. Fortunately, in two-group studies, simulations suggest that such biases may offset each other and hence that two-group comparisons may have acceptable accuracy. If we can resolve the bias issue, electronic data from a vehicle might be useful in predicting future errors and crashes.
更多查看译文
AI 理解论文
溯源树
样例
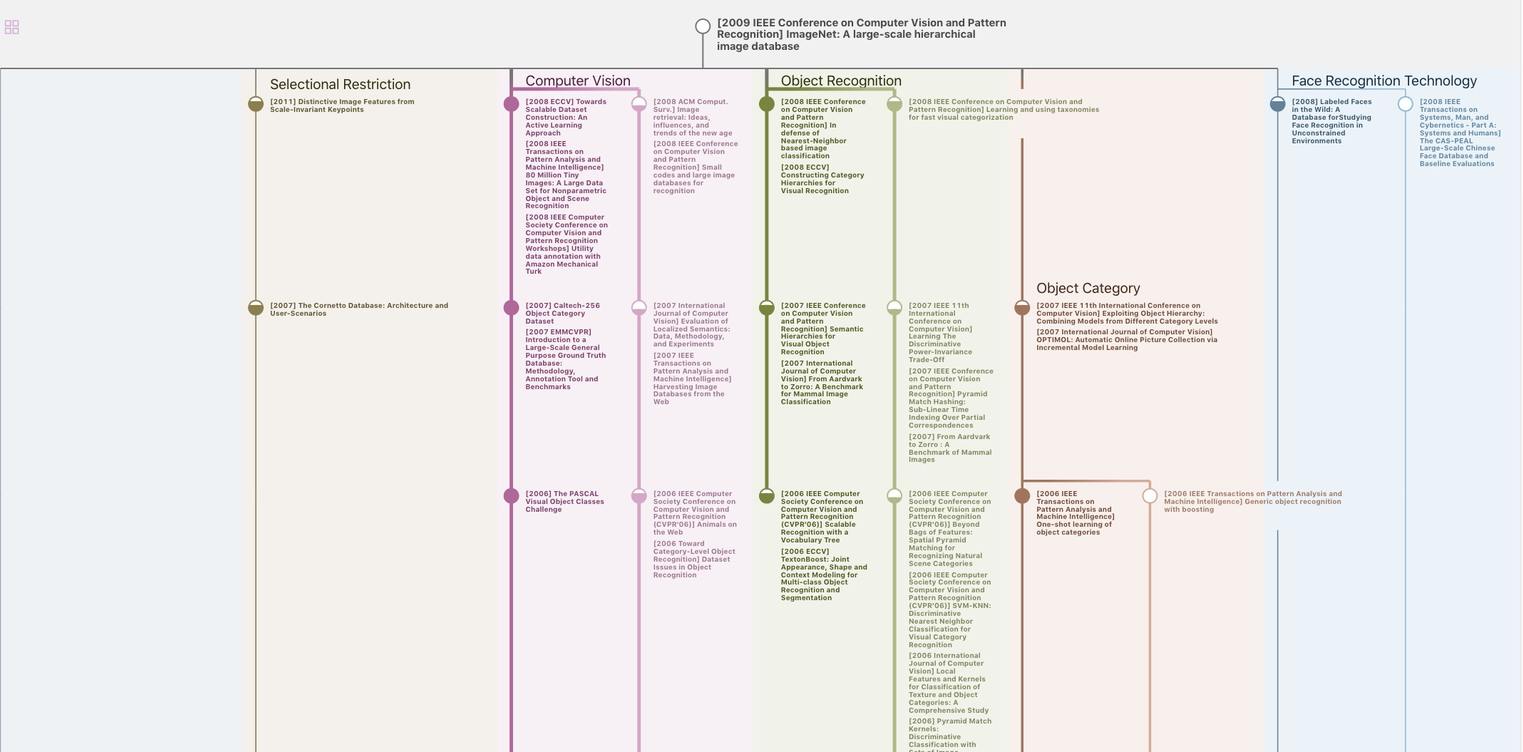
生成溯源树,研究论文发展脉络
Chat Paper
正在生成论文摘要