Ensemble Learning-Based Differential Distinguishers for Lightweight Cipher
Proceedings of the 2021 5th International Conference on Electronic Information Technology and Computer Engineering(2021)
摘要
Combination of machine learning and cryptoanalysis is a novel filed. The application of machine learning decreases the manual work, time cost and storage space. On the basis of CRYPTO 2019 Gohr's work, we proposed an EL (ensemble learning) based differential distinguishers of AdaBoost, Random Forest, Extremely Randomized Trees and Gradient Boosting Decision Tree which prove stable differential distinguishing result. The result shows the Random Forest based differential distinguisher can distinguish the 6-round Speck with cipher 106 pairs in 89.01 seconds. The Gradient Boosting Decision Tree distinguisher can distinguish 6-round Speck with 106 cipher pairs in 175.60 seconds and proves accuracy up to 0.5840. After our analysis, compared with the analysis method based on machine learning, the amount of data needed by the traditional difference analysis method is about 67 times as much as that of the new method. Our finding shows the ensemble learning method performs well in the differential distinguishing task for lightweight Markov cipher.
更多查看译文
AI 理解论文
溯源树
样例
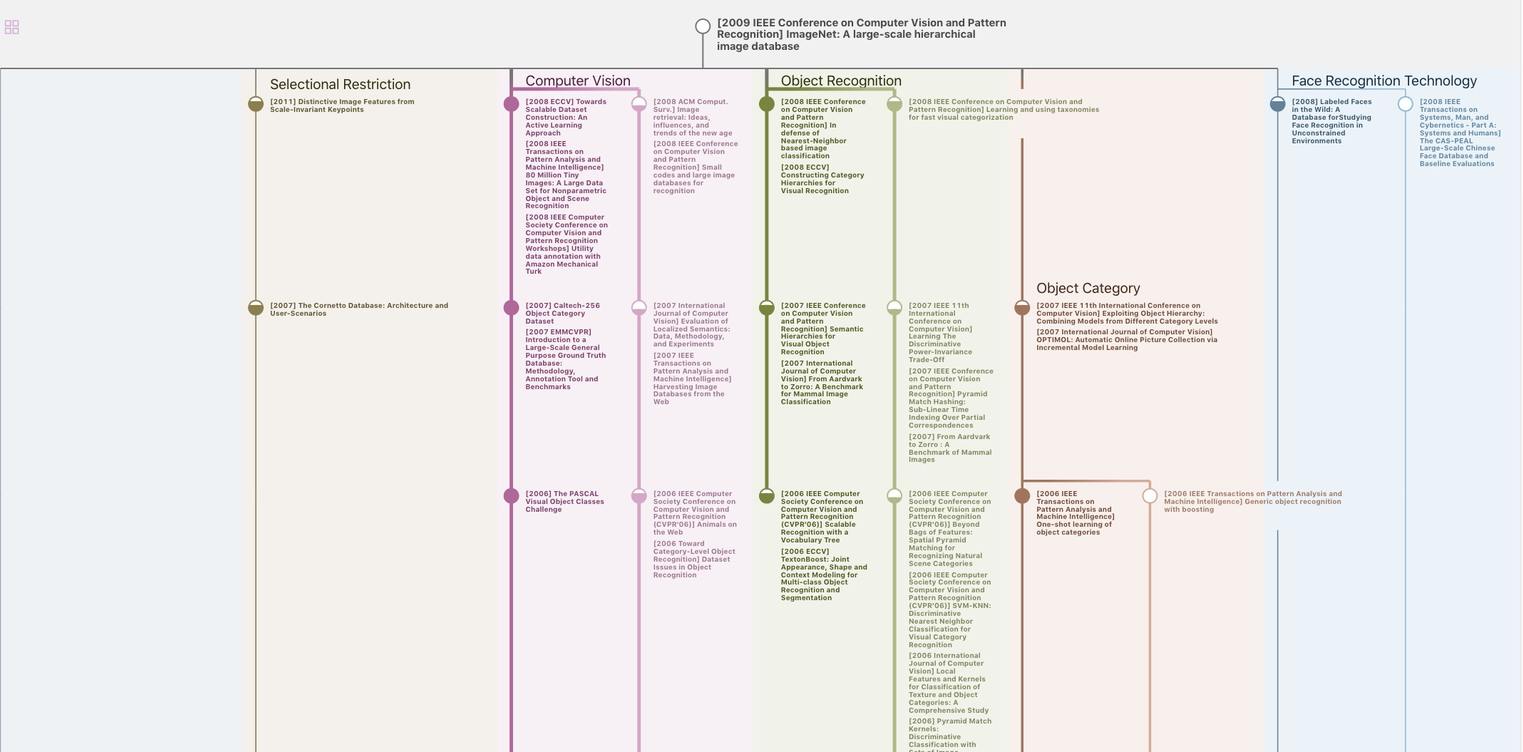
生成溯源树,研究论文发展脉络
Chat Paper
正在生成论文摘要