A clustering-based active learning method to query informative and representative samples
Applied Intelligence(2022)
摘要
Active learning (AL) has widely been used to address the shortage of labeled datasets. Yet, most AL techniques require an initial set of labeled data as the knowledge base to perform active querying. The informativeness of the initial labeled set significantly affects the subsequent active query; hence the performance of active learning. In this paper, a new clustering-based active learning framework, namely Active Learning using a Clustering-based Sampling (ALCS), is proposed to simultaneously consider the representativeness and informativeness of samples using no prior label information. A density-based clustering approach is employed to explore the cluster structure from the data without requiring exhaustive parameter tuning. A simple yet effective distance-based querying strategy is adopted to adjust the sampling weight between the center-based and boundary-based selections for active learning. A novel bi-cluster boundary-based sample query procedure is introduced to select the most uncertain samples across the boundary among adjacent clusters. Additionally, we developed an effective diversity exploration strategy to address the redundancy among queried samples. Our extensive experimentation provided a comparison of the ALCS approach with state-of-the-art methods, exhibiting that ALCS produces statistically better or comparable performance than state-of-the-art methods.
更多查看译文
关键词
Active learning,Clustering,Informative-based query,Representative-based query,Center-based selection,Boundary-based selection
AI 理解论文
溯源树
样例
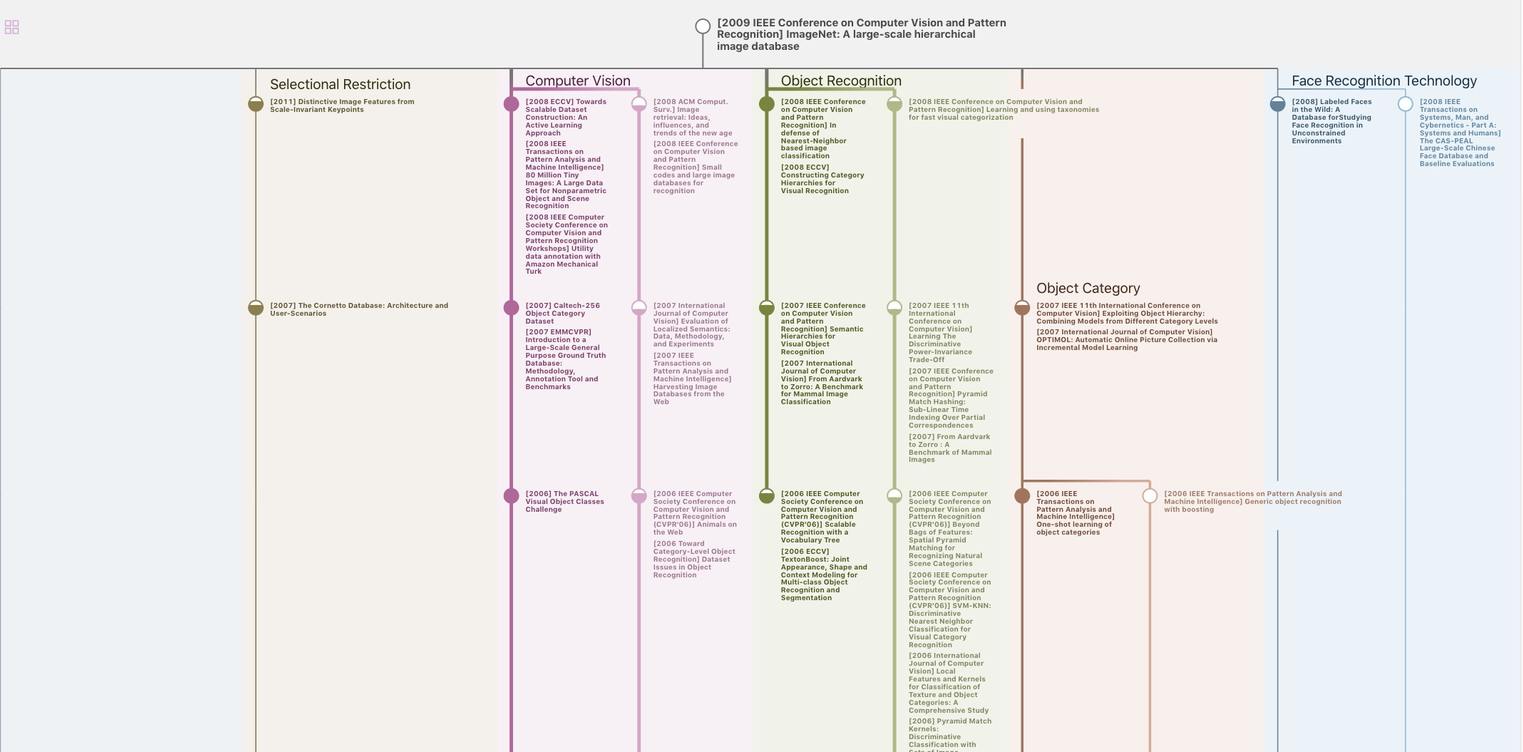
生成溯源树,研究论文发展脉络
Chat Paper
正在生成论文摘要