Bipartite graph capsule network
World Wide Web (WWW)(2022)
摘要
Graphs have been widely adopted in various fields, where many graph models are developed. Most of previous research focuses on unipartite or homogeneous graph analysis. In this graphs, the relationships between the same type of entities are preserved in the graphs. Meanwhile, the bipartite graphs that model the complex relationships among different entities with vertices partitioned into two disjoint sets, are becoming increasing popular and ubiquitous in many real life applications. Though several graph classification methods on unipartite and homogenous graphs have been proposed by using kernel method, graph neural network, etc. However, these methods are unable to effectively capture the hidden information in bipartite graphs. In this paper, we propose the first bipartite graph-based capsule network, namely Bipartite Capsule Graph Neural Network (BCGNN), for the bipartite graph classification task. BCGNN exploits the capsule network and obtains information between the same type vertices in the bipartite graphs by constructing the one-mode projection. Extensive experiments are conducted on real-world datasets to demonstrate the effectiveness of our proposed method.
更多查看译文
关键词
Capsule network,Graph neural network,Bipartite graph,Graph classification
AI 理解论文
溯源树
样例
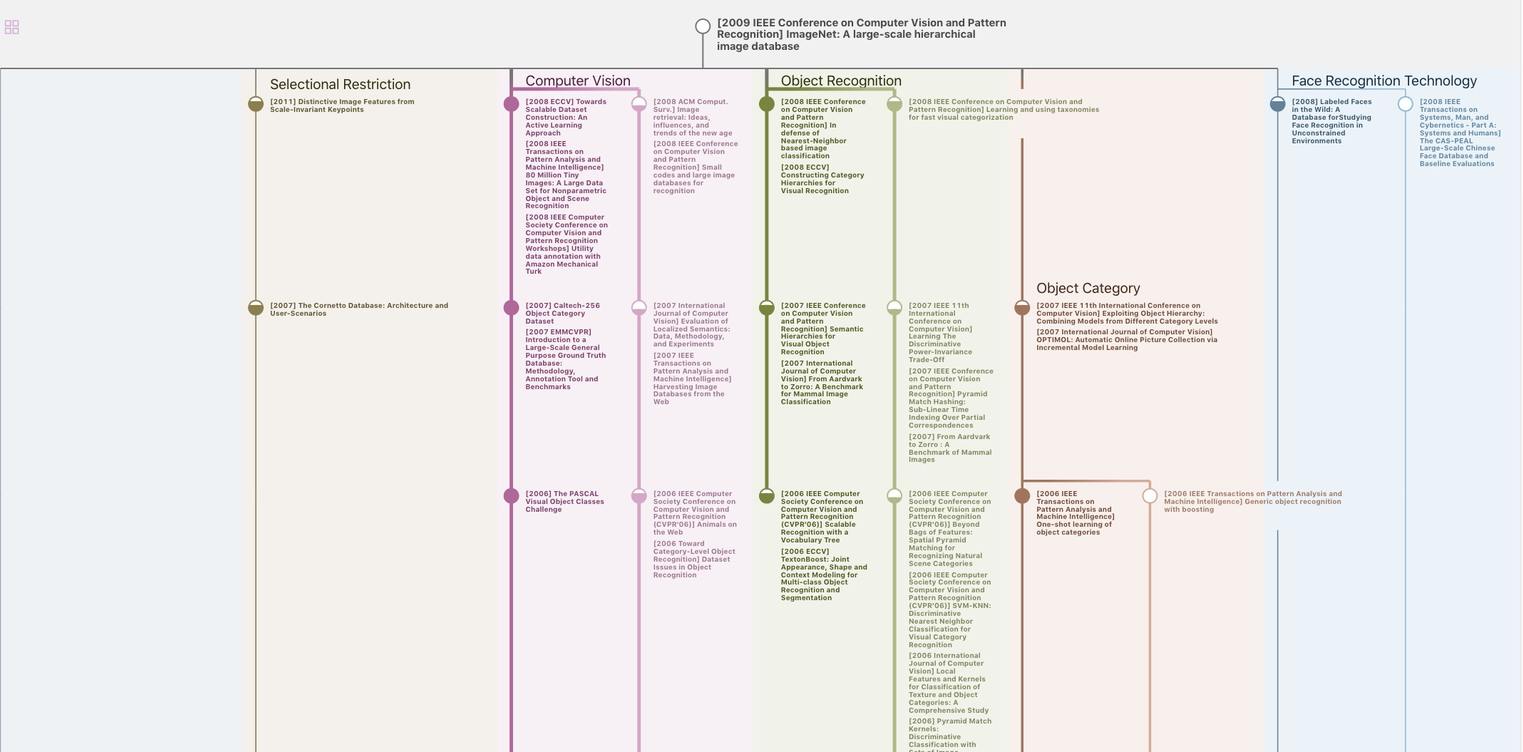
生成溯源树,研究论文发展脉络
Chat Paper
正在生成论文摘要