Ultrasonic Characterization of Biomimetic Porous Scaffold Using Machine Learning: Application of Biot’s Theory
Volume 1: Acoustics, Vibration, and Phononics(2021)
摘要
Abstract A two-dimensional infinite length porous slab is employed to simulate biomimetic porous scaffold. The pores of slab are saturated with a relatively low and high viscous fluids such as air and bone marrow. Ultrasonic waves based on the Biot-JKD formulation travel through the porous slab and create viscous exchanges between the skeletal frame and the fluid. The Biot-JKD formulation focuses on the parameters, biomarkers of the biomimetic porous scaffold, which are sensitive to the transmission and reflection signals. These parameters include porosity, tortuosity, viscous characteristic length, Young’s modulus, and Poisson’s ratio. An artificial neural network (ANN) based on a set of the biomarkers is rendered to model the transmitted and reflected waves from the porous slab. The validation of the proposed analytical approach and released artificial neural network is evaluated by the pertinent literature. The output of the artificial neural network, the transmitted-reflected waves, is inversely applied to the analytical expression to estimate the biomarkers associated with bone regeneration. The results show that for a medium filled with a relatively high viscous fluid the longitudinal waves are more prone to estimate mechanical properties of the medium such as Young’s modulus and Poisson’s ratio while the transverse waves, in addition to longitudinal waves, are essential to estimate the physical properties of the medium including porosity, tortuosity, and viscous characteristic length. Furthermore, it is also concluded that for the medium filled with a relatively low viscous fluid such as air the longitudinal waves alone is able to estimate the biomarkers, which reduce significantly the computational efforts.
更多查看译文
关键词
Acoustic Waves, Biomimetic porous scaffold, Machine Learning, Porous Media, Viscous Fluid, Inverse Problem
AI 理解论文
溯源树
样例
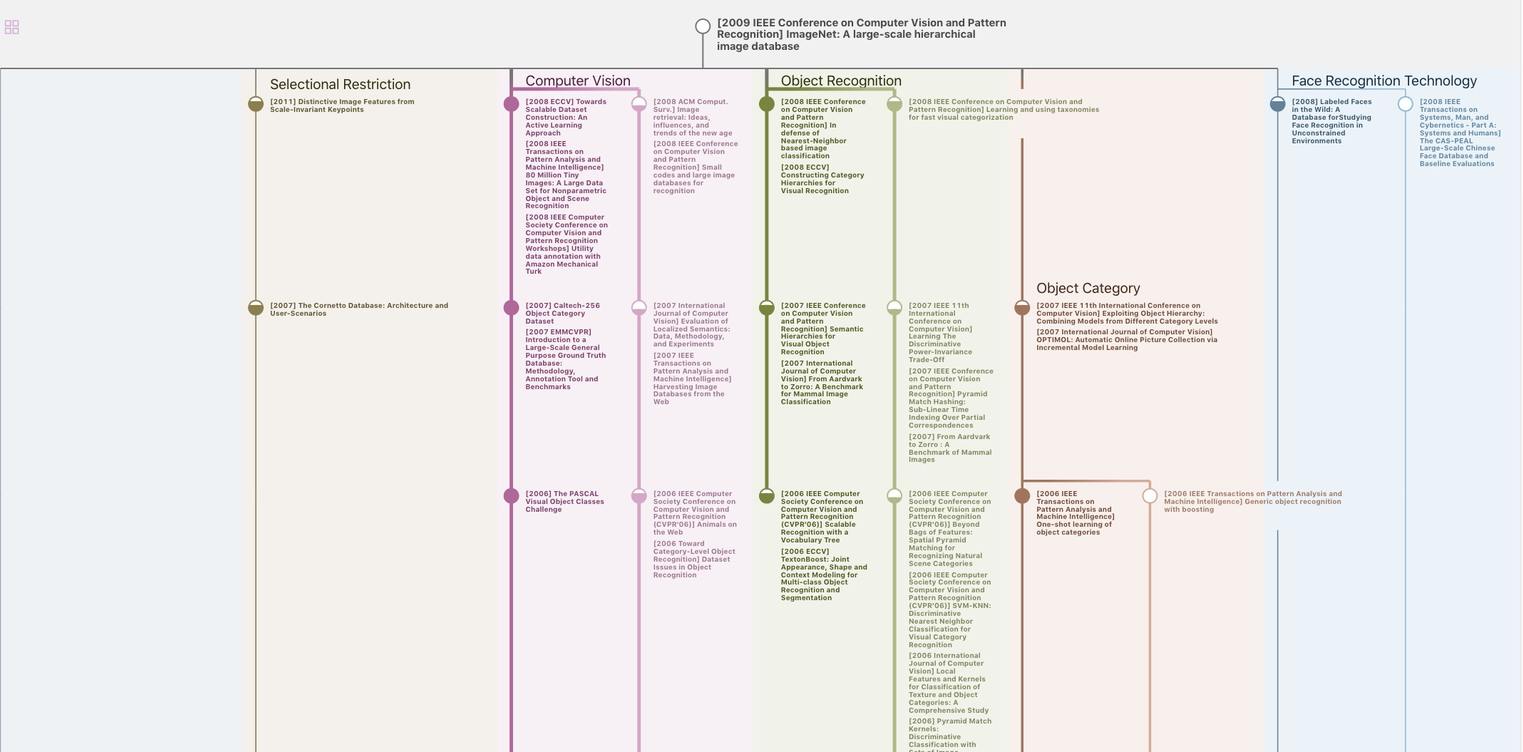
生成溯源树,研究论文发展脉络
Chat Paper
正在生成论文摘要